Classification Performance Of Multilayer Perceptrons With Different Risk Functionals
INTERNATIONAL JOURNAL OF PATTERN RECOGNITION AND ARTIFICIAL INTELLIGENCE(2014)
摘要
In the present paper, we assess the performance of information-theoretic inspired risk functionals in multilayer perceptrons with reference to the two most popular ones, Mean Square Error and Cross-Entropy. The information-theoretic inspired risks, recently proposed: HS and HR2 are, respectively, the Shannon and quadratic Renyi entropies of the error; ZED is a risk reflecting the error density at zero errors; EXP is a generalized exponential risk, able to mimic a wide variety of risk functionals, including the information-thoeretic ones. The experiments were carried out with multilayer perceptrons on 35 public real-world datasets. All experiments were performed according to the same protocol. The statistical tests applied to the experimental results showed that the ubiquitous mean square error was the less interesting risk functional to be used by multilayer perceptrons. Namely, mean square error never achieved a significantly better classification performance than competing risks. Cross-entropy and EXP were the risks found by several tests to be significantly better than their competitors. Counts of significantly better and worse risks have also shown the usefulness of HS and HR2 for some datasets.
更多查看译文
关键词
Risk functionals, classification, multilayer perceptrons
AI 理解论文
溯源树
样例
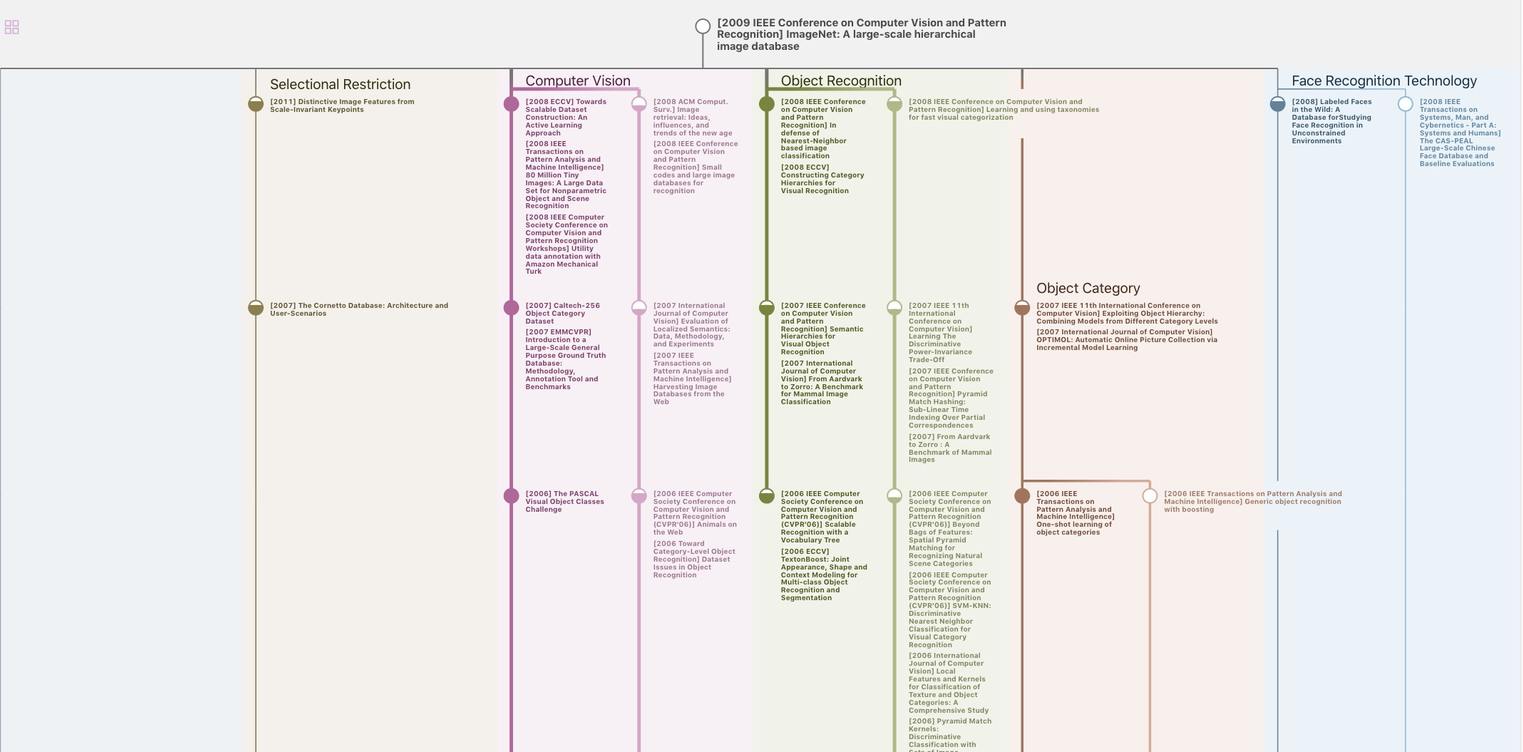
生成溯源树,研究论文发展脉络
Chat Paper
正在生成论文摘要