Time-Series Classification with COTE: The Collective of Transformation-Based Ensembles
IEEE Trans. Knowl. Data Eng.(2016)
摘要
Recently, two ideas have been explored that lead to more accurate algorithms for time-series classification (TSC). First, it has been shown that the simplest way to gain improvement on TSC problems is to transform into an alternative data space where discriminatory features are more easily detected. Second, it was demonstrated that with a single data representation, improved accuracy can be achieved through simple ensemble schemes. We combine these two principles to test the hypothesis that forming a collective of ensembles of classifiers on different data transformations improves the accuracy of time-series classification. The collective contains classifiers constructed in the time, frequency, change, and shapelet transformation domains. For the time domain we use a set of elastic distance measures. For the other domains we use a range of standard classifiers. Through extensive experimentation on 72 datasets, including all of the 46 UCR datasets, we demonstrate that the simple collective formed by including all classifiers in one ensemble is significantly more accurate than any of its components and any other previously published TSC algorithm. We investigate alternative hierarchical collective structures and demonstrate the utility of the approach on a new problem involving classifying Caenorhabditis elegans mutant types.
更多查看译文
关键词
correlation,accuracy,frequency domain analysis,time series analysis
AI 理解论文
溯源树
样例
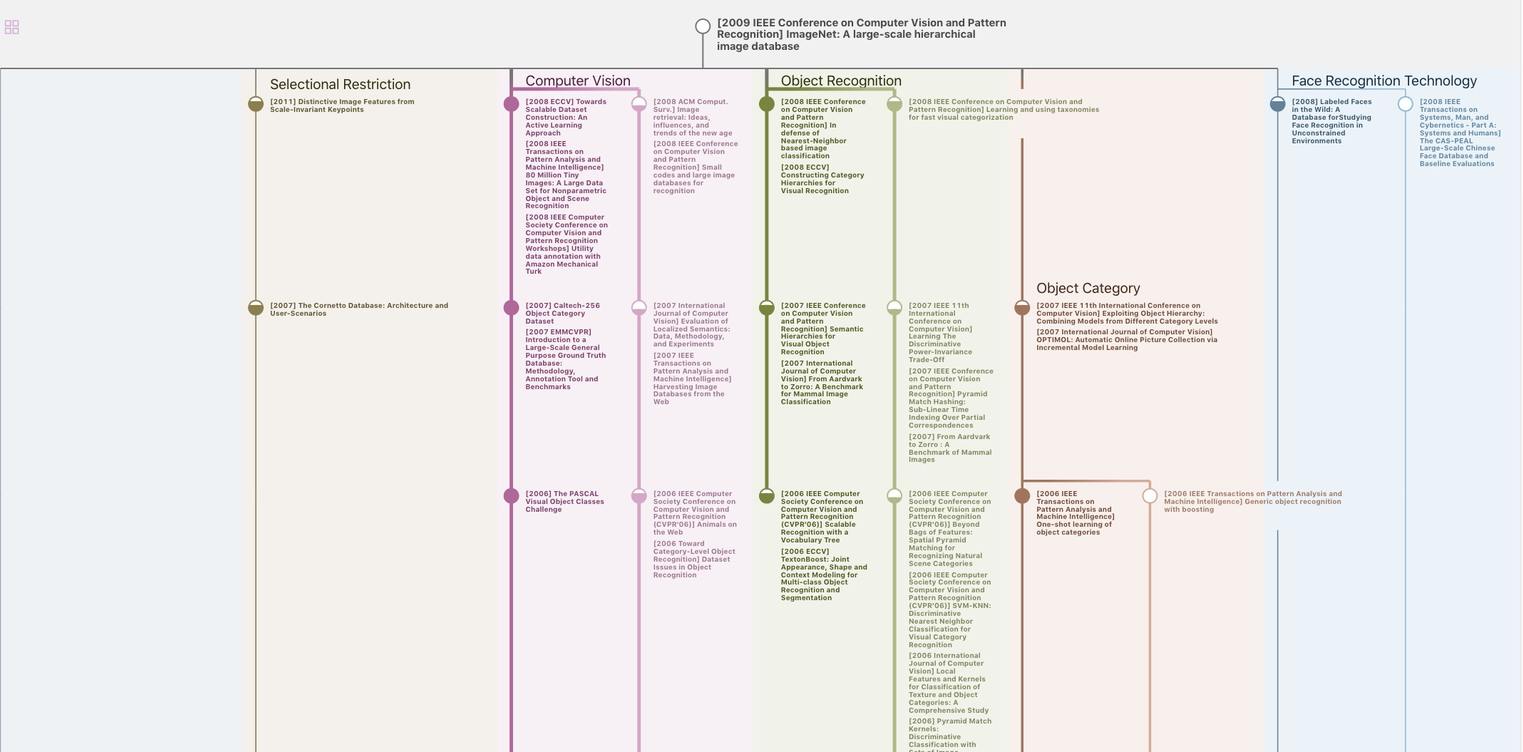
生成溯源树,研究论文发展脉络
Chat Paper
正在生成论文摘要