A method for attribute reduction using rough set theory and improved particle swarm optimization
ICIC Express Letters, Part B: Applications(2011)
摘要
Attribute reduction is one of the important topics in data mining which is aimed at eliminating irrelevant and redundant attributes, thereby finding minimal attribute subset(s) in a problem domain. Particle swarm optimization (PSO) is an at- tractive technique for attribute reduction. However, this method still cannot always find optimal subsets. In this article, we propose a framework to hybridize the improved particle swarm optimization (IPSO) with rough set theory (RST) in an attempt to combat this shortcoming, generating one or more optimal reductions. Two concepts - support degree of conditional attributes on decision attribute, and relative attribute importance degree are introduced in order to obtain relative core and treat it as initial population in IPSO to accelerate convergence. Punishing function is employed in fitness function to ensure reduction(s) have fewer attributes and better search efficiency. Experimental results state competitive performance of the suggested algorithm. © 2011 ICIC International.
更多查看译文
关键词
attribute reduction,particle swarm optimization (pso),rough set
AI 理解论文
溯源树
样例
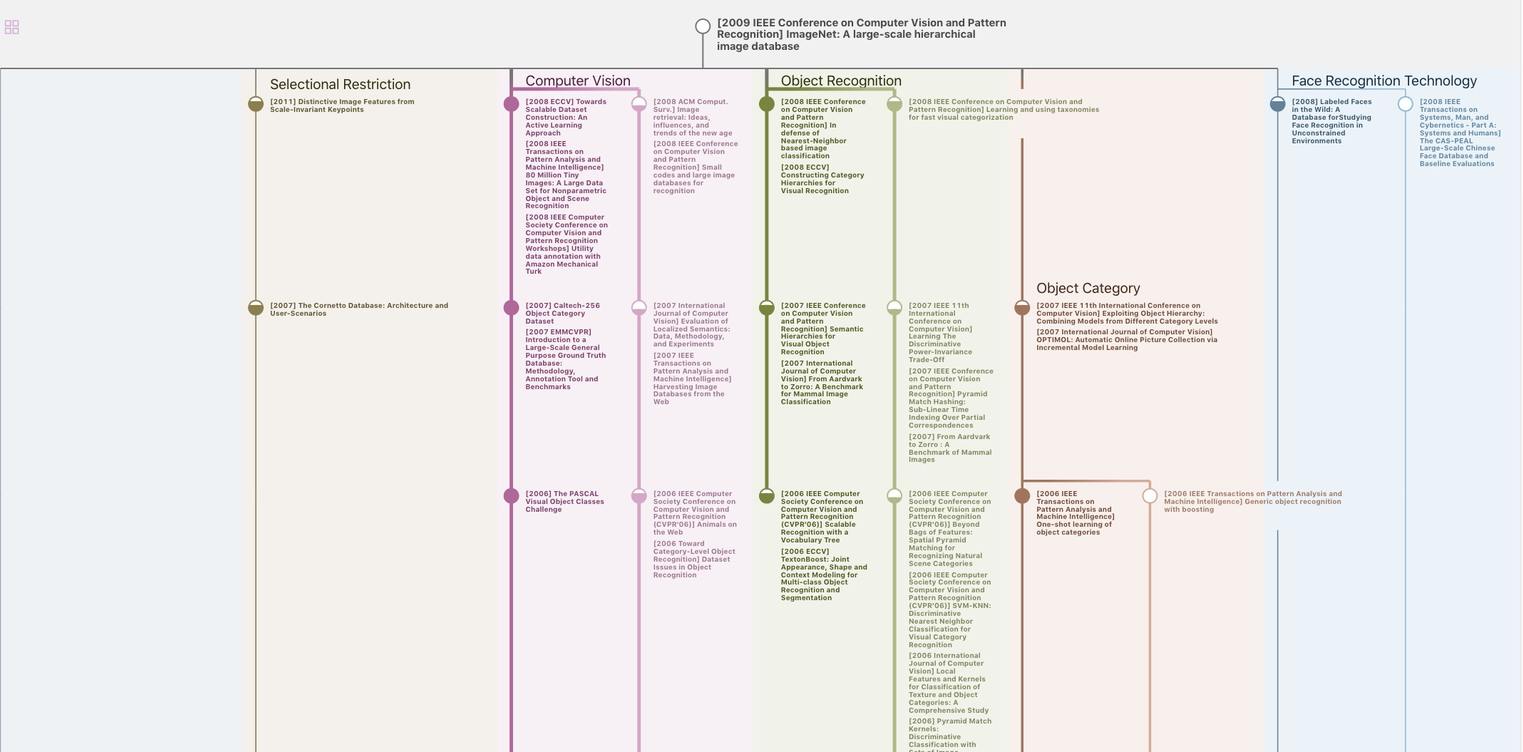
生成溯源树,研究论文发展脉络
Chat Paper
正在生成论文摘要