Discovering biological patterns from short time-series gene expression profiles with integrating PPI data.
Neurocomputing(2014)
摘要
As genes with similar functions are closely related, cluster analysis becomes an important tool to understand and predicts gene functions (patterns) from gene expression profiles. In many situations, each gene expression profile only contains a few data points. Directly applying traditional clustering algorithms to such short gene expression profiles cannot obtain biological meaningful patterns. In this paper, we propose a novel method to discover biologically meaningful patterns by clustering short time-series gene expression profiles with integrating protein–protein interaction (PPI) data. Numerical experiments are conducted on two sets of Arabidopsis thaliana short time-series gene expression profiles, with treatments of cold stress and drought stress, respectively. The proposed method can effectively assign genes belonging to target functional clusters (patterns), in terms of having small p-value of GO term ‘response to cold’ (GO:0009409) in dataset one, and small p-value of GO term ‘response to water deprivation’ (GO:0009414) in dataset two than those from an existing clustering algorithm (namely STEM) for short time-series gene expression profiles. Additionally, our proposed method is able to identify higher percentage of stress-related genes and un-annotated genes in resultant cluster than STEM for both datasets; which does not only improve gene clustering effectiveness, but also contribute to functional prediction of un-annotated genes.
更多查看译文
关键词
Pattern discovery,Cluster analysis,Short time-series gene expression profile,Protein–protein interaction,GO term
AI 理解论文
溯源树
样例
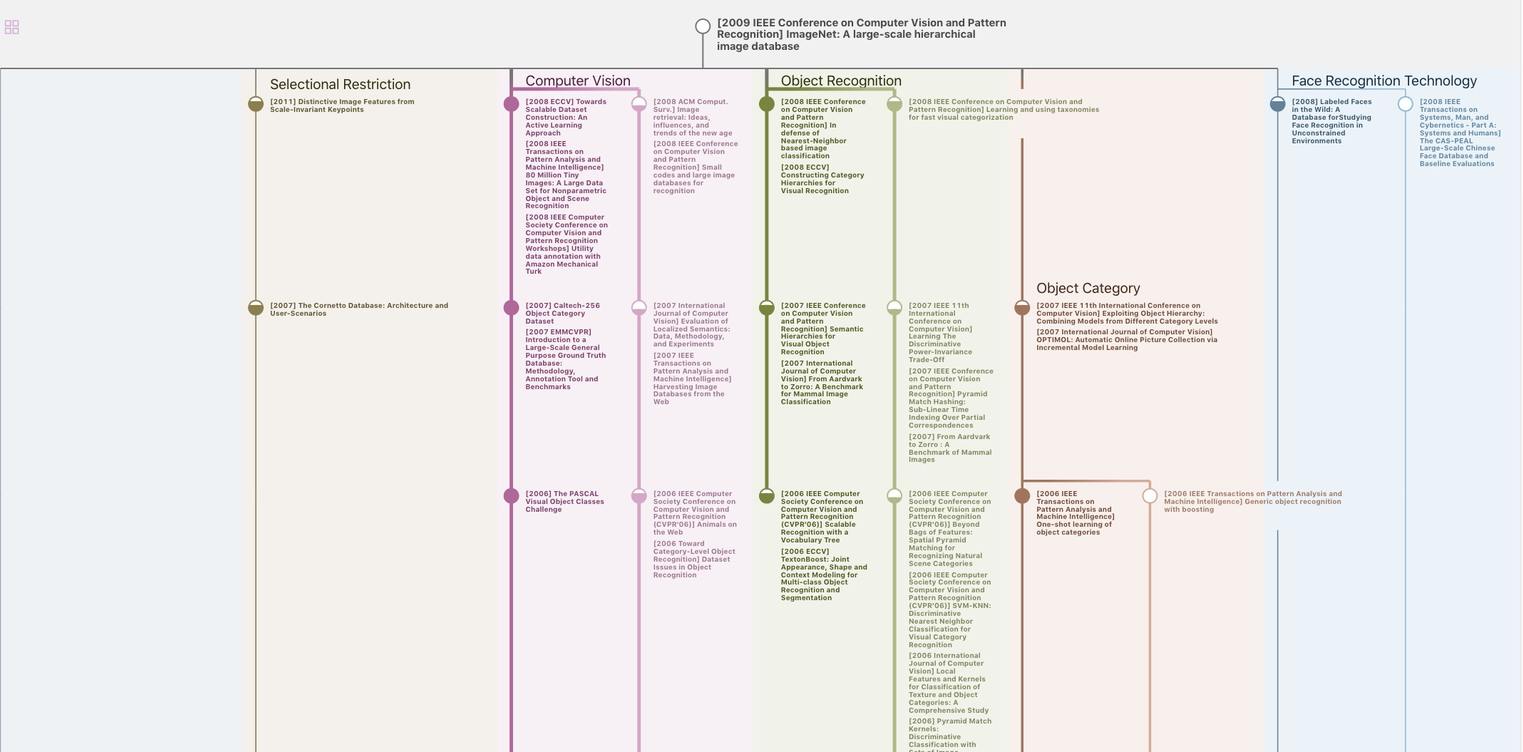
生成溯源树,研究论文发展脉络
Chat Paper
正在生成论文摘要