Computer-aided diagnosis of mammographic masses using scalable image retrieval.
IEEE Trans. Biomed. Engineering(2015)
摘要
Computer-aided diagnosis of masses in mammograms is important to the prevention of breast cancer. Many approaches tackle this problem through content-based image retrieval techniques. However, most of them fall short of scalability in the retrieval stage, and their diagnostic accuracy is, therefore, restricted. To overcome this drawback, we propose a scalable method for retrieval and diagnosis of mammographic masses. Specifically, for a query mammographic region of interest (ROI), scale-invariant feature transform (SIFT) features are extracted and searched in a vocabulary tree, which stores all the quantized features of previously diagnosed mammographic ROIs. In addition, to fully exert the discriminative power of SIFT features, contextual information in the vocabulary tree is employed to refine the weights of tree nodes. The retrieved ROIs are then used to determine whether the query ROI contains a mass. The presented method has excellent scalability due to the low spatial-temporal cost of vocabulary tree. Extensive experiments are conducted on a large dataset of 11 553 ROIs extracted from the digital database for screening mammography, which demonstrate the accuracy and scalability of our approach.
更多查看译文
关键词
mammography,sift feature,query mammographic region of interest,retrieval stage,contextual information,data analysis,content-based image retrieval (cbir),breast masses,image retrieval technique,mammographic mass diagnosis,mammographic mass retrieval,diagnostic accuracy,query roi,cancer,breast cancer prevention,mammographic mass computer-aided diagnosis,mammographic roi,image retrieval,scalable image retrieval,mammogram,scale-invariant feature transform,transforms,tree node weight,digital database,vocabulary tree low spatial-temporal cost,retrieved roi,computer-aided diagnosis (cad),visualization,design automation,biomedical imaging,feature extraction
AI 理解论文
溯源树
样例
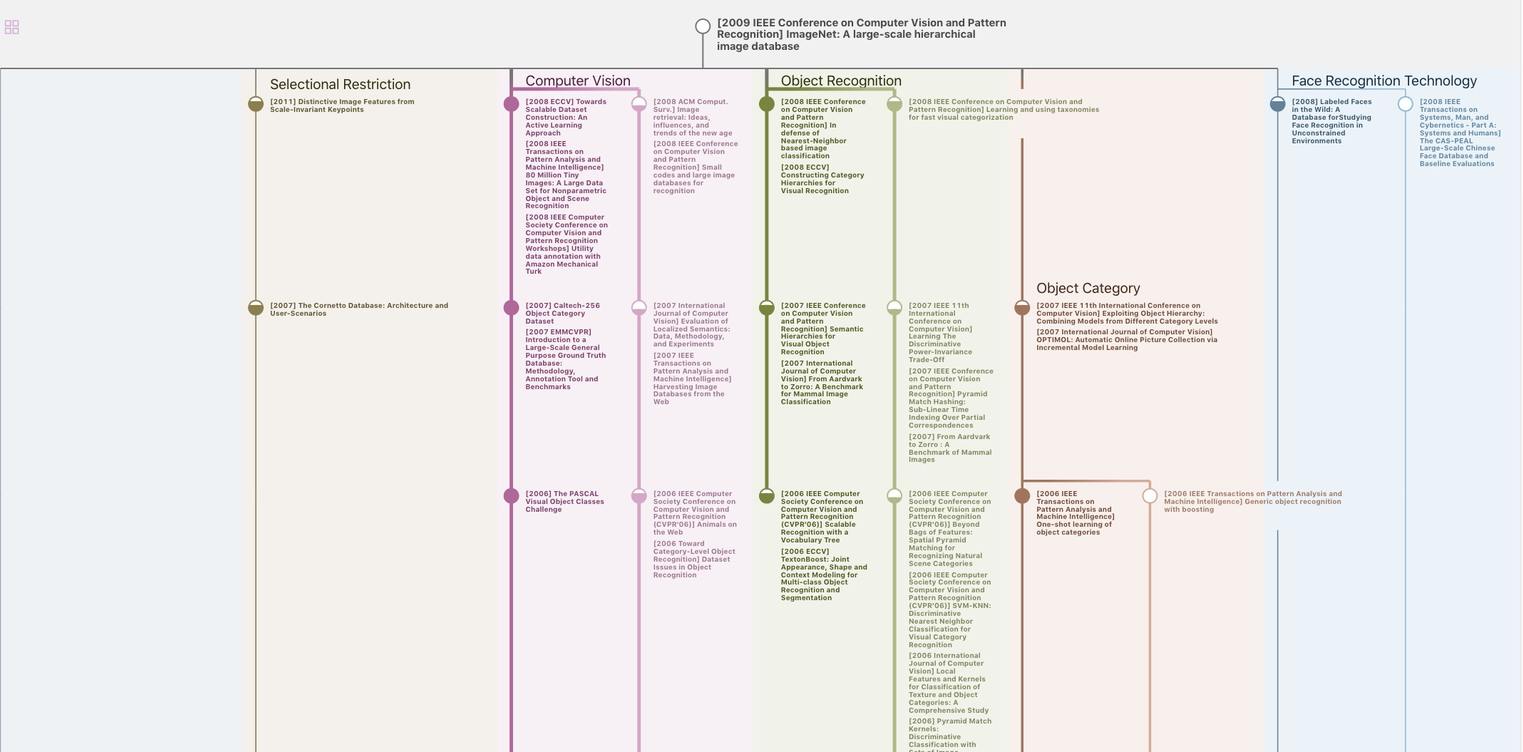
生成溯源树,研究论文发展脉络
Chat Paper
正在生成论文摘要