A lightweight decentralized algorithm for jointly sparse optimization
Control Conference(2013)
摘要
This paper develops a lightweight decentralized algorithm to solve the convex jointly sparse optimization problem, as known as the group lasso. In a networked multi-agent system, each agent takes linear measurements from its signal, and all signals share the same sparsity pattern. In decentralized jointly sparse optimization, agents collaborate to recover their signals by taking advantage of the same sparsity pattern, but they are allowed to have limited information exchange with their one-hop neighbors. We propose to use the block coordinate descent algorithm to solve the convex jointly sparse optimization problem in a centralized manner, and adopt an inexact average consensus technique for its decentralized implementation. The proposed decentralized algorithm is lightweight; each agent neither exchanges its measurement matrix and measurement vector nor shares its current estimate of its signal with its one-hop neighbors. Simulation results demonstrate the effectiveness of the proposed algorithm, as well as its empirical global convergence to the centralized optimal solution.
更多查看译文
关键词
measurement matrix,block coordinate descent,decentralized optimization,lightweight decentralized algorithm,decentralized implementation,networked multi-agent system,jointly sparse optimization,matrix algebra,one-hop neighbors,convex programming,sparsity pattern,multi-agent systems,convergence,global convergence,networked multiagent system,compressed sensing,decentralized jointly sparse optimization,centralized manner,average consensus technique,linear measurements,group lasso,convex jointly sparse optimization problem,information exchange,centralized optimal solution,block coordinate descent algorithm,vectors,measurement vector,multi agent systems,algorithm design and analysis,minimization,optimization
AI 理解论文
溯源树
样例
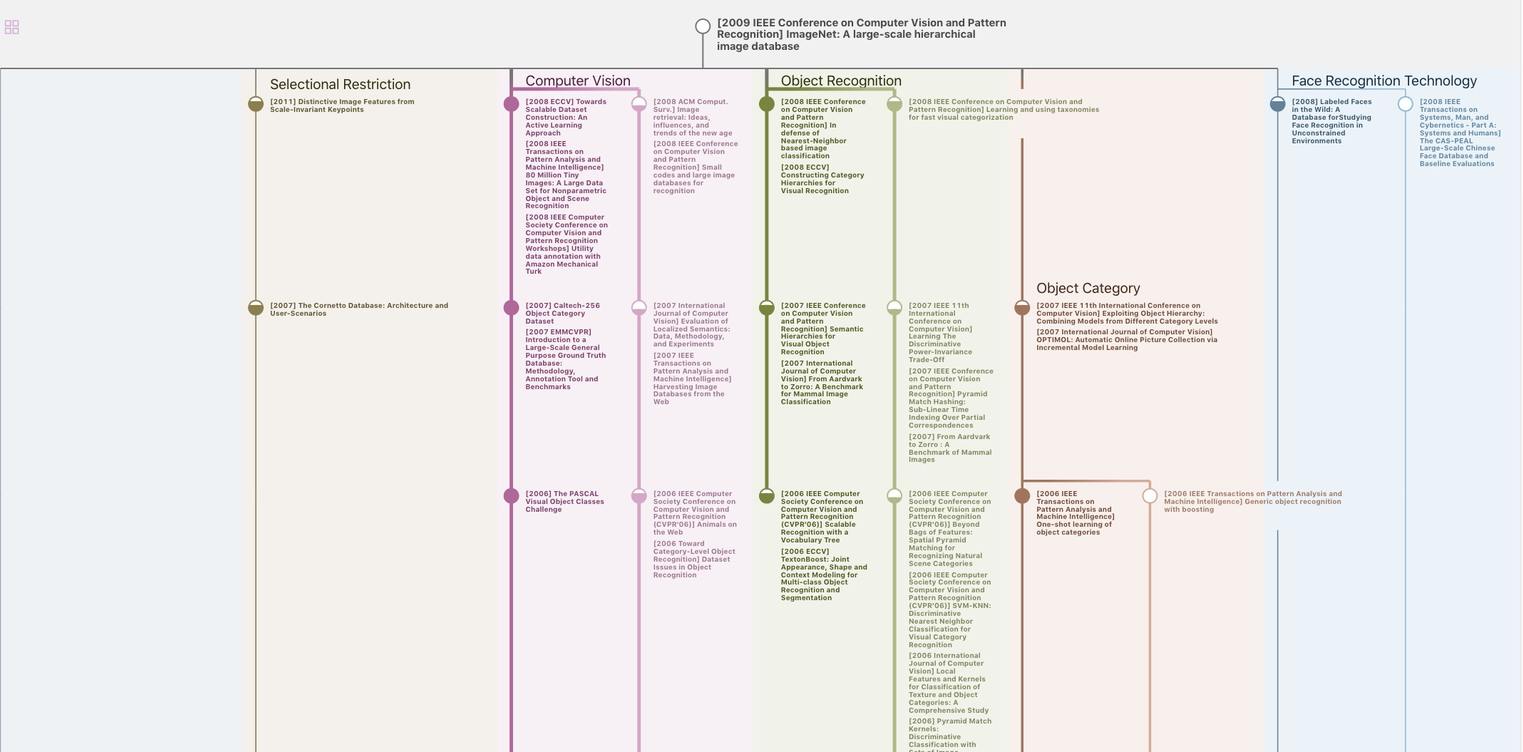
生成溯源树,研究论文发展脉络
Chat Paper
正在生成论文摘要