Elimination of Outliers from 2-D Point Sets Using the Helmholtz Principle
Signal Processing Letters, IEEE (2015)
摘要
A method for modeling and removing outliers from 2-D sets of scattered points is presented. The method relies on a principle due to Helmholtz stating that every large deviation from uniform noise should be perceptible, provided that the deviation is generated by an a contrario model of geometric structures. By assuming local linearity, we first employ a robust algorithm to model the local manifold of the corrupted data by local line segments. Our rationale is that long line segments should not be expected in a noisy set of points. This assumption leads to the modeling of the lengths of the line segments by a Pareto distribution, which is the adopted a contrario model for the observations. The model is successfully evaluated on two problems in computer vision: shape recovery and linear regression.
更多查看译文
关键词
linear regression,outlier modeling,point cloud,shape detection,computational modeling,shape,manifolds,computer vision,data models,noise
AI 理解论文
溯源树
样例
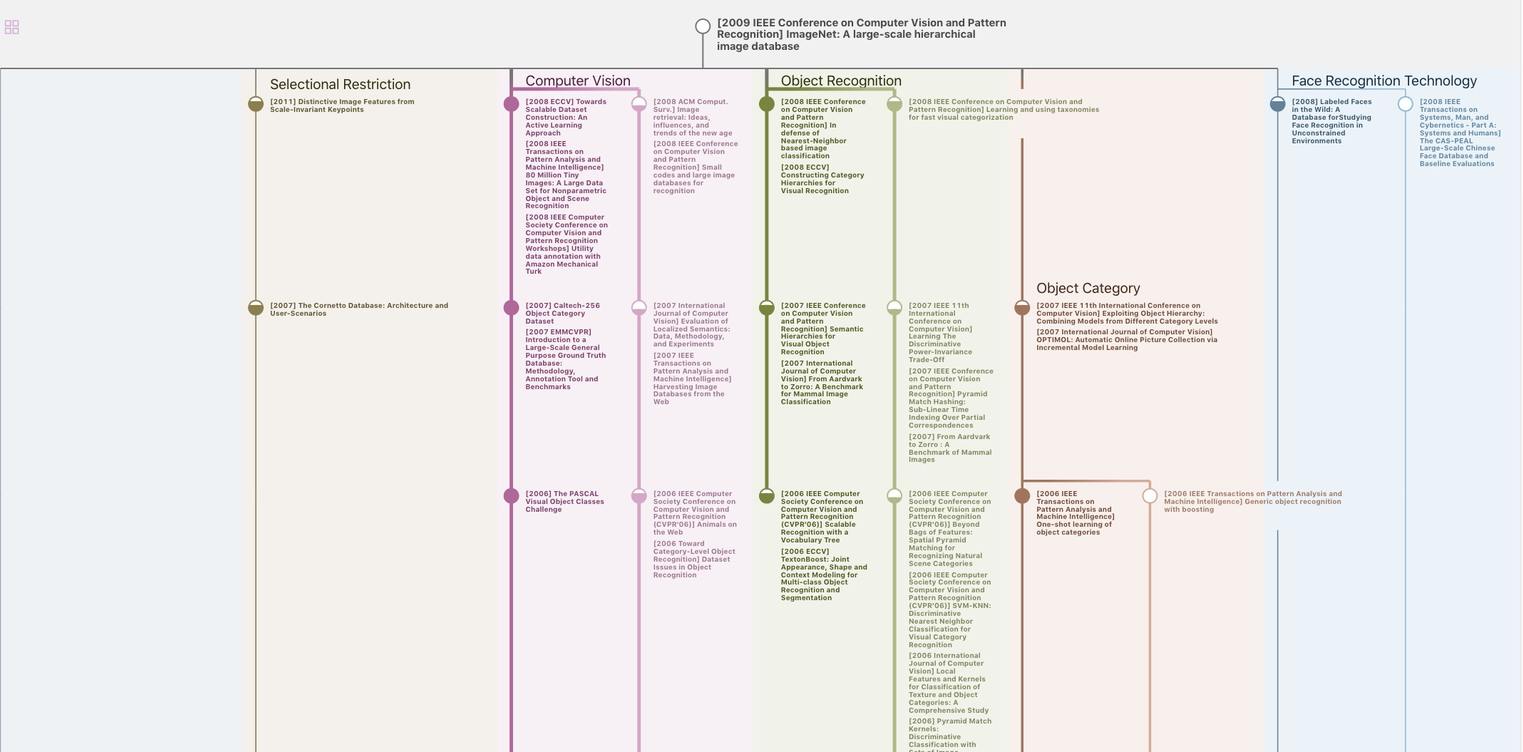
生成溯源树,研究论文发展脉络
Chat Paper
正在生成论文摘要