Multi-view and 3D Deformable Part Models
Pattern Analysis and Machine Intelligence, IEEE Transactions (2015)
摘要
As objects are inherently 3-dimensional, they have been modeled in 3D in the early days of computer vision. Due to the ambiguities arising from mapping 2D features to 3D models, 3D object representations have been neglected and 2D feature-based models are the predominant paradigm in object detection nowadays. While such models have achieved outstanding bounding box detection performance, they come with limited expressiveness, as they are clearly limited in their capability of reasoning about 3D shape or viewpoints. In this work, we bring the worlds of 3D and 2D object representations closer, by building an object detector which leverages the expressive power of 3D object representations while at the same time can be robustly matched to image evidence. To that end, we gradually extend the successful deformable part model [1] to include viewpoint information and part-level 3D geometry information, resulting in several different models with different level of expressiveness. We end up with a 3D object model, consisting of multiple object parts represented in 3D and a continuous appearance model. We experimentally verify that our models, while providing richer object hypotheses than the 2D object models, provide consistently better joint object localization and viewpoint estimation than the state-of-the-art multi-view and 3D object detectors on various benchmarks (KITTI [2], 3D object classes [3], Pascal3D+ [4], Pascal VOC 2007 [5], EPFL multi-view cars [6]).
更多查看译文
关键词
3d object models,object detection,deformable part models,structured output learning,detectors,feature extraction,solid modeling,geometry
AI 理解论文
溯源树
样例
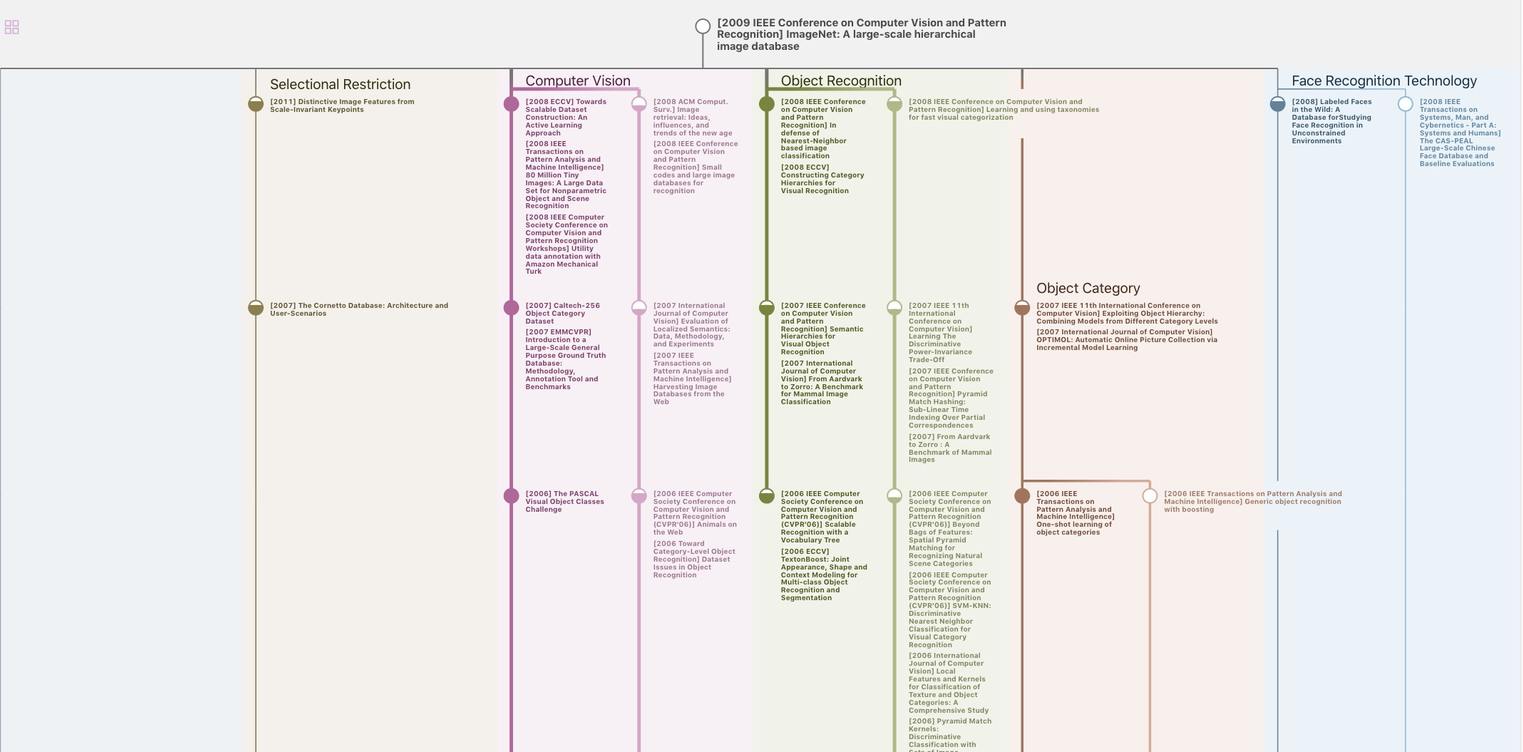
生成溯源树,研究论文发展脉络
Chat Paper
正在生成论文摘要