Multi-view Clustering via Multi-manifold Regularized Nonnegative Matrix Factorization
Data Mining(2014)
摘要
Multi-view clustering integrates complementary information from multiple views to gain better clustering performance rather than relying on a single view. NMF based multi-view clustering algorithms have shown their competitiveness among different multi-view clustering algorithms. However, NMF fails to preserve the locally geometrical structure of the data space. In this paper, we propose a multi-manifold regularized nonnegative matrix factorization framework (MMNMF) which can preserve the locally geometrical structure of the manifolds for multi-view clustering. MMNMF regards that the intrinsic manifold of the dataset is embedded in a convex hull of all the views' manifolds, and incorporates such an intrinsic manifold and an intrinsic (consistent) coefficient matrix with a multi-manifold regularizer to preserve the locally geometrical structure of the multi-view data space. We use linear combination to construct the intrinsic manifold, and propose two strategies to find the intrinsic coefficient matrix, which lead to two instances of the framework. Experimental results show that the proposed algorithms outperform existing NMF based algorithms for multi-view clustering.
更多查看译文
关键词
pattern clustering,intrinsic coefficient matrix,multiview clustering,multimanifold regularized nonnegative matrix factorization,geometrical structure,matrix decomposition,linear combination,mmnmf,linear programming,manifolds,clustering algorithms,convergence
AI 理解论文
溯源树
样例
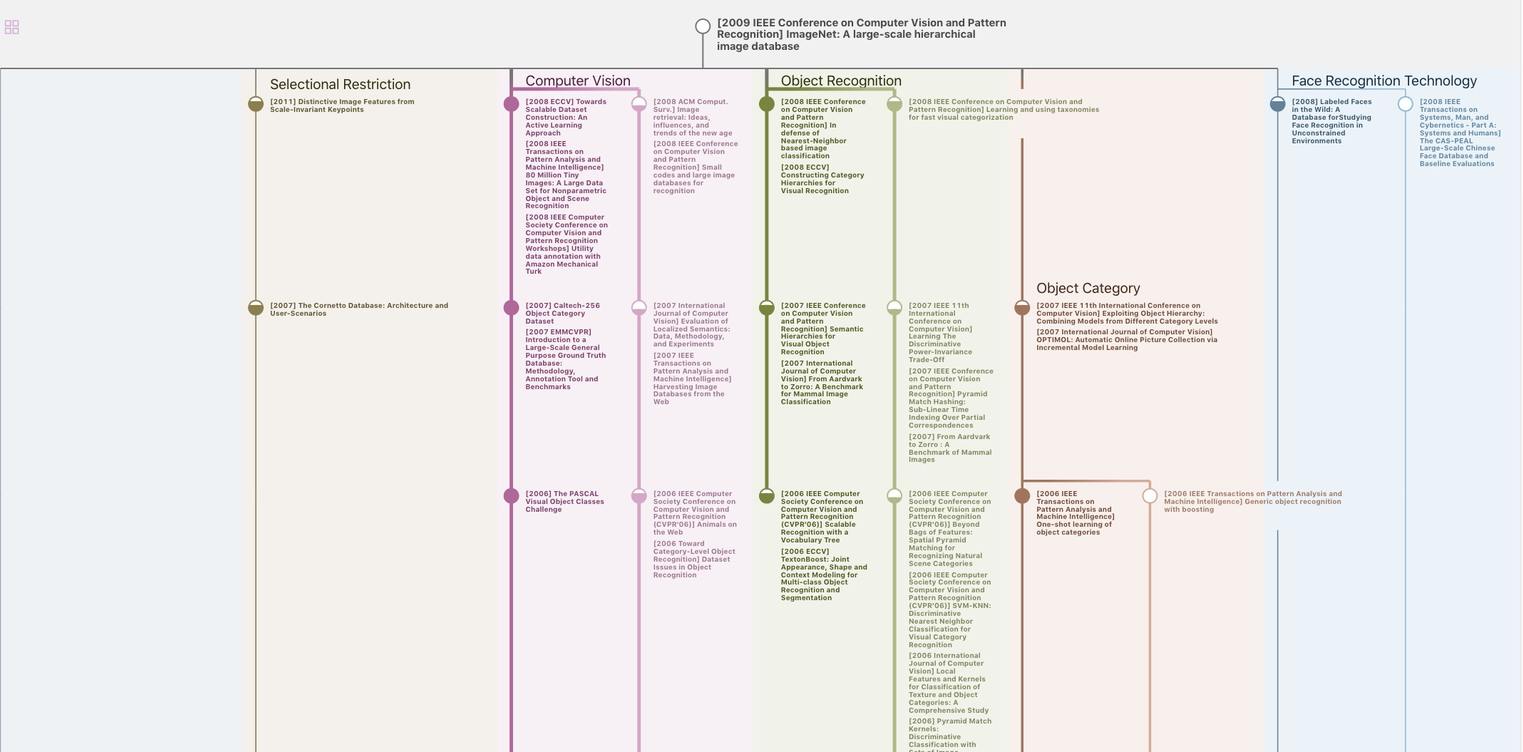
生成溯源树,研究论文发展脉络
Chat Paper
正在生成论文摘要