Sparse DNN-based speaker segmentation using side information
Electronics Letters (2015)
摘要
Sparse deep neural networks (SDNNs) for speaker segmentation are proposed. First, the SDNNs are trained using the side information that is the class label of the input. Then, speaker-specific features are extracted from the super-vector feature of the speech signal by the SDNNs. Lastly, the label of each speech frame is obtained by K-means clustering, which is used to segment different speakers of a continuous speech stream. The performance evaluation using the multi-speaker speech stream corpus generated from the TIMIT database shows that the proposed speaker segmentation algorithm outperforms the Bayesian information criterion method and the deep auto-encoder networks method.
更多查看译文
关键词
bayes methods,audio databases,feature extraction,neural nets,pattern clustering,speaker recognition,bic method,bayesian information criterion method,sdnn,timit database,continuous speech stream,deep auto-encoder networks method,input class label,k-means clustering,multispeaker speech stream corpus,side information,sparse dnn-based speaker segmentation,sparse deep neural networks,speaker-specific feature extraction,speech frame,speech signal,supervector feature,k means clustering
AI 理解论文
溯源树
样例
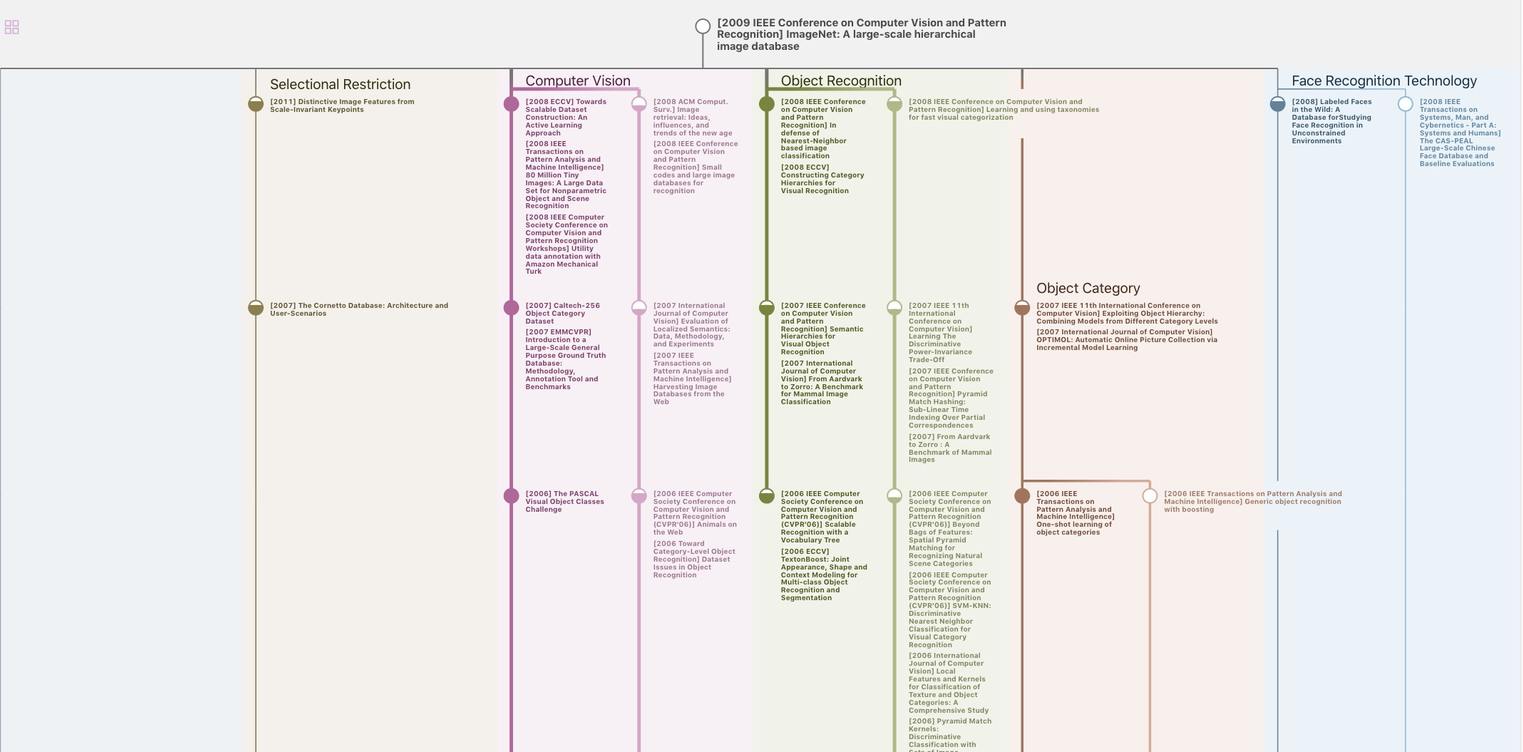
生成溯源树,研究论文发展脉络
Chat Paper
正在生成论文摘要