Scaling range automated selection for wavelet leader multifractal analysis.
Signal Processing(2014)
摘要
Scale invariance and multifractal analysis constitute paradigms nowadays widely used for real-world data characterization. In essence, they amount to assuming power law behaviors of well-chosen multiresolution quantities as functions of the analysis scale. The exponents of these power laws, the scaling exponents, are then measured and involved in classical signal processing tasks. Yet, the practical estimation of such exponents implies the selection of a range of scales where the power law behaviors hold, a difficult task with yet crucial impact on performance. In the present contribution, a nonparametric bootstrap based procedure is devised to achieve scaling range automated selection. It is shown to be effective and relevant in practice. Its performance, benefits and computational costs are assessed by means of Monte Carlo simulations. It is applied to synthetic multifractal processes and shown to yield robust and accurate estimation of multifractal parameters, despite various difficulties such as noise corruption or inter-subject variability. Finally, its potential is illustrated at work for the analysis of adult heart rate variability on a large database.
更多查看译文
关键词
Automated scaling range selection,Bootstrap,Wavelet leaders,Multifractal analysis
AI 理解论文
溯源树
样例
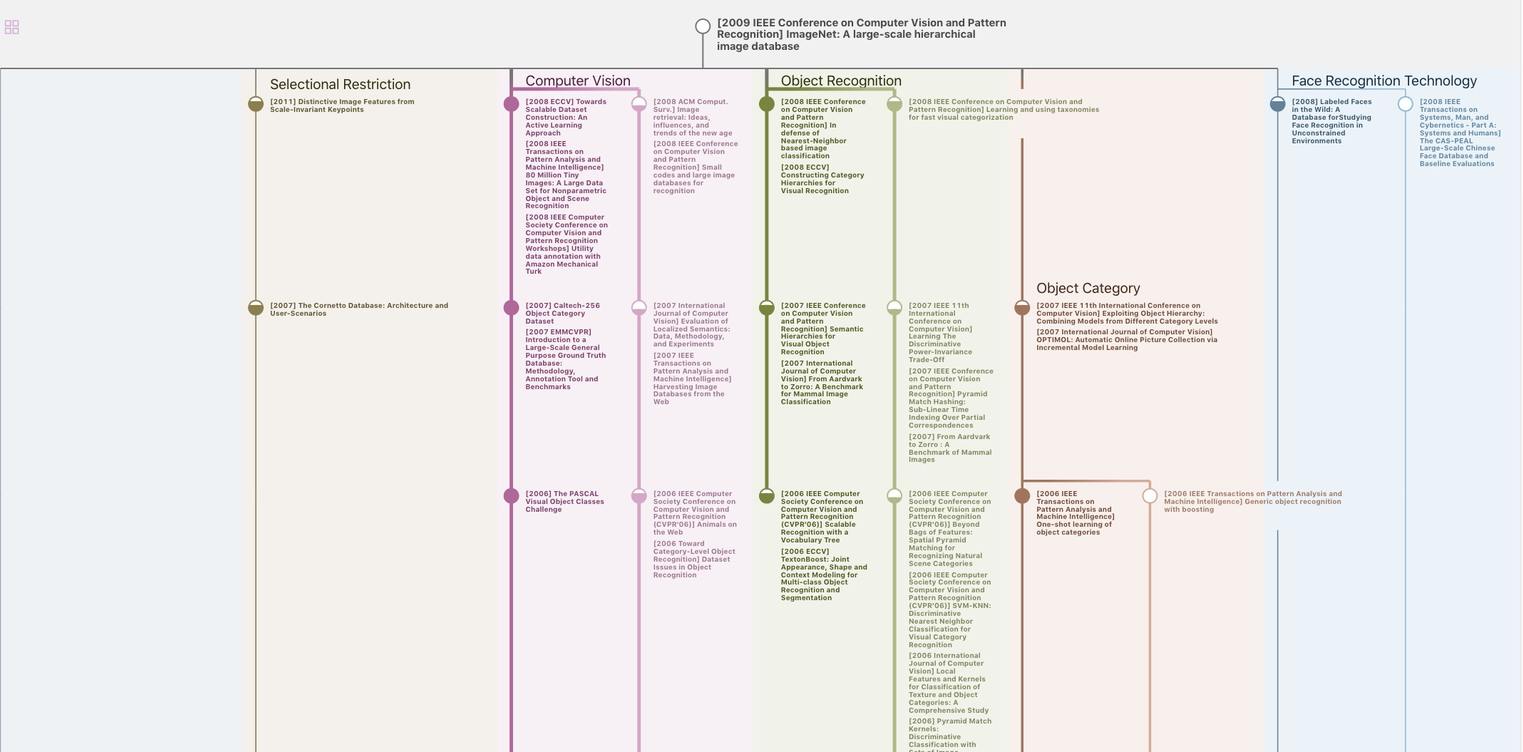
生成溯源树,研究论文发展脉络
Chat Paper
正在生成论文摘要