Learning Efficient Sparse and Low Rank Models.
IEEE Transactions on Pattern Analysis and Machine Intelligence(2015)
摘要
Parsimony, including sparsity and low rank, has been shown to successfully model data in numerous machine learning and signal processing tasks. Traditionally, such modeling approaches rely on an iterative algorithm that minimizes an objective function with parsimony-promoting terms. The inherently sequential structure and data-dependent complexity and latency of iterative optimization constitute a major limitation in many applications requiring real-time performance or involving large-scale data. Another limitation encountered by these modeling techniques is the difficulty of their inclusion in discriminative learning scenarios. In this work, we propose to move the emphasis from the model to the pursuit algorithm, and develop a process-centric view of parsimonious modeling, in which a learned deterministic fixed-complexity pursuit process is used in lieu of iterative optimization. We show a principled way to construct learnable pursuit process architectures for structured sparse and robust low rank models, derived from the iteration of proximal descent algorithms. These architectures learn to approximate the exact parsimonious representation at a fraction of the complexity of the standard optimization methods. We also show that appropriate training regimes allow to naturally extend parsimonious models to discriminative settings. State-of-the-art results are demonstrated on several challenging problems in image and audio processing with several orders of magnitude speed-up compared to the exact optimization algorithms.
更多查看译文
关键词
nmf,big data,deep learning,parsimonious modeling,proximal methods,real-time implementations,sparse and low-rank models
AI 理解论文
溯源树
样例
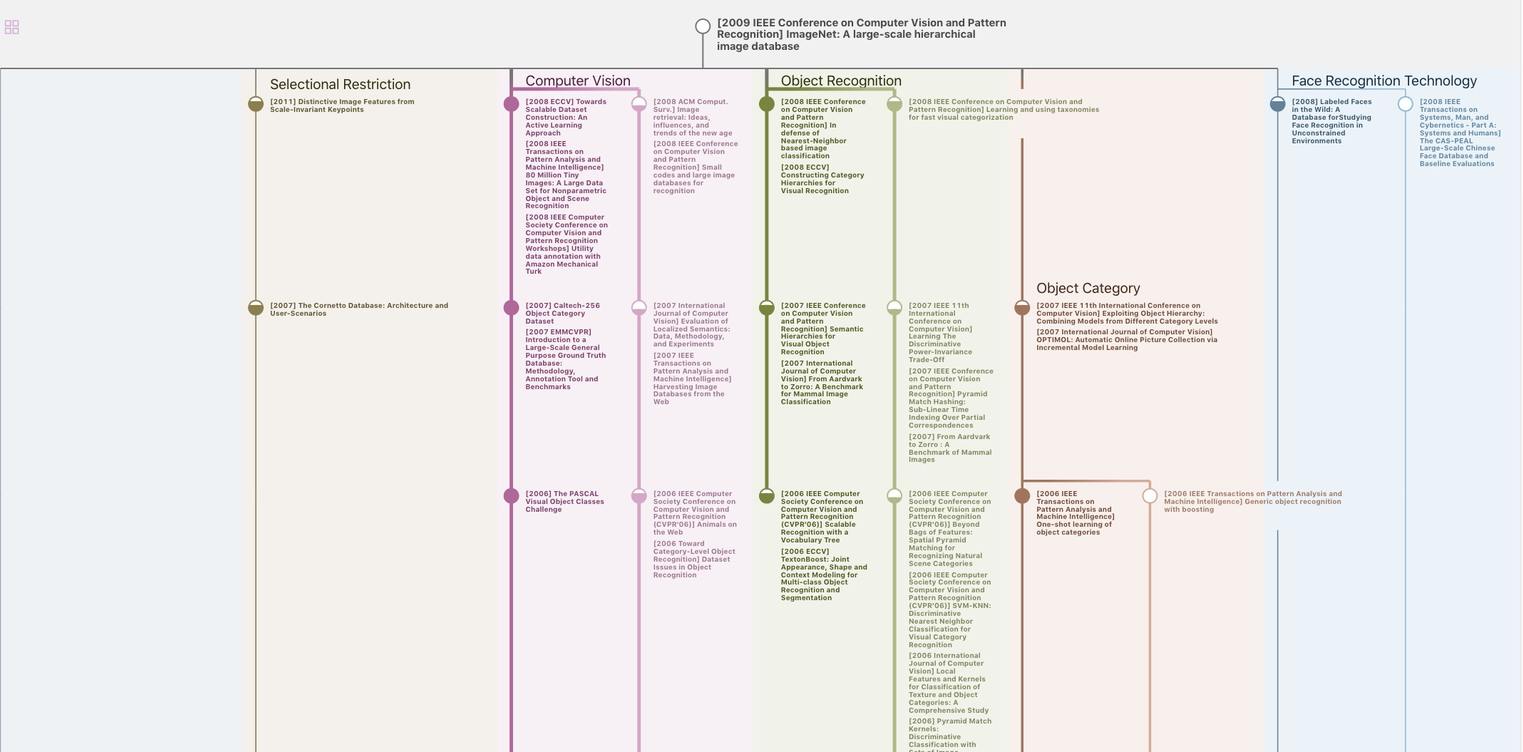
生成溯源树,研究论文发展脉络
Chat Paper
正在生成论文摘要