Training algorithms for Radial Basis Function Networks to tackle learning processes with imbalanced data-sets.
Applied Soft Computing(2014)
摘要
Nowadays, many real applications comprise data-sets where the distribution of the classes is significantly different. These data-sets are commonly known as imbalanced data-sets. Traditional classifiers are not able to deal with these kinds of data-sets because they tend to classify only majority classes, obtaining poor results for minority classes. The approaches that have been proposed to address this problem can be categorized into three types: resampling methods, algorithmic adaptations and cost sensitive techniques. Radial Basis Function Networks (RBFNs), artificial neural networks composed of local models or RBFs, have demonstrated their efficiency in different machine learning areas. Centers, widths and output weights for the RBFs must be determined when designing RBFNs. Taking into account the locally tuned response of RBFs, the objective of this paper is to study the influence of global and local paradigms on the weights training phase, within the RBFNs design methodology, for imbalanced data-sets. Least Mean Square and the Singular Value Decomposition have been chosen as representatives of local and global weights training paradigms respectively. These learning algorithms are inserted into classical RBFN design methods that are run on imbalanced data-sets and also on these data-sets preprocessed with re-balance techniques. After applying statistical tests to the results obtained, some guidelines about the RBFN design methodology for imbalanced data-sets are provided. (C) 2014 Elsevier B.V. All rights reserved.
更多查看译文
关键词
Imbalanced data-sets,Radial Basis Function Networks,Training algorithms,Evolutionary computation
AI 理解论文
溯源树
样例
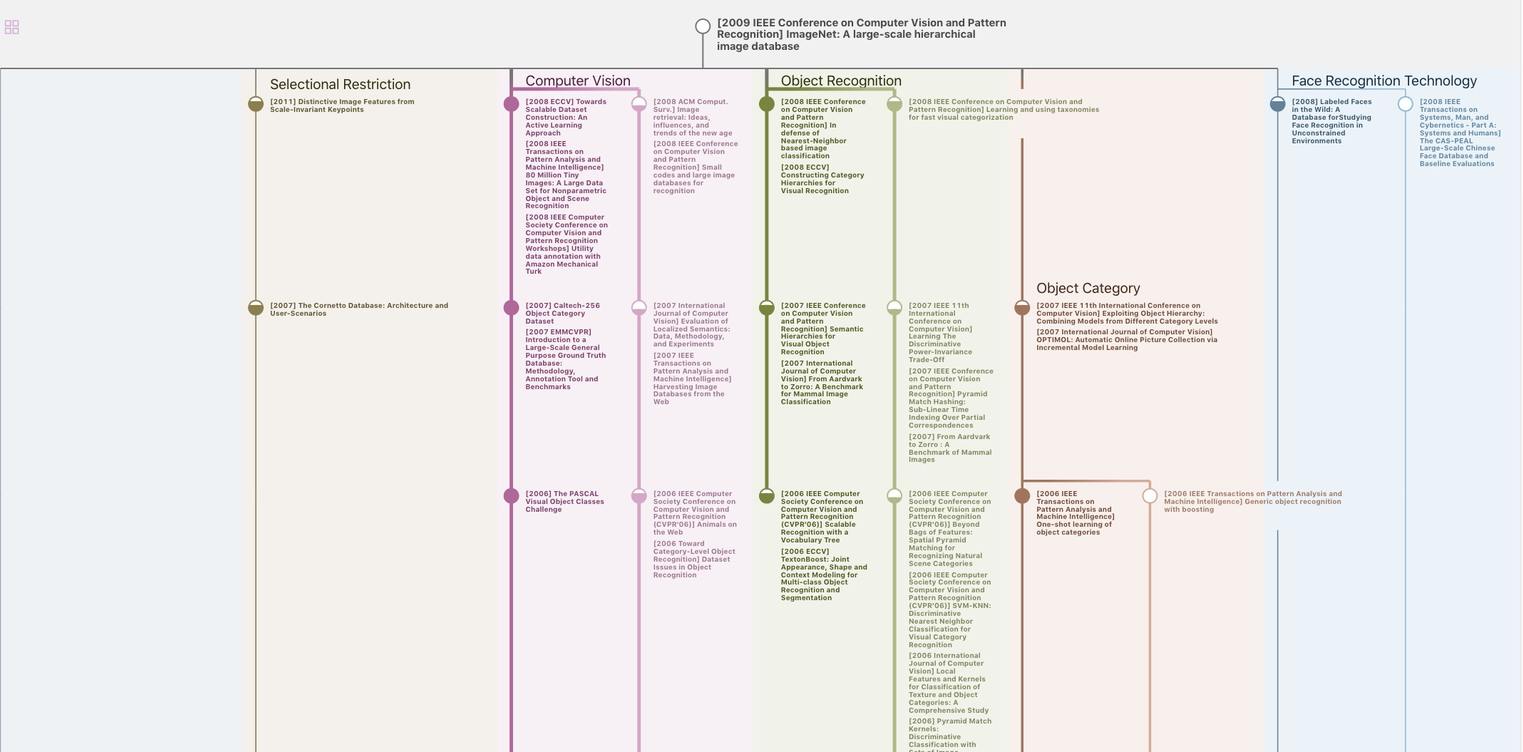
生成溯源树,研究论文发展脉络
Chat Paper
正在生成论文摘要