A statistical approach to instance-level schema matching
Journal of Information and Computational Science(2009)
摘要
Information integration refers to the problem of merging, coalescing and transforming autonomous heterogeneous data sources into a single global homogeneous database and providing a unified view of these data for future query processing purposes. One of the fundamental operations in the integration process is schema matching, which takes two schemas as input and produces a mapping between the attributes of the two schemas that correspond semantically to each other [4, 6]. Matching techniques can be grouped into two broad categories: Schema-level matching and instance-level matching [11]. In schema-level matching, we consider only the properties of schema elements, such as names, descriptions, data types, constraints and structures [2]. For each match candidate pair of attributes, the degree of similarity is estimated by a normalized numeric value between 0 and 1. On the other hand, instance-level matching employs information available in the data contents of each table to determine the relationship between any two attributes. In this paper, we propose a statistical model to compare the likeliness of two lists of values under two attributes from separate databases, in order to derive the similarity ratio of the two attributes. Our framework provides efficient procedures to compute the degree ratio using statistical coefficients for both categorical and numeric attributes. © 2009 Binary Information Press.
更多查看译文
关键词
Data integration,Instance level,Schema matching,Similarity matching,Statistical methods
AI 理解论文
溯源树
样例
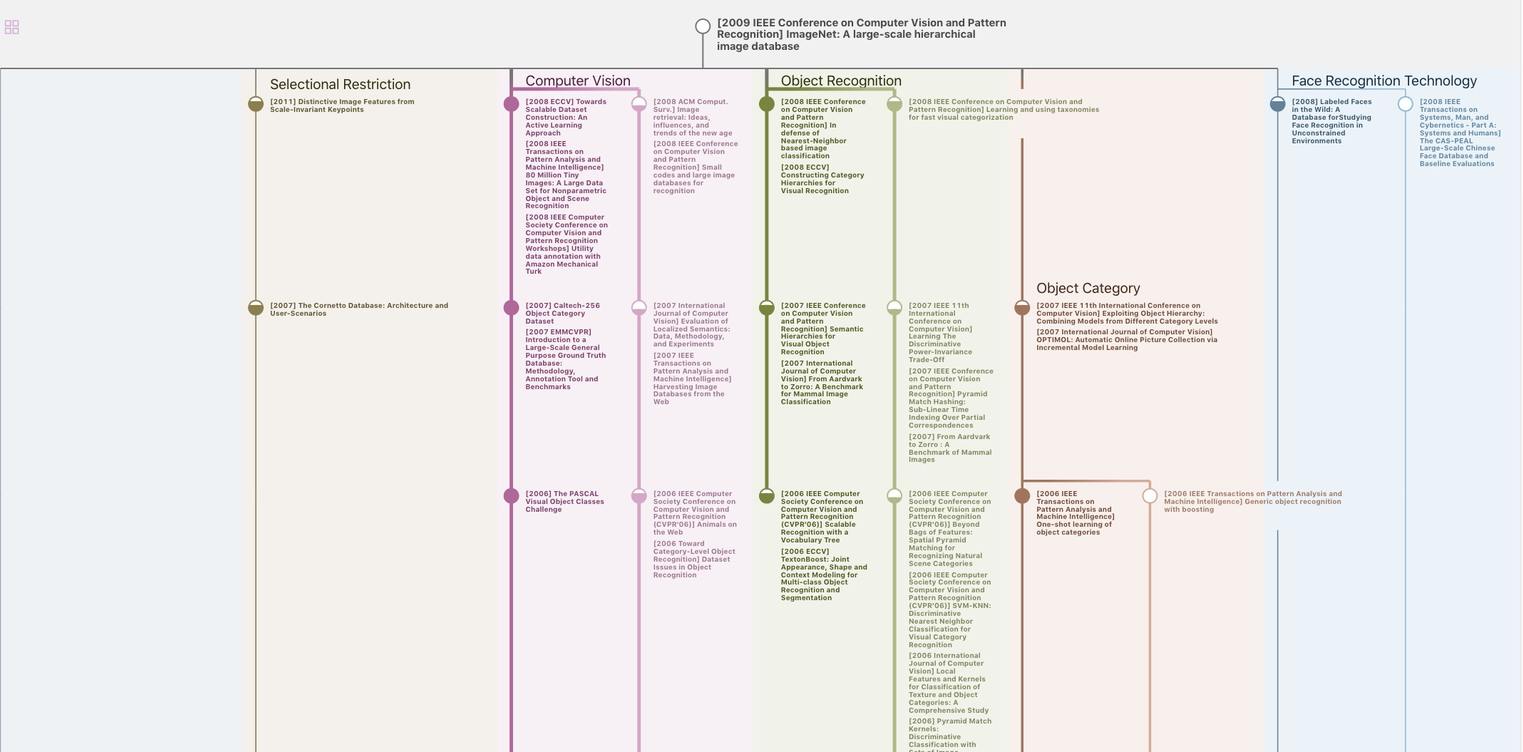
生成溯源树,研究论文发展脉络
Chat Paper
正在生成论文摘要