Bounded Conditional Mean Imputation With Observation Uncertainties And Acoustic Model Adaptation
IEEE Transactions on Audio, Speech, and Language Processing(2015)
摘要
Automatic speech recognition systems use noise compensation and acoustic model adaptation to increase robustness towards speaker and environmental variation. The current work focuses on noise compensation with bounded conditional mean imputation (BCMI). BCMI approaches are missing-data methods which operate on the assumption that noise-corrupted observations can be divided into reliable and unreliable components. BCMI methods substitute the unreliable components with a clean speech posterior distribution. The posterior means can be used as clean speech estimates and the posterior variances can be introduced in acoustic model likelihood calculation as observation uncertainties. In addition, we propose in the current work that similar uncertainties are introduced in acoustic model adaptation. Evaluation with speech data recorded in diverse public and car environments indicates that the proposed uncertainties improve adaptation performance. When uncertainties were used in acoustic model likelihood calculation and adaptation, the proposed imputation and adaptation system introduced 15%-84% relative error reductions to an uncompensated baseline system performance.
更多查看译文
关键词
Acoustic model adaptation,missing data,noise-robust speech recognition,observation uncertainties
AI 理解论文
溯源树
样例
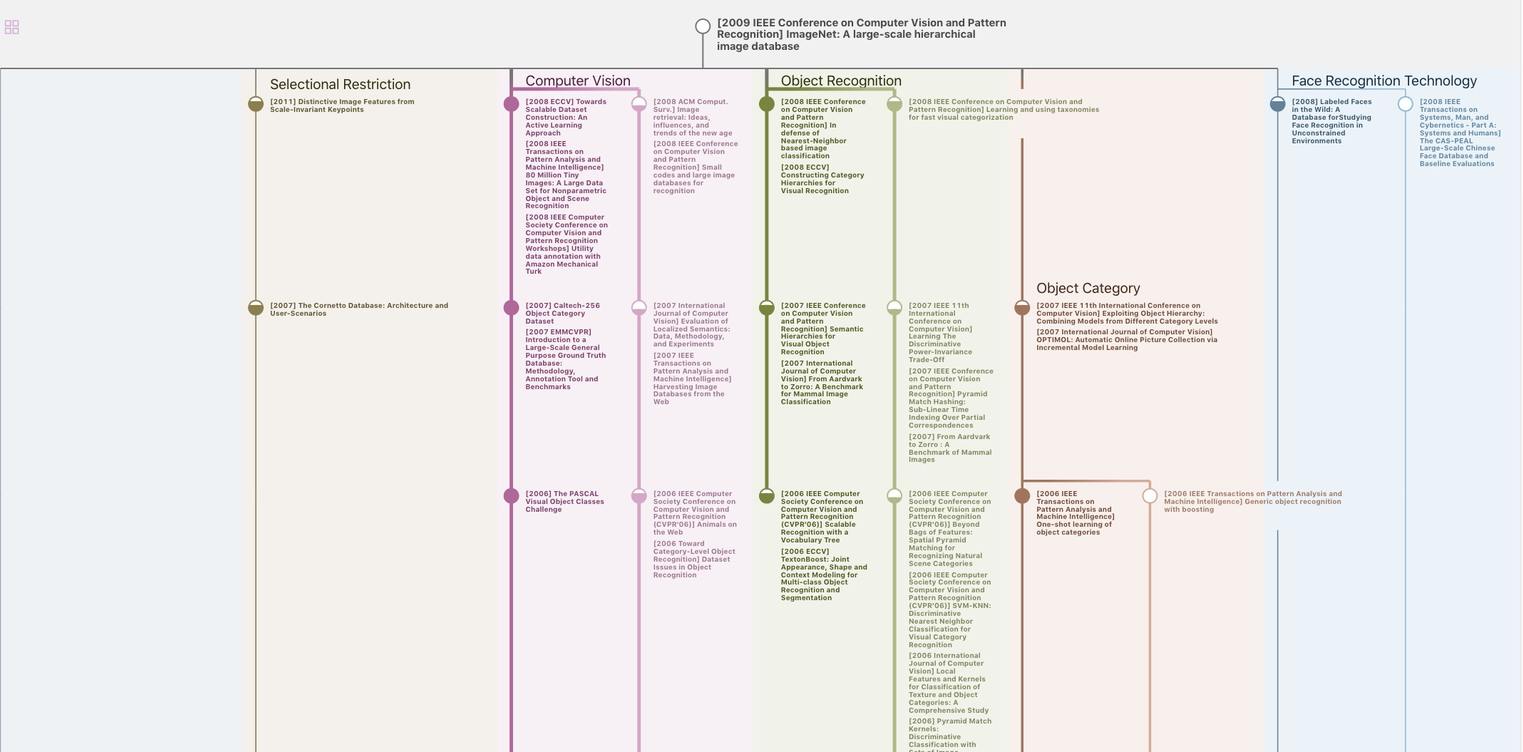
生成溯源树,研究论文发展脉络
Chat Paper
正在生成论文摘要