Mean Polynomial Kernel And Its Application To Vector Sequence Recognition
IEICE TRANSACTIONS ON INFORMATION AND SYSTEMS(2014)
摘要
Classification tasks in computer vision and brain-computer interface research have presented several applications such as biometrics and cognitive training. However, like in any other discipline, determining suitable representation of data has been challenging, and recent approaches have deviated from the familiar form of one vector for each data sample. This paper considers a kernel between vector sets, the mean polynomial kernel, motivated by recent studies where data are approximated by linear subspaces, in particular, methods that were formulated on Gra.ssmann manifolds. This kernel takes a more general approach given that it can also support input data that can be modeled as a vector sequence, and not necessarily requiring it to be a linear subspace. We discuss how the kernel can be associated with the Projection kernel, a Grassmann kernel. Experimental results using face image sequences and physiological signal data show that the mean polynomial kernel surpasses existing subspace-based methods on Grassmann manifolds in terms of predictive performance and efficiency.
更多查看译文
关键词
kernel methods, support vector machines, Grassmann distance and kernels, face recognition, brain-computer interface, vector sequence, binary classification
AI 理解论文
溯源树
样例
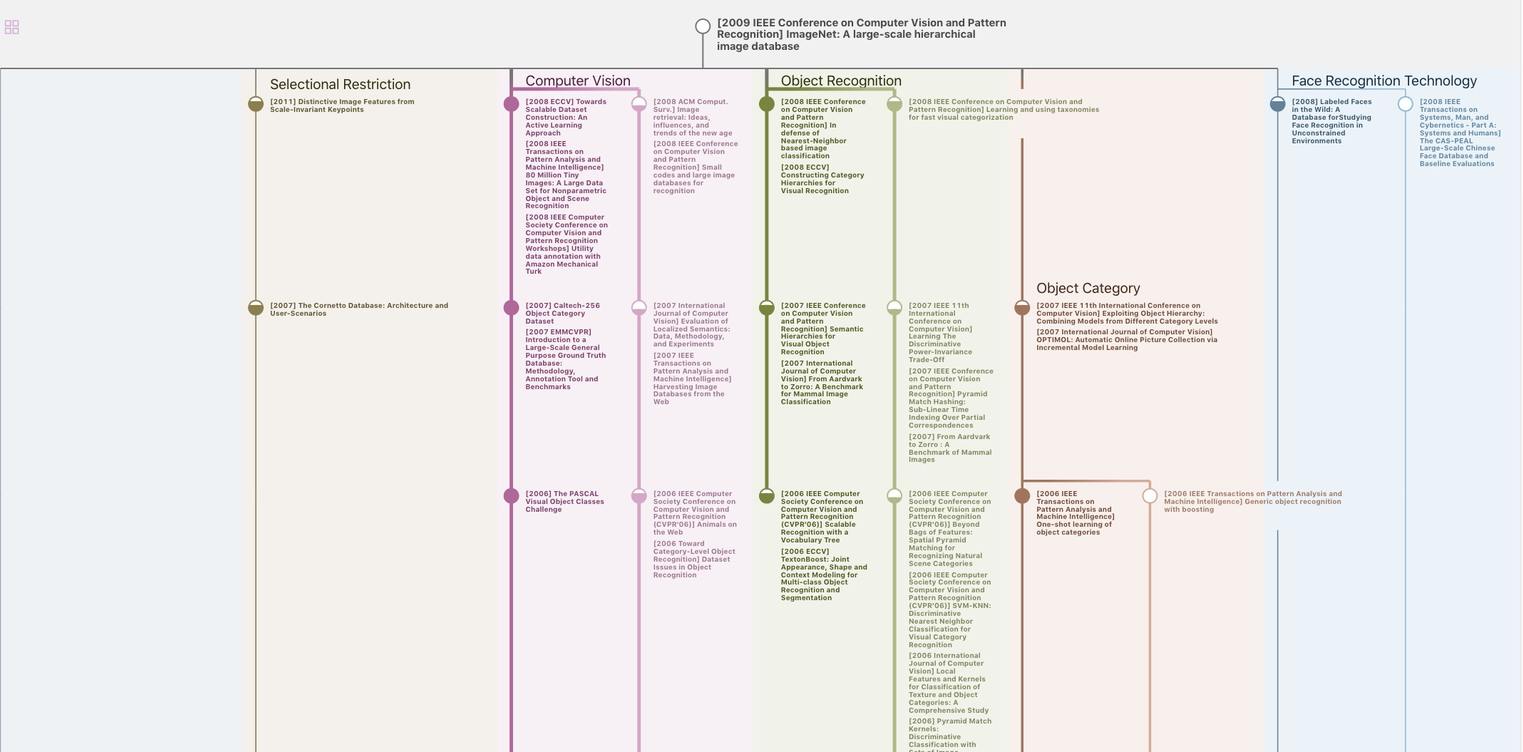
生成溯源树,研究论文发展脉络
Chat Paper
正在生成论文摘要