10.4 A 5.8Gb/s adaptive integrating duobinary-based DFE receiver for multi-drop memory interface
ISSCC(2015)
摘要
Emerging applications like cloud computing require high-speed low-latency access to high-volume data. In these applications, use of memory modules having multi-drop channels may be needed for time-efficient access to high-density memory data. A key design issue here is how to let interface transceivers not be affected by ISI and reflection noise generated by multi-drop channels having imperfect termination. The current-integrating decision-feedback equalizer (DFE) [1], which can cancel both high-frequency noise and post-cursor ISI simultaneously, has a limitation due to high gain-boosting and/or tap weight over-emphasis in equalizers to avoid eye closure caused by ISI-referred input pattern dependency. Duobinary signaling [2], which requires less boosting for equalizers by taking advantage of channel roll-off characteristic, is not effective in a multi-drop channel application because even a small timing or waveform variation due to high-frequency noise may cause degradation of the quality of duobinary signals. This work presents an integrating duobinary-based DFE receiver to avoid drawbacks described above and to increase the effective-data rate of multi-drop channels. A synergistic combination between the integrating equalizer and the duobinary signaling can provide advantages such as 1) lower gain-boosting for equalizers, 2) no need for precursor equalization, 3) ideally no input-pattern dependency during integration, 4) being more robust to high-frequency noise, 5) alleviated DFE critical timing, and 6) embedding DFE taps into duobinary circuits.
更多查看译文
关键词
integrated memory circuits,multidrop memory interface,bit rate 5.8 gbit/s,circuit noise,duobinary signaling,integrating equalizer,adaptive integrating duobinary-based dfe receiver,tap weight,current-integrating decision-feedback equalizer,channel roll-off characteristic,receivers,decision feedback equalisers,high gain-boosting,input-pattern dependency,decoding,noise,reflection
AI 理解论文
溯源树
样例
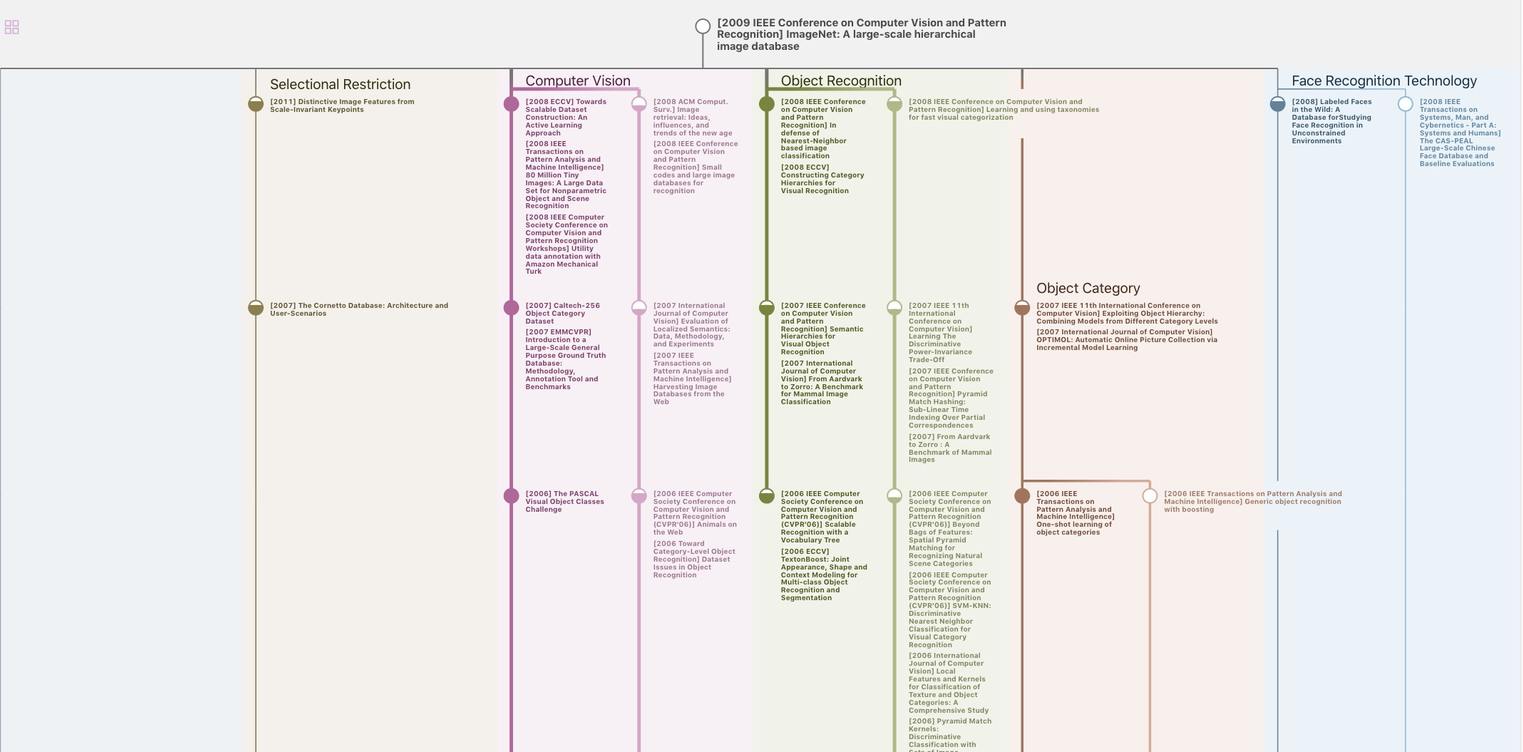
生成溯源树,研究论文发展脉络
Chat Paper
正在生成论文摘要