Quid Pro Quo: Reducing Peak Traffic Costs with (Subscriber) Price Incentives.
ACM SIGMETRICS Performance Evaluation Review(2014)
摘要
Motivation: ISPs today are seeing unprecedented growth in residential broadband traffic volumes driven by the wide- spread popularity of video streaming services such as Net- flix, Hulu, Youtube and so on. This traffic explosion creates a need for (expensive) periodic network capacity upgrades and also increases transit costs paid to upstream providers. As a response, several ISPs started to impose time-of-day data caps, i.e., where per subscriber data usage limits are defined for particular periods of the day, e.g. BRC [USA] from 5pm-1am daily.While these have the effect of reducing traffic in busy periods (and hence costs), they also run the risk of alienating subscribers due their punitive nature. In this abstract, we propose an alternative mechanism where the ISP has a simple fixed monthly subscription price but also applies price discounts to influence subscribers to shift their traffic demands outside of the peak windows. Cost Model: Most ISPs size the network for peak demand and over-provision network capacity so that average utilization stays below a threshold p . Capacity upgrades are triggered by sustained, upward shifts in the peak traffic (denoted P 100 ). Hence, capacity cost can be assumed to scale proportionally to a rate factor c (ε/Mbps) and P 100 . The second cost component, transit, is charged based on the 95th percentile (denoted P 95 ) of the transit traffic (computed over 5 minute windows in a month) and a committed price r (ε per Mbps). The actual fraction of transit traffic ( f ) depends greatly on several factors -- ISP size, geographic market, etc. For example, f ~ 0.3 in Japan, and f ~ 0.8 in Africa [1]. Utility Model: Previous work has considered user utility to be a quadratic function of the monthly data usage [2]. In this abstract, we assume a general model where each subscriber may have different utility functions at different peak-time intervals. Hence, for a subscriber k that generates w t amount of data (in bytes) in t , the total utility will be: U k (w) = ∑/ t α kt • ( b kt • w' t -- w' 2 t /2) (1) where w' t = min v{ w t , b kt } and α kt , b kt are constants. IncentiveMechanism: The exact price discount is based on two values computed by the ISP in each interval t: (i) The implementation of the scientific publication is co-financed through the Project "State Scholarships Foundation" from the resources of the Operational Program "Education & Lifelong Learning", European Social Fund (ESF) and the NSRF, 2007-2013. The first author would like to acknowledge the "Alexander S. Onassis" Public Benefit Foundation, Greece for providing a scholarship. a data threshold D t ≥ 0 (in bytes) and (ii) a discount rate pt ≥ 0 (in ε/byte). If the monthly data usage of k inside t , d kt , is lower than D t , k gets a discount pt • ( D t -- d kt ). Then, k will adopt her behavior so that: Max/ d k ≥0 U k ( d k ) + ∑ P t • ( D t ? d kt (D, p))+ (2) where (.)+ = max(., 0). D, p and d k are the vectors 8t. We assume that the ISP can estimate the per subscriber daily traffic pattern and predict how it will change as a response to the incentives by solving the problem in (2). Then, the ISP will try to minimize the incentive and traffic costs: Min/D,p ∑/ t ( pt ∑( D t -- d kt (D, p))+) + r • f • P95 + c/? • P 100 (3) where P 95 , P 100 are the traffic percentiles after incentives. Approximation: The above problem is NP-Hard in general. The difficulty lies on the ordering in the objective function, which renders it non-continuous. Interestingly, the slightly different problem for which we are to minimize the weighted sum of all the percentile values from the 95th up to the 100th, is of lower complexity. Specifically, this problem can be solved by replacing the percentile terms with new optimization variables and adding a set of linear constraints [3]. The ISP can alternatively solve the above problem to get a solution close to the optimal one for the problem in (3). Evaluation: We applied the price incentives on a dataset of 223 subscribers of a European ISP measured in Dec. 2013. The ISP offers discounts only during the peak period of 6- 11pm, at 1 hour granularity. We set bkt to the total data usage of k in t in our dataset, and considered utility to be equal to the tariff price to find akt. We find evidence of tangible benefits to the ISP (up to 24% cost reduction), while the discounts varied from 0.08e - 1.45e per subscriber. Conclusion: The proposed mechanism does not mandate changes in behavior, but instead offers a quid-pro-quo to subscribers. In contrast to previous incentive mechanisms [4], [5], our scheme is designed requiring no dynamic traffic management and additional communication between the ISP and the subscribers.
