An Intelligent Fault Diagnosis Method Of Rolling Bearings Based On Regularized Kernel Marginal Fisher Analysis
25TH INTERNATIONAL CONGRESS ON CONDITION MONITORING AND DIAGNOSTIC ENGINEERING (COMADEM 2012)(2012)
摘要
Generally, the vibration signals of fault bearings are non-stationary and highly nonlinear under complicated operating conditions. Thus, it's a big challenge to extract optimal features for improving classification and simultaneously decreasing feature dimension. Kernel Marginal Fisher analysis (KMFA) is a novel supervised manifold learning algorithm for feature extraction and dimensionality reduction. In order to avoid the small sample size problem in KMFA, we propose regularized KMFA (RKMFA). A simple and efficient intelligent fault diagnosis method based on RKMFA is put forward and applied to fault recognition of rolling bearings. So as to directly excavate nonlinear features from the original high-dimensional vibration signals, RKMFA constructs two graphs describing the intra-class compactness and the inter-class separability, by combining traditional manifold learning algorithm with fisher criteria. Therefore, the optimal low-dimensional features are obtained for better classification and finally fed into the simplest K-nearest neighbor (KNN) classifier to recognize different fault categories of bearings. The experimental results demonstrate that the proposed approach improves the fault classification performance and outperforms the other conventional approaches.
更多查看译文
关键词
manifold learning,feature extraction,operant conditioning,k nearest neighbor
AI 理解论文
溯源树
样例
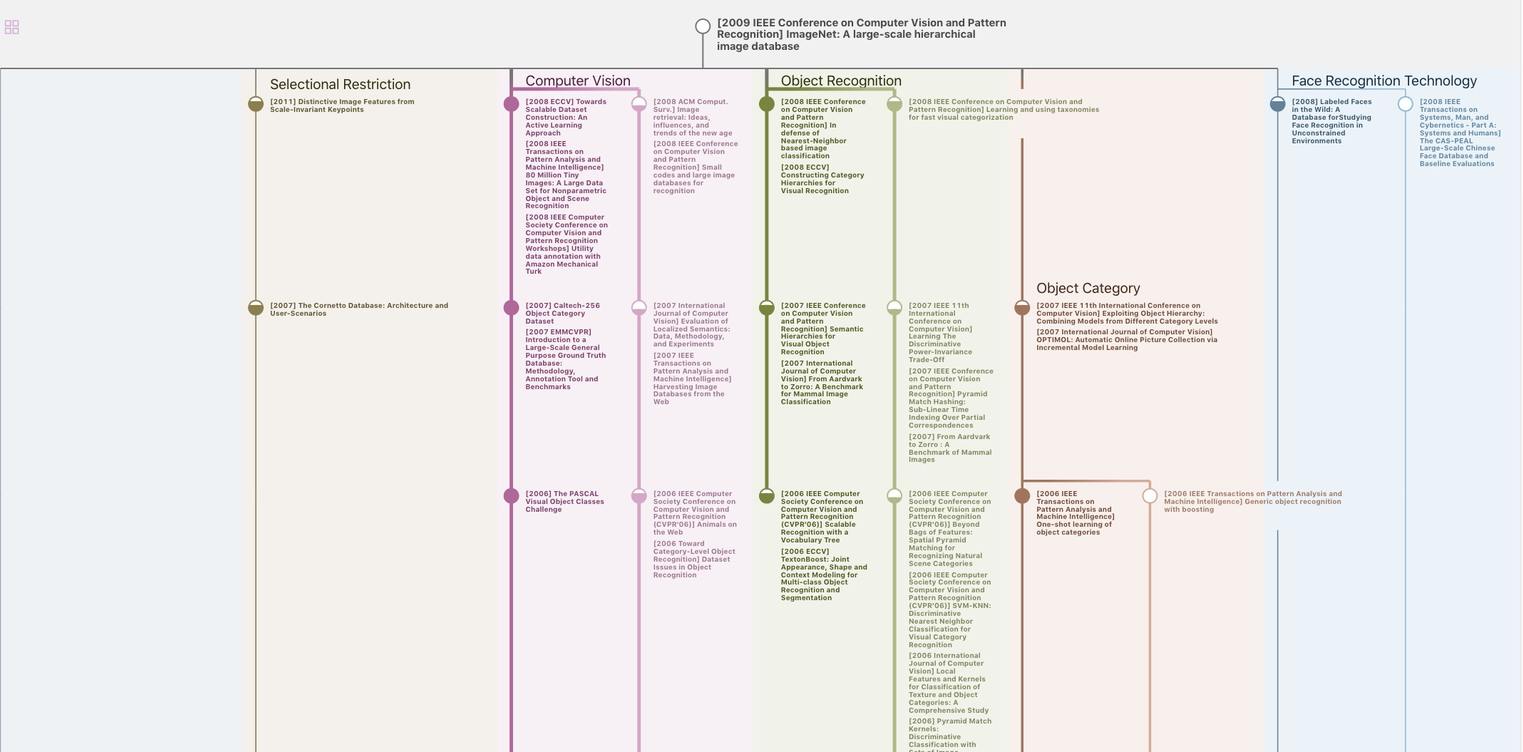
生成溯源树,研究论文发展脉络
Chat Paper
正在生成论文摘要