Using preliminary and secondary classification to learn imbalanced data
Journal of Computational Information Systems(2006)
摘要
Learning from imbalanced data sets presents a new challenge to machine learning community, as traditional algorithms are biased to majority classes and produce poor detection rate of minority classes. To overcome the shortcomings of re-sampling methods, research focuses on a new approach which uses preliminary and secondary classification to improve the classification performance of the minority class. Firstly, the approach retains the useful information of the majority class through the preliminary classification. Secondly, it improves the classification performance of the minority class effectively through the secondary classification. Experimental results show that the new approach not only improves the classification performance of the minority class more effectively, but also is superior in the classification performance of the majority class and the whole data set comparing with other methods.
更多查看译文
关键词
Imbalanced data sets,Over-sampling,Preliminary classification,Secondary classification,Under-sampling
AI 理解论文
溯源树
样例
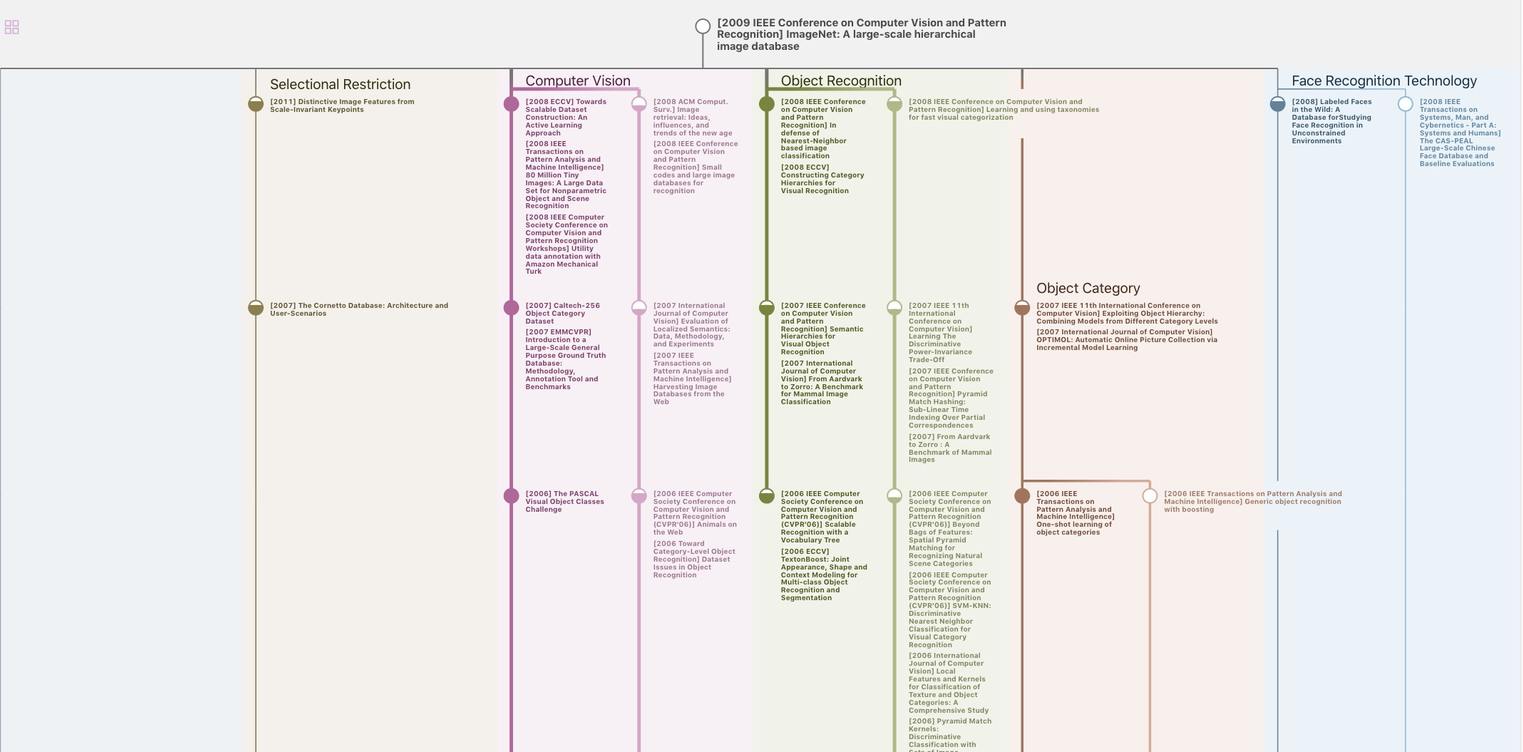
生成溯源树,研究论文发展脉络
Chat Paper
正在生成论文摘要