更多查看译文
AI 理解论文
溯源树
样例
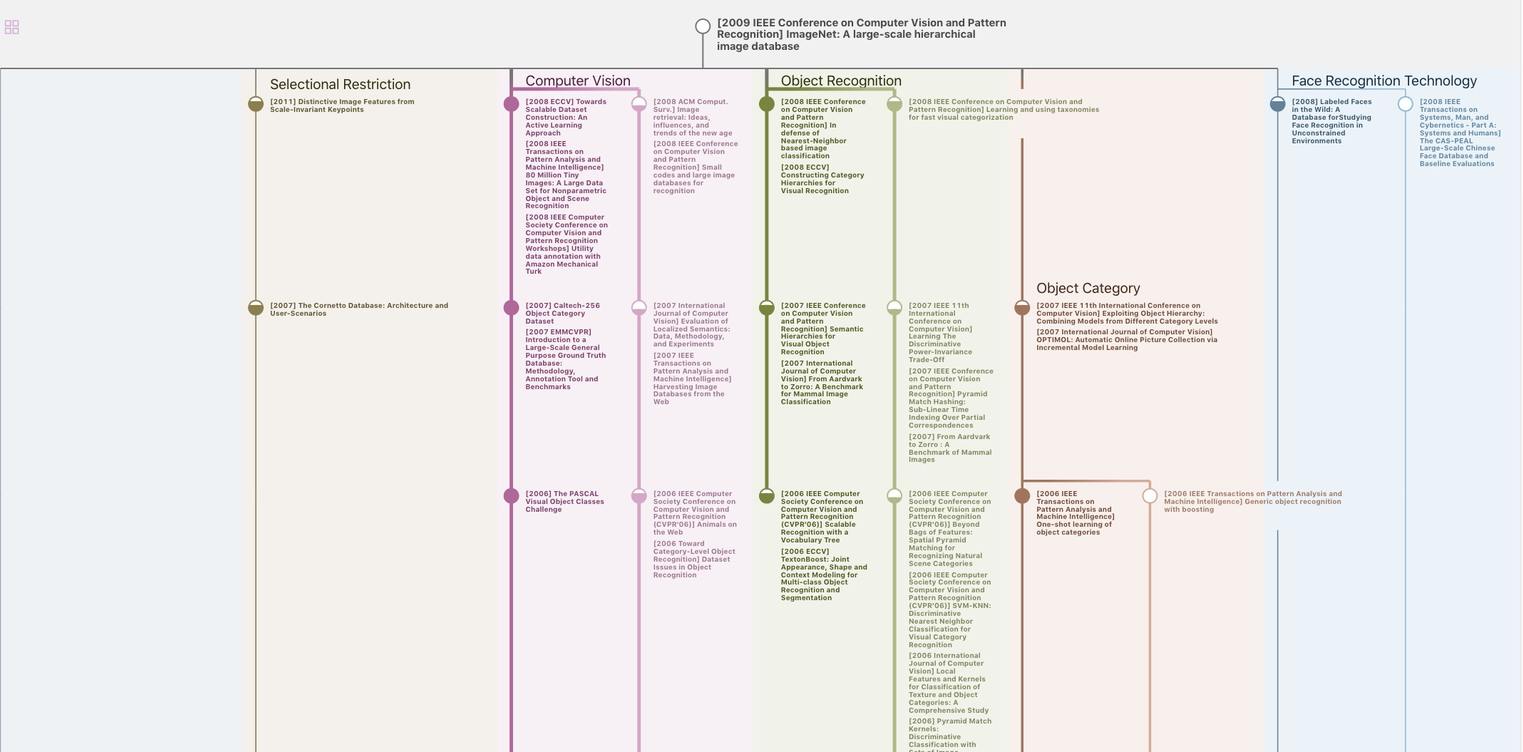
生成溯源树,研究论文发展脉络
Chat Paper
正在生成论文摘要