2D-SPP: A two-dimensional extension of sparsity preserving projections
Journal of Information and Computational Science(2012)
摘要
Recently SPP was proposed for Dimensionality Reduction (DR), which is a new unsupervised DR method. Although SPP belongs to global methods in nature, it owns some local properties due to the sparse representation procedure. As far as matrix data, such as images, are concerned, they are often vectorized for SPP algorithm to find the intrinsic manifold structure. While the dimension of matrix data is usually very high, SPP cannot be implemented because of the singularity of matrix. In this paper, we propose a method called Two-dimensional Sparsity Preserving Projections (2D-SPP) for image recognition, which is based directly on 2D image matrices rather than 1D vectors as conventional SPP does. The 2D methods preserving the spatial structure information of the original image matrices, and the manifold methods preserving the local structure of the samples distribution. To evaluate the performance of 2D-SPP, several experiments are conducted on the ORL face database, the Yale face database and the AR face database show the effectiveness of the proposed method. 1548-7741/Copyright © 2012 Binary Information Press.
更多查看译文
关键词
Dimensionality reduction,Sparsity preserving projections,Two-dimensional projection
AI 理解论文
溯源树
样例
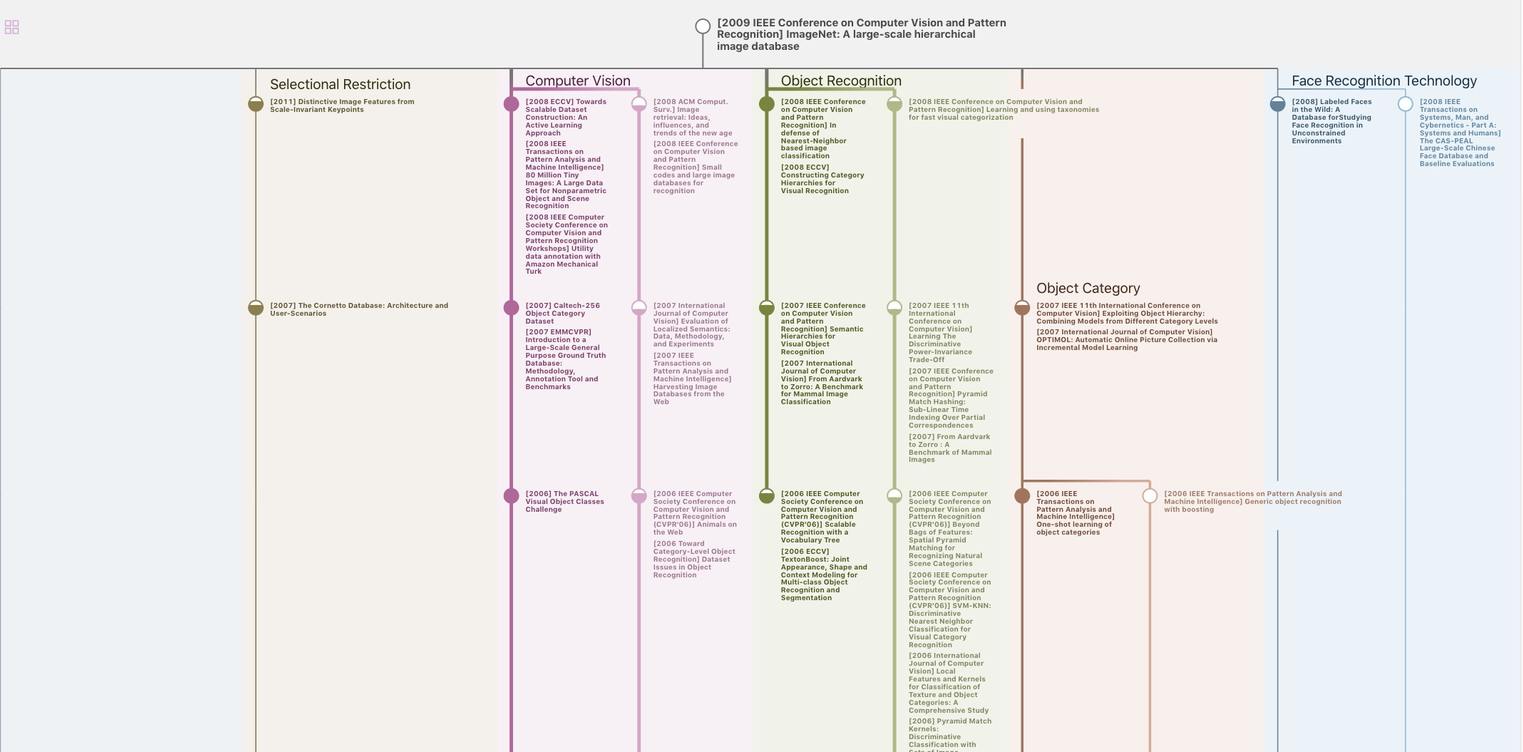
生成溯源树,研究论文发展脉络
Chat Paper
正在生成论文摘要