Binary/ternary extreme learning machines
Neurocomputing(2015)
摘要
In this paper, a new hidden layer construction method for Extreme Learning Machines (ELMs) is investigated, aimed at generating a diverse set of weights. The paper proposes two new ELM variants: Binary ELM, with a weight initialization scheme based on {0,1}–weights; and Ternary ELM, with a weight initialization scheme based on {−1,0,1}–weights. The motivation behind this approach is that these features will be from very different subspaces and therefore each neuron extracts more diverse information from the inputs than neurons with completely random features traditionally used in ELM. Therefore, ideally it should lead to better ELMs. Experiments show that indeed ELMs with ternary weights generally achieve lower test error. Furthermore, the experiments show that the Binary and Ternary ELMs are more robust to irrelevant and noisy variables and are in fact performing implicit variable selection. Finally, since only the weight generation scheme is adapted, the computational time of the ELM is unaffected, and the improved accuracy, added robustness and the implicit variable selection of Binary ELM and Ternary ELM come for free.
更多查看译文
关键词
Extreme learning machine,Hidden layer initialization,Intrinsic plasticity,Random projection,Binary features,Ternary features
AI 理解论文
溯源树
样例
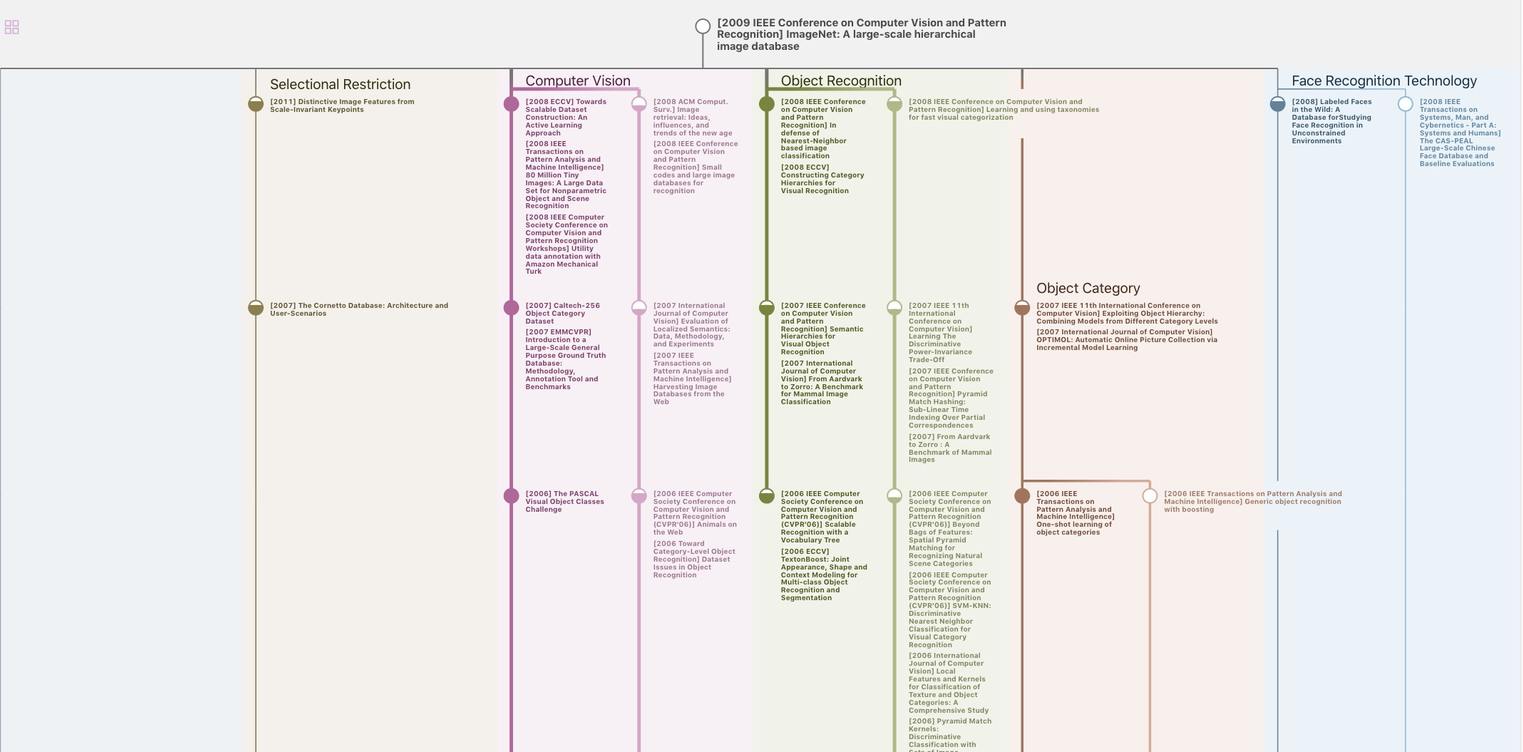
生成溯源树,研究论文发展脉络
Chat Paper
正在生成论文摘要