Cognitive pedestrian detector: Adapting detector to specific scene by transferring attributes.
Neurocomputing(2015)
摘要
Training a reliable generic pedestrian detector on different scenes is still a very challenging problem. In this paper, we propose a novel transfer learning framework for improving the performance of a generic detector by adapting the detector to a scene specific detector. The main contributions come from 2 aspects: (1) instead of hand-crafted ad-hoc rules, a scene based auxiliary attribute classifier and a position priori map are automatically trained from target scene to collect confident samples; (2) conditional distribution transfer sparse coding is presented to match the conditional distributions of the source and the target samples. Experiments show our approach significantly improves the performance of the generic detector and outperforms the state-of-the-art adapting approaches in benchmark datasets. Comparing with the state-of-the-art methods, the improvements are 6% on the CUHK square pedestrian dataset and 33% on the ETH pedestrian dataset which is considered quite hard because the background is dynamic.
更多查看译文
关键词
Detector adaption,Transfer learning,Sparse coding,Pedestrian detection
AI 理解论文
溯源树
样例
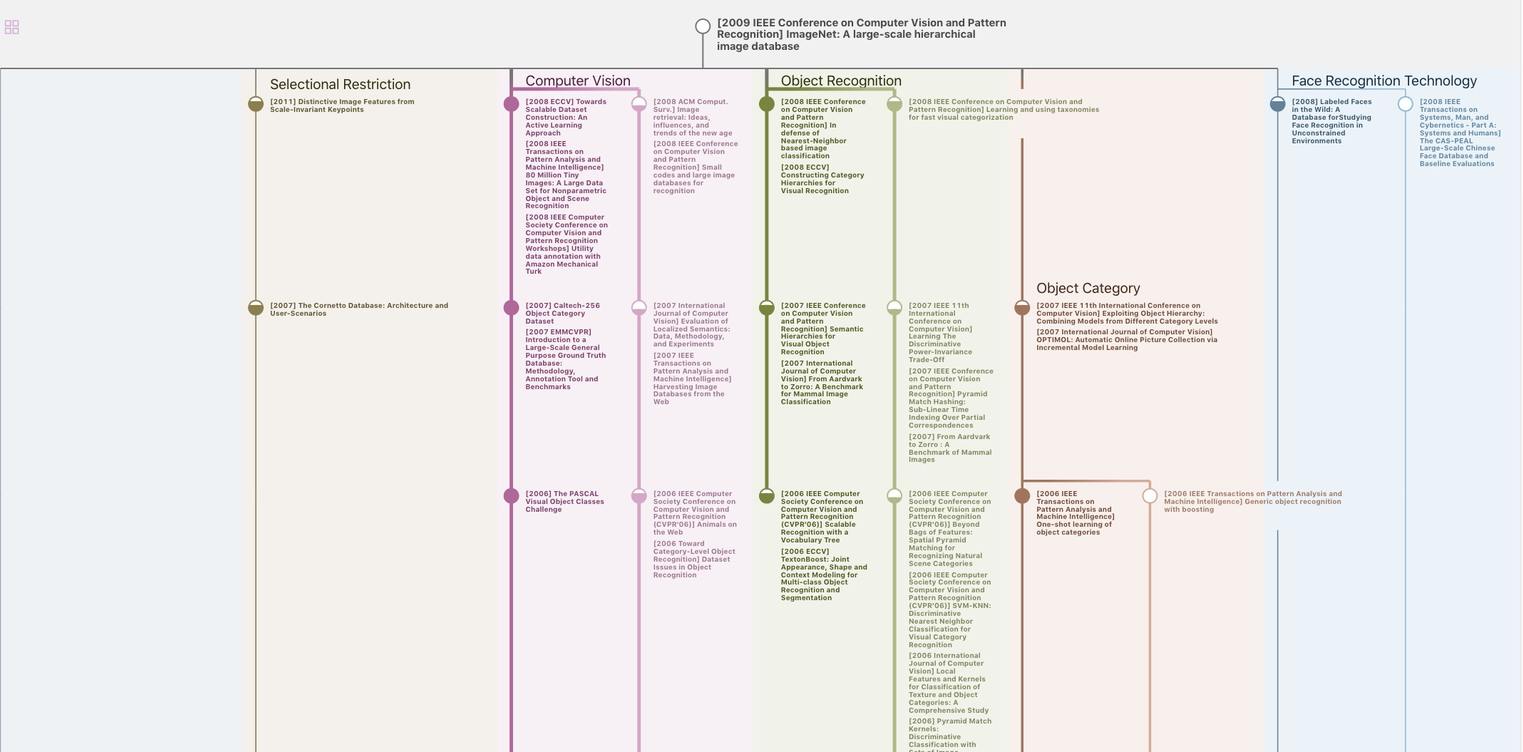
生成溯源树,研究论文发展脉络
Chat Paper
正在生成论文摘要