An approach based on multi-feature wavelet and elm algorithm for forecasting outlier occurrence in chinese stock market
Journal of Theoretical and Applied Information Technology(2013)
摘要
The prediction of outliers plays an important role in stock arbitrage and risk avoiding. While most of researches focused on detecting outliers and removi ng them to forecast time series data, few focused o n forecasting the occurrence of outliers. The main go al of this work is to forecast outlier occurrence i n Chinese stock market. Firstly, we detect abnormal p oints of two market indexes and six individual stoc ks based on multi-feature wavelet method. Compared wit h s ngle feature wavelet method, multi-feature wavelet method was more reliable, since it captures more information of the market and avoids “masking effect”. Moreover, according to the detected result s, we construct an outlier forecasting model for th e two market indexes and six individual stocks based on m ulti-feature extreme learning machine (ELM) algorithm. Finally, we find out the optimal number of hidden nodes to forecast when the mean of forecasting accuracy was the highest which approxim ately equals 98.255% and the variance of forecastin g accuracy was only 0.000193.
更多查看译文
关键词
Extreme learning machine (ELM),Multi-feature wavelet,Outlier detection,Outlier forecasting
AI 理解论文
溯源树
样例
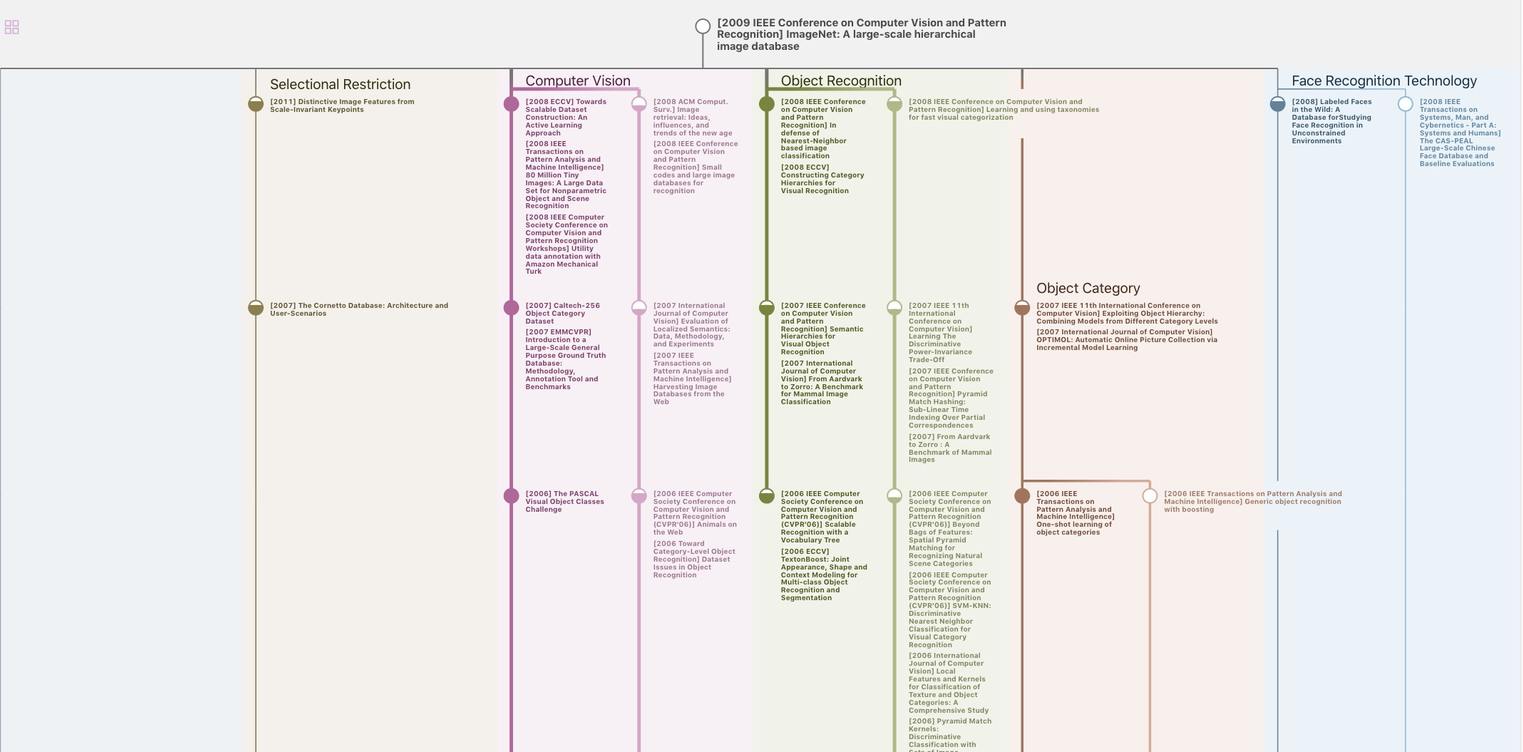
生成溯源树,研究论文发展脉络
Chat Paper
正在生成论文摘要