Semi-Supervised Cross-Media Feature Learning with Unified Patch Graph Regularization
IEEE Trans. Circuits Syst. Video Techn.(2016)
摘要
With the rapid growth of multimedia data such as text, image, video, audio and 3D model, cross-media retrieval has become increasingly important, because users can retrieve the results with various types of media by submitting a query of any media type. Comparing with single-media retrieval such as image retrieval and text retrieval, cross-media retrieval is better because it provides the retrieval results with all kinds of media at the same time. In this paper, we focus on how to learn cross-media features for different media types, which is a key challenge for cross-media retrieval. Existing methods either model different media types separately or only exploit the labeled multimedia data. Actually, data from different media types with the same semantic category are complementary to each other, and jointly modeling them is able to improve the accuracy of cross-media retrieval. In addition, although the labeled data are accurate, they require a lot of human labor and thus are very scarce. To address the above problems, we propose a semi-supervised crossmedia feature learning algorithm with unified patch graph regularization (S2UPG). Our motivation and contributions mainly lie in the following three aspects: (1) Existing methods only model different media types in different graphs, while we employ one joint graph to simultaneously model all the media types. The joint graph is able to fully exploit the semantic correlations among various media types, which are complementary to provide the rich hint for cross-media correlation. (2) Existing methods only consider the original media instances (such as images, videos, texts, audios, and 3D models) but ignore their patches, while we make full use of both the media instances and their patches in one graph. Cross-media patches could emphasize the important parts and make cross-media correlations more precise. (3) Traditional semi-supervised learning methods only exploit single-media unlabeled instances, while our approach f- lly exploits cross-media unlabeled instances and their patches, which can increase the diversity of training data and boost the accuracy of cross-media retrieval. Comparing with the current state-of-theart methods on 3 datasets, including the challenging XMedia dataset with 5 media types, the comprehensive experimental results show that our proposed approach performs better.
更多查看译文
关键词
cross-media retrieval,patch graph regularization,semi-supervised crossmedia feature learning
AI 理解论文
溯源树
样例
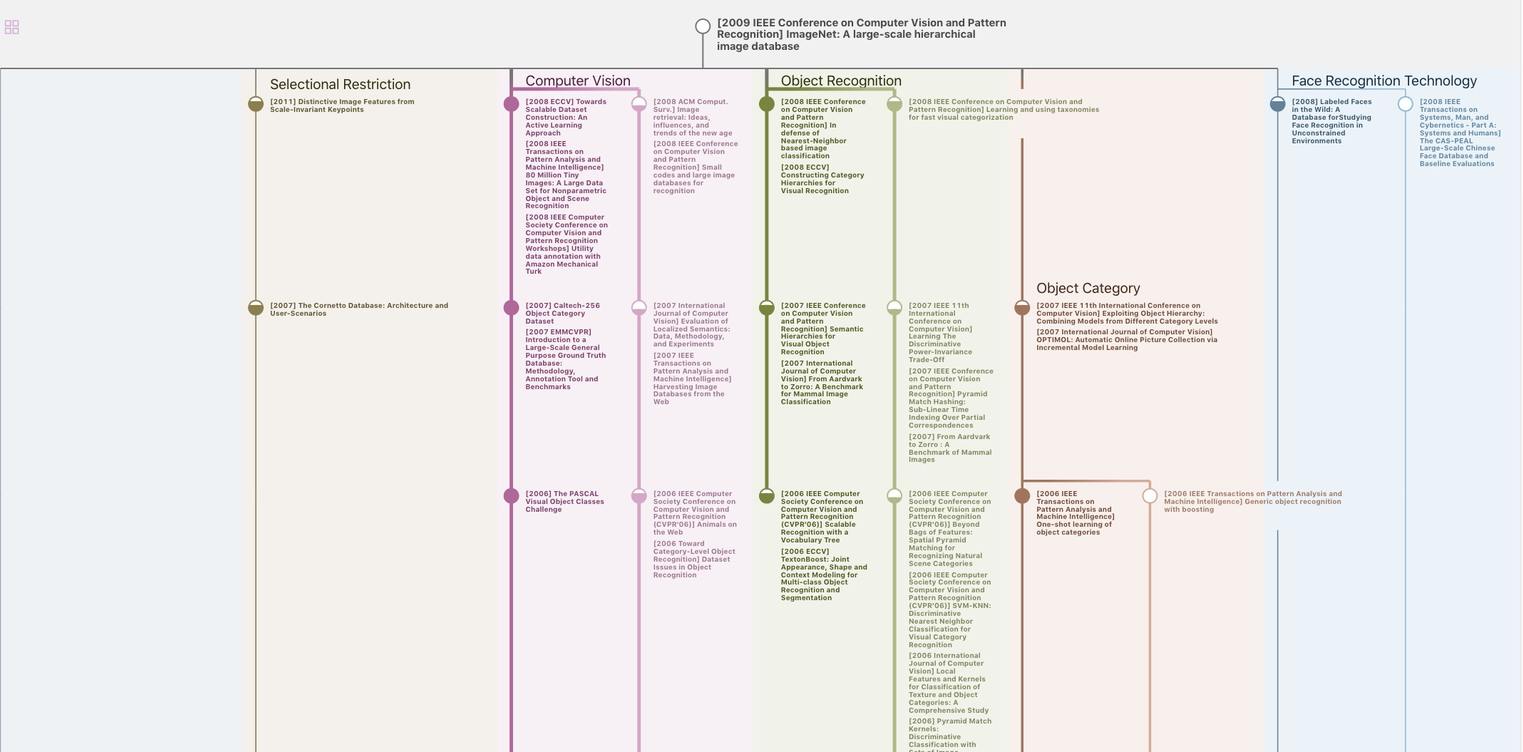
生成溯源树,研究论文发展脉络
Chat Paper
正在生成论文摘要