Hashtag Graph Based Topic Model for Tweet Mining
Data Mining(2014)
摘要
Mining topics in Twitter is increasingly attracting more attention. However, the shortness and informality of tweets leads to extreme sparse vector representation with a large vocabulary, which makes the conventional topic models (e.g., Latent Dirichlet Allocation) often fail to achieve high quality underlying topics. Luckily, tweets always show up with rich user-generated hash tags as keywords. In this paper, we propose a novel topic model to handle such semi-structured tweets, denoted as Hash tag Graph based Topic Model (HGTM). By utilizing relation information between hash tags in our hash tag graph, HGTM establishes word semantic relations, even if they haven't co-occurred within a specific tweet. In addition, we enhance the dependencies of both multiple words and hash tags via latent variables (topics) modeled by HGTM. We illustrate that the user-contributed hash tags could serve as weakly-supervised information for topic modeling, and hash tag relation could reveal the semantic relation between tweets. Experiments on a real-world twitter data set show that our model provides an effective solution to discover more distinct and coherent topics than the state-of-the-art baselines and has a strong ability to control sparseness and noise in tweets.
更多查看译文
关键词
twitter data set,word semantic relations,extreme sparse vector representation,topics mining,semistructured tweets,weakly supervised information,hash tag graph based topic model,topic modeling,user-generated hash tags,keywords,data mining,hash tag relation,social networking (online),tweet mining,hgtm,latent variables,vectors,semantics,mathematical model,data models
AI 理解论文
溯源树
样例
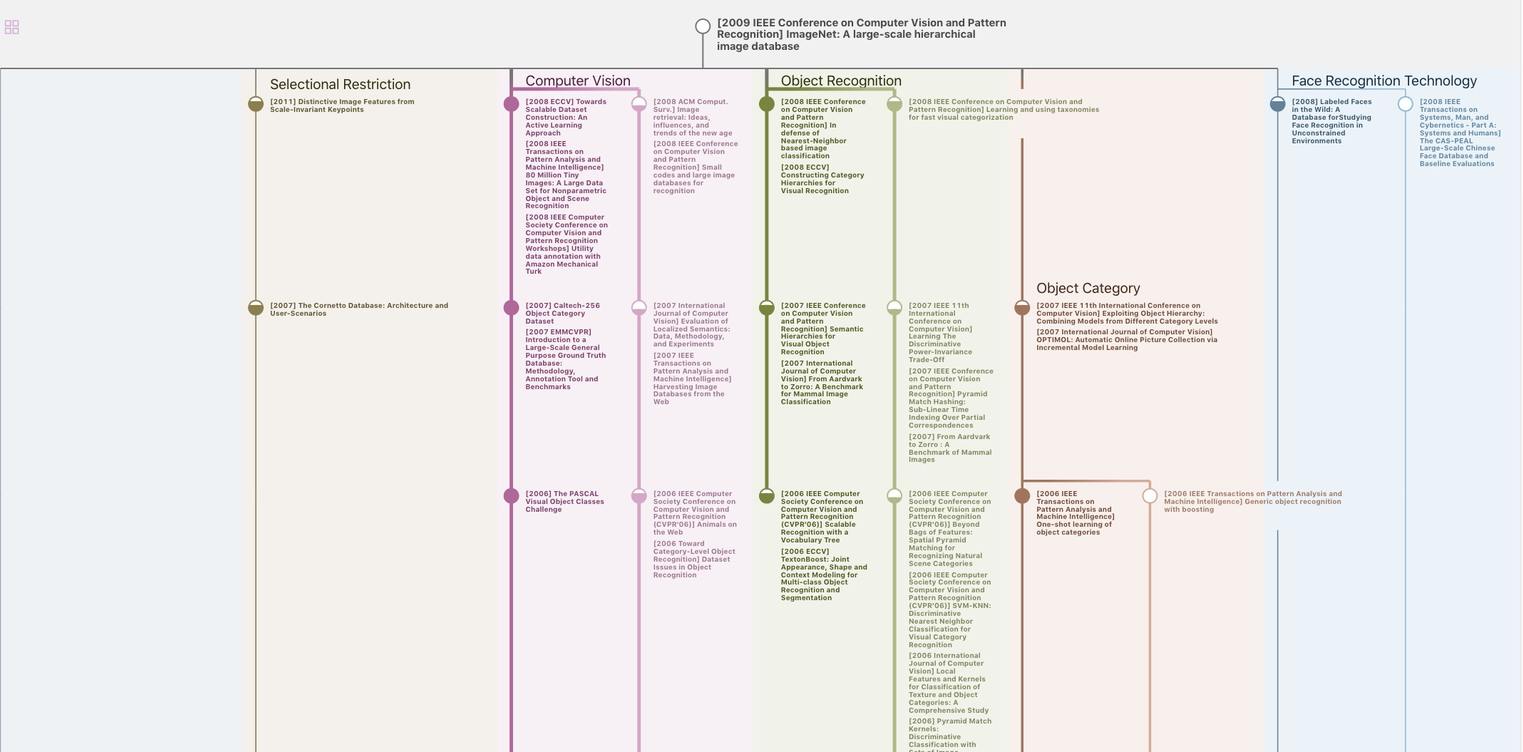
生成溯源树,研究论文发展脉络
Chat Paper
正在生成论文摘要