The lasso under poisson-like heteroscedasticity
STATISTICA SINICA(2013)
摘要
The performance of the Lasso is well understood under the assumptions of the standard sparse linear model with homoscedastic noise. However, in several applications, the standard model does not describe the important features of the data. This paper examines how the Lasso performs on a non-standard model that is motivated by medical imaging applications. In these applications, the variance of the noise scales linearly with the expectation of the observation. Like all heteroscedastic models, the noise terms in this Poisson-like model are not independent of the design matrix. Under a sparse Poisson-like model for the high-dimension regime that allows the number of predictors (p) >> sample size (n), we give necessary and sufficient conditions for the sign consistency of the Lasso estimate. Simulations reveal that the Lasso performs equally well in terms of model selection performance on both Poisson-like data and homoscedastic data (with properly scaled noise variance), across a range of parameterizations.
更多查看译文
关键词
Heteroscedasticity,Lasso,Poisson-like model,sign consistency
AI 理解论文
溯源树
样例
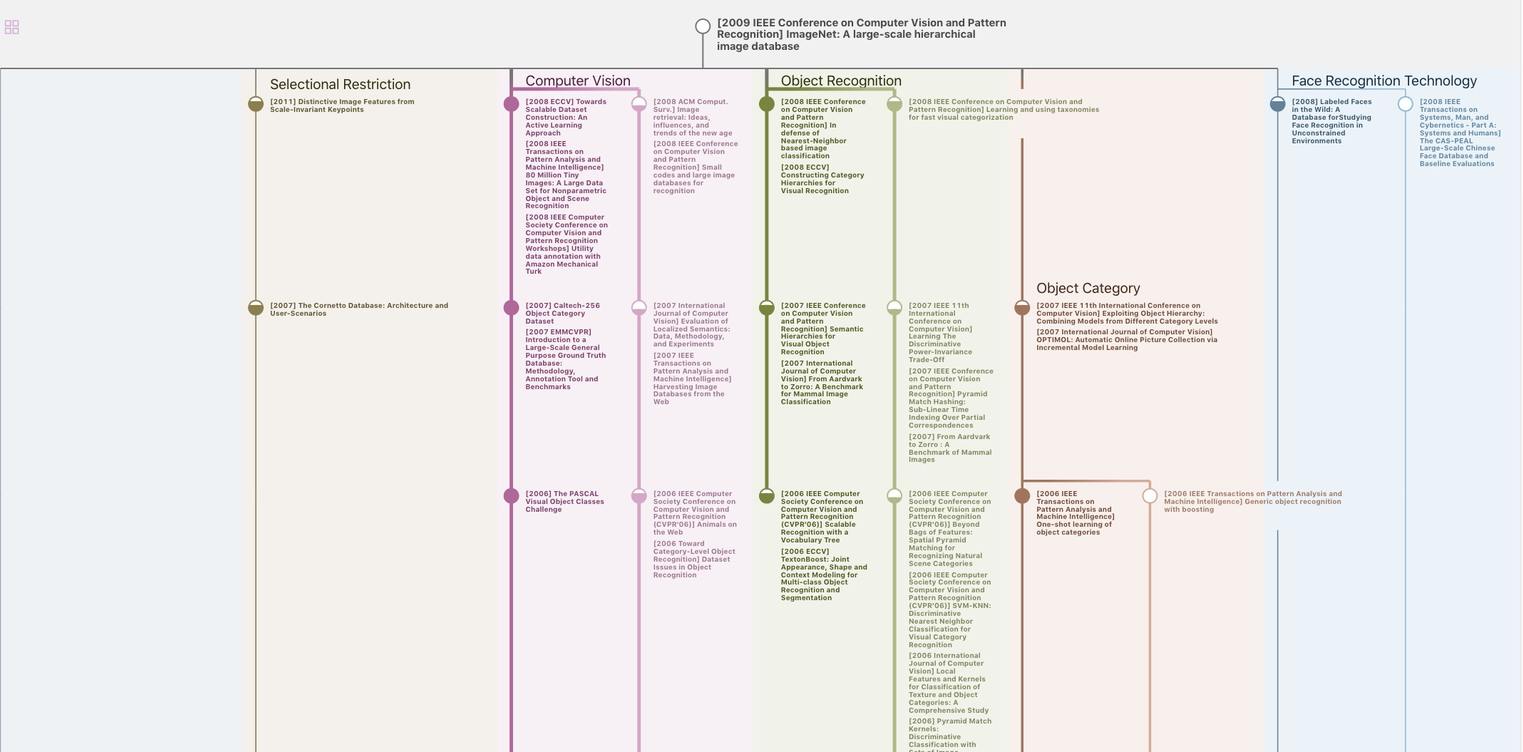
生成溯源树,研究论文发展脉络
Chat Paper
正在生成论文摘要