Monte Carlo Tracking Method With Threshold Constraint
PROCEEDINGS OF THE 2009 2ND INTERNATIONAL CONGRESS ON IMAGE AND SIGNAL PROCESSING, VOLS 1-9(2009)
摘要
Monte Carlo Method has been proven very successful for non-linear and non-Gaussian estimation problems. However, a Monte Carlo filter usually has a lot of samples, which increase the computational load greatly. This paper presents threshold constraint into Monte Carlo. The target model is defined by the color information of the tracked object. Use the Bhattacharyya coefficient to get the similarity measure between each sample and the model. Then, get the descending order of the similarity measures and choose the median as the threshold. The samples whose similarity measure value is greater than the threshold are chosen for transferring and resampling. Experimental results show that the proposed method perform better than Monte Carlo method without threshold constraint and simultaneously lower the computational burden. When choose 200 samples, the average computational load is 54 milliseconds for proposed method and is approximate 164 milliseconds for traditional methods for target of 60*30 pixels.
更多查看译文
关键词
Monte Carlo Method,Bayesian theory,target tracking,Bhattacharyya coefficient,Color filtering
AI 理解论文
溯源树
样例
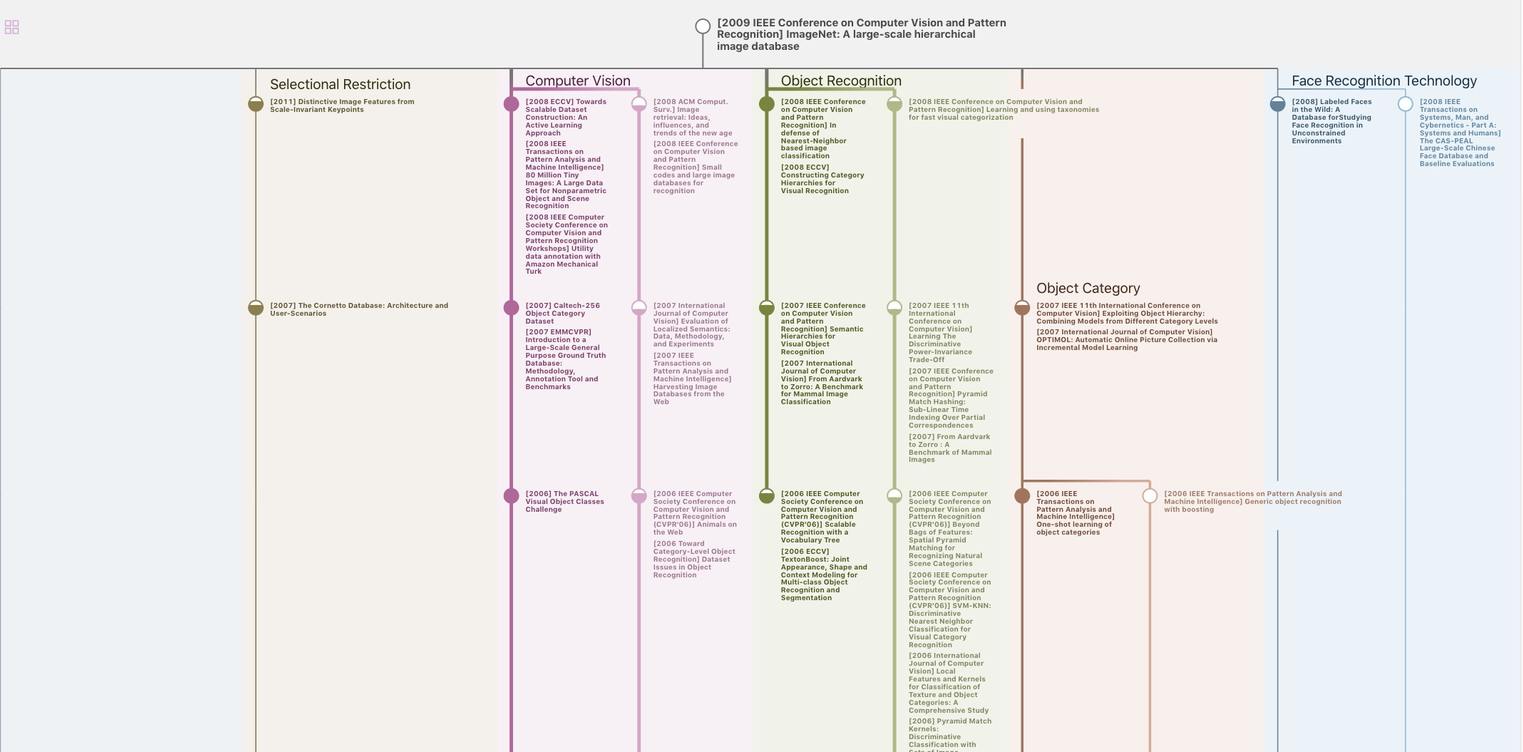
生成溯源树,研究论文发展脉络
Chat Paper
正在生成论文摘要