Bayesian Nonparametric Inverse Reinforcement Learning for Switched Markov Decision Processes
Machine Learning and Applications(2014)
摘要
In this paper we develop a Bayesian nonparametric Inverse Reinforcement Learning technique for switched Markov Decision Processes (MDP). Similar to switched linear dynamical systems, switched MDP (sMDP) can be used to represent complex behaviors composed of temporal transitions between simpler behaviors each represented by a standard MDP. We use sticky Hierarchical Dirichlet Process as a nonparametric prior on the sMDP model space, and describe a Markov Chain Monte Carlo method to efficiently learn the posterior given the behavior data. We demonstrate the effectiveness of sMDP models for learning, prediction and classification of complex agent behaviors in a simulated surveillance scenario.
更多查看译文
关键词
Bayes methods,Markov processes,Monte Carlo methods,decision making,learning (artificial intelligence),nonparametric statistics,pattern classification,Bayesian nonparametric inverse reinforcement learning technique,Markov chain Monte Carlo method,hierarchical Dirichlet process,sMDP model space,simulated surveillance scenario,switched MDP,switched Markov decision processes,switched linear dynamical systems,Bayesian Nonparametrics,Inverse Reinforcement Learning,Markov Decision Processes
AI 理解论文
溯源树
样例
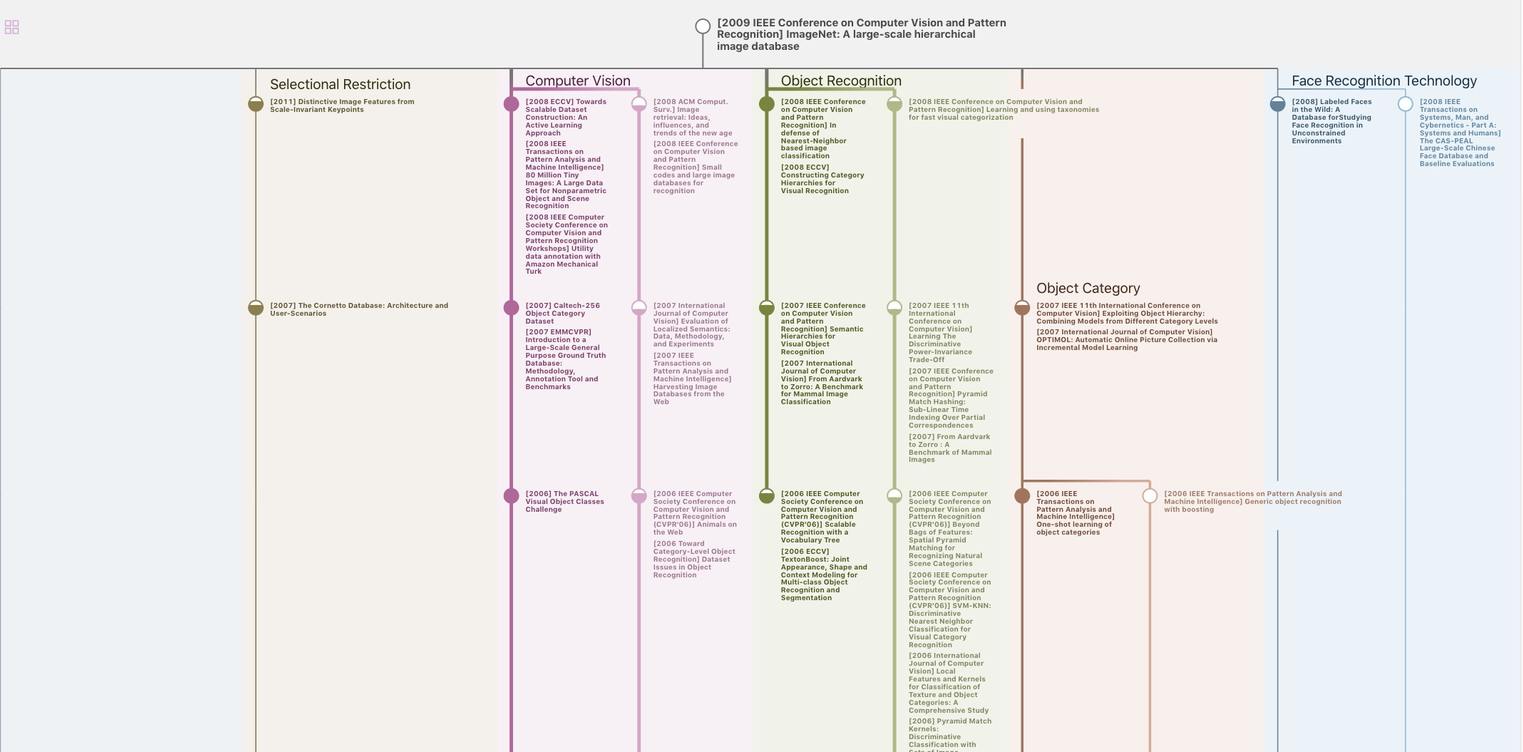
生成溯源树,研究论文发展脉络
Chat Paper
正在生成论文摘要