Maximum Likelihood Passive and Active Sensing of Wideband Power Spectra From Few Bits
Signal Processing, IEEE Transactions (2015)
摘要
Wideband power spectrum sensing is essential for cognitive radio and many other applications. Aiming to crowdsource spectrum sensing operations, a novel frugal sensing framework was recently proposed, employing a network of low duty-cycle sensors (e.g., running in background mode on consumer devices) reporting randomly filtered broadband power measurement bits to a fusion center, which in turn estimates the ambient power spectrum. Frugal sensing is revisited here from a statistical estimation point of view. Taking into account fading and insufficient sample averaging considerations, maximum likelihood (ML) formulations are developed which outperform the original minimum power and interior point solutions when the soft power estimates prior to thresholding are noisy. Assuming availability of a downlink channel that the fusion center can use to send threshold information, active sensing strategies are developed that quickly narrow down and track the power spectrum estimate, using ideas borrowed from cutting plane methods to develop active ML solutions. Simulations show that satisfactory wideband power spectrum estimates can be obtained with passive ML sensing from few bits, and much better performance can be attained using active sensing. Various other aspects, such as known emitter spectral shapes and different types of non-negativity constraints, are also considered.
更多查看译文
关键词
cognitive radio,maximum likelihood estimation,spread spectrum communication,statistical analysis,active sensing strategies,ambient power spectrum,crowdsource spectrum sensing,frugal sensing framework,low duty-cycle sensors,maximum likelihood active sensing,maximum likelihood passive sensing,randomly filtered broadband power measurement,statistical estimation,wideband power spectrum sensing,collaborative sensing,spectral analysis,spectrum sensing,fading,sensors,correlation,gaussian distribution
AI 理解论文
溯源树
样例
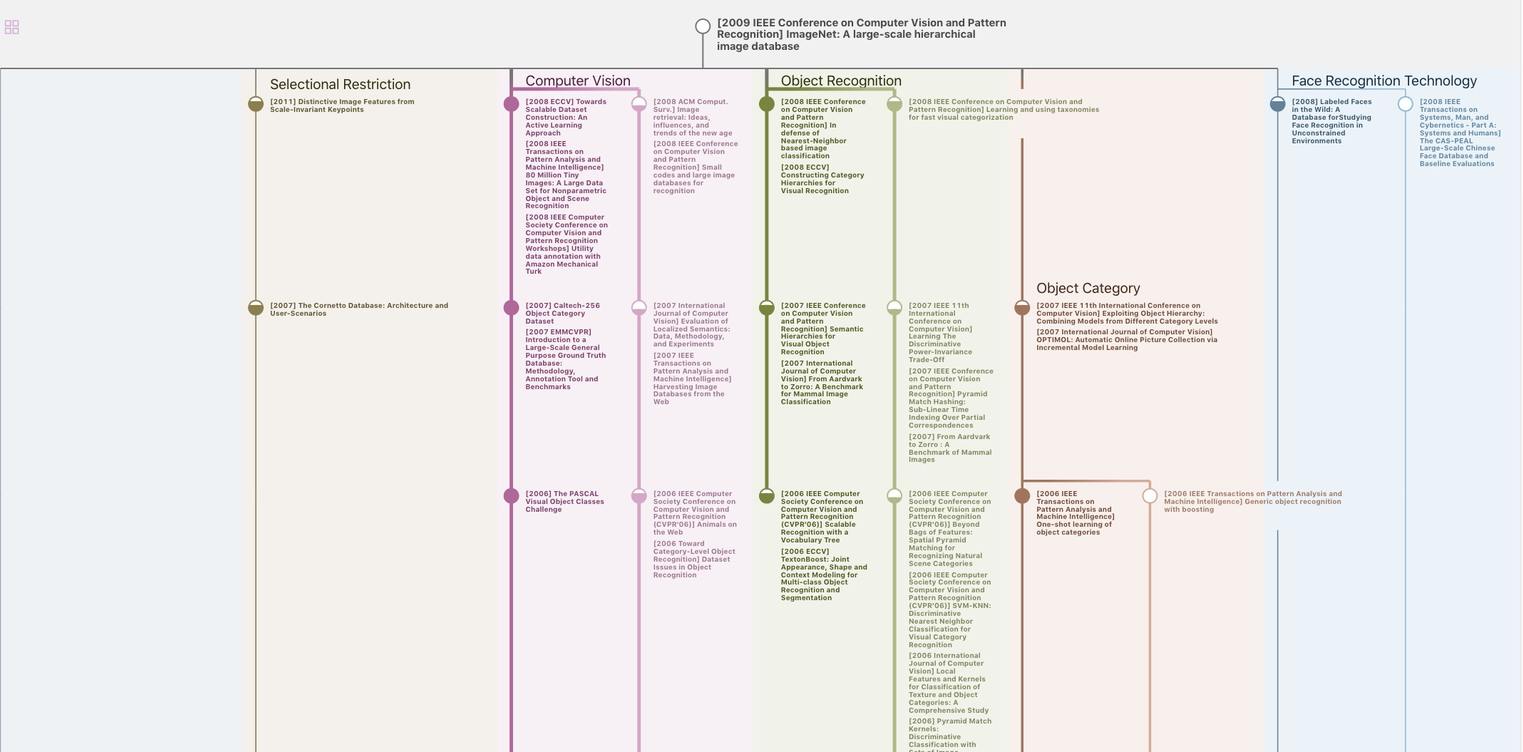
生成溯源树,研究论文发展脉络
Chat Paper
正在生成论文摘要