Structural least square twin support vector machine for classification
Applied Intelligence(2014)
摘要
The least square twin support vector machine (LS-TSVM) obtains two non-parallel hyperplanes by directly solving two systems of linear equations instead of two quadratic programming problems (QPPs) as in the conventional twin support vector machine (TSVM), which makes the computational speed of LS-TSVM faster than that of the TSVM. However, LS-TSVM ignores the structural information of data which may contain some vital prior domain knowledge for training a classifier. In this paper, we apply the prior structural information of data into the LS-TSVM to build a better classifier, called the structural least square twin support vector machine (S-LSTSVM). Since it incorporates the data distribution information into the model, S-LSTSVM has good generalization performance. Furthermore, S-LSTSVM costs less time by solving two systems of linear equations compared with other existing methods based on structural information. Experimental results on twelve benchmark datasets demonstrate that our S-LSTSVM performs well. Finally, we apply it into Alzheimer’s disease diagnosis to further demonstrate the advantage of our algorithm.
更多查看译文
关键词
TSVM,Least square TSVM,Structural information,Structural least square TSVM
AI 理解论文
溯源树
样例
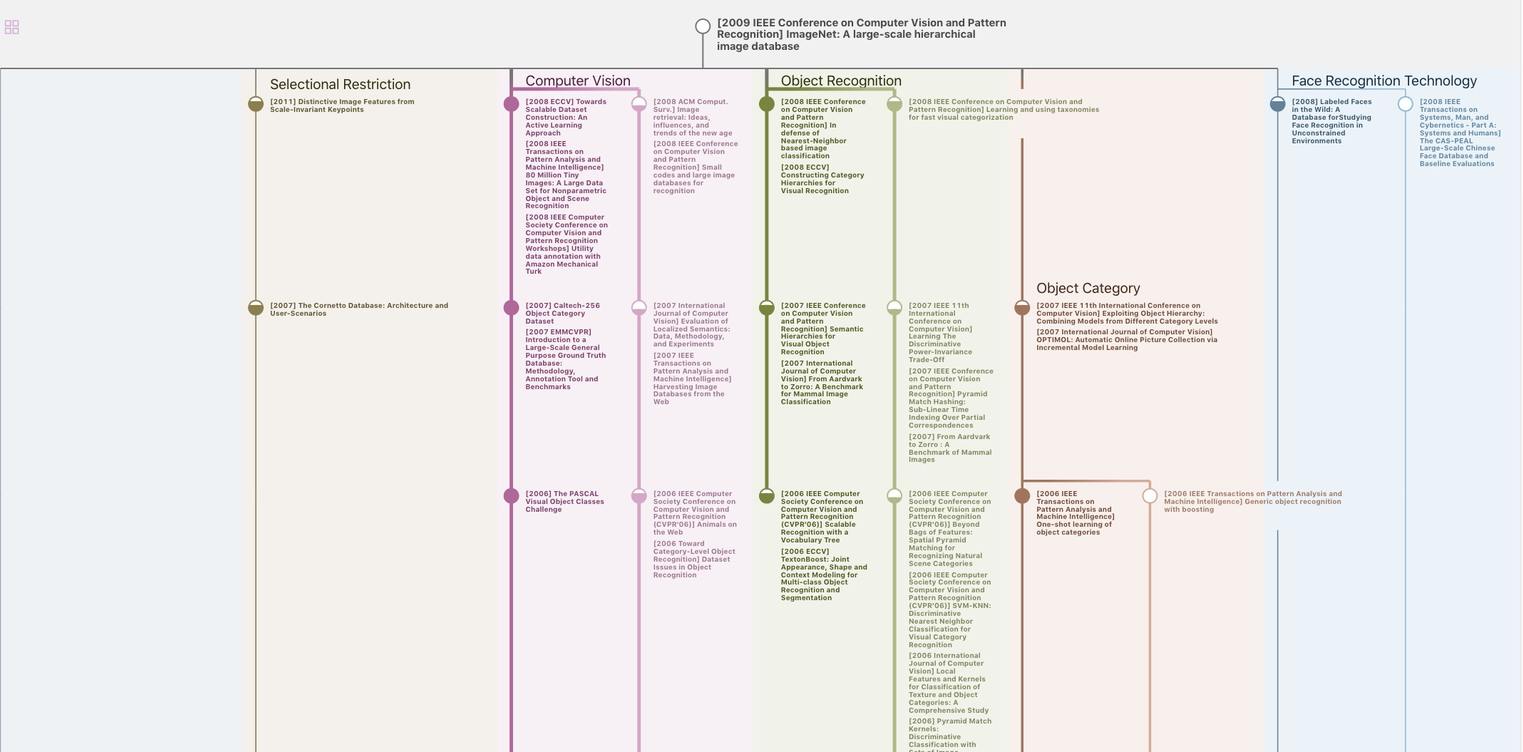
生成溯源树,研究论文发展脉络
Chat Paper
正在生成论文摘要