Multi-view Intact Space Learning
IEEE Transactions on Pattern Analysis and Machine Intelligence(2019)
摘要
It is practical to assume that an individual view is unlikely to be sufficient for effective multi-view learning. Therefore, integration of multi-view information is both valuable and necessary. In this paper, we propose the Multi-view Intact Space Learning (MISL) algorithm, which integrates the encoded complementary information in multiple views to discover a latent intact representation of the data. Even though each view on its own is insufficient, we show theoretically that by combing multiple views we can obtain abundant information for latent intact space learning. Employing the Cauchy loss (a technique used in statistical learning) as the error measurement strengthens robustness to outliers. We propose a new definition of multi-view stability and then derive the generalization error bound based on multi-view stability and Rademacher complexity, and show that the complementarity between multiple views is beneficial for the stability and generalization. MISL is efficiently optimized using a novel Iteratively Reweight Residuals (IRR) technique, whose convergence is theoretically analyzed. Experiments on synthetic data and real-world datasets demonstrate that MISL is an effective and promising algorithm for practical applications.
更多查看译文
关键词
multi-view learning,robust algorithms
AI 理解论文
溯源树
样例
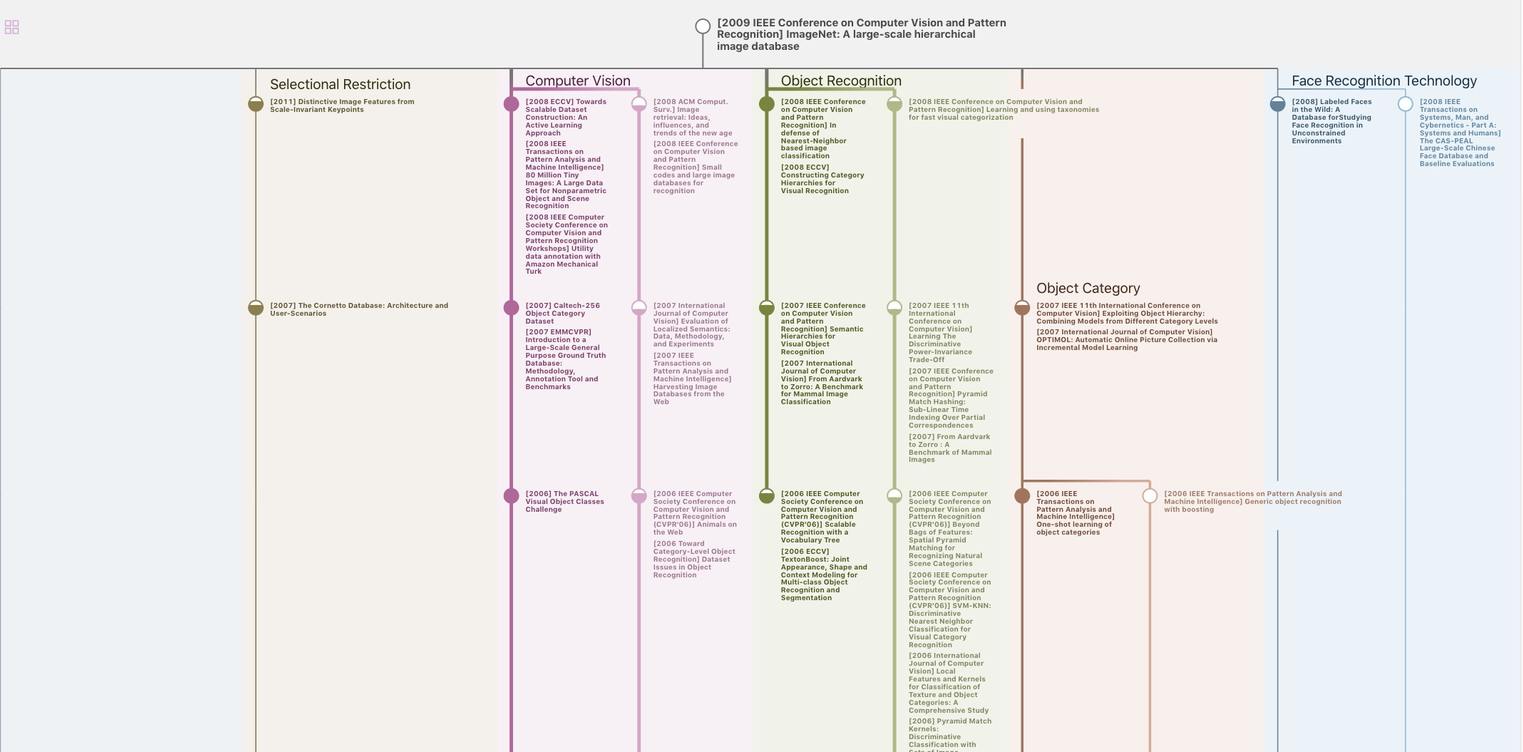
生成溯源树,研究论文发展脉络
Chat Paper
正在生成论文摘要