Hierarchical proportional redistribution principle for uncertainty reduction and BBA approximation
Proceedings of the World Congress on Intelligent Control and Automation (WCICA)(2012)
摘要
Dempster-Shafer evidence theory is very important in the fields of information fusion and decision making. However, it always brings high computational cost when the frames of discernments to deal with become large. To reduce the heavy computational load involved in many rules of combinations, the approximation of a general belief function is needed. In this paper we present a new general principle for uncertainty reduction based on hierarchical proportional redistribution (HPR) method which allows to approximate any general basic belief assignment (bba) at a given level of non-specificity, up to the ultimate level 1 corresponding to a Bayesian bba. The level of non-specificity can be adjusted by the users. Some experiments are provided to illustrate our proposed HPR method. © 2012 IEEE.
更多查看译文
关键词
belief approximation,belief functions,evidence combination,hierarchical proportional redistribution (hpr),bayesian methods,function approximation,cognition,uncertainty reduction,case based reasoning,uncertainty
AI 理解论文
溯源树
样例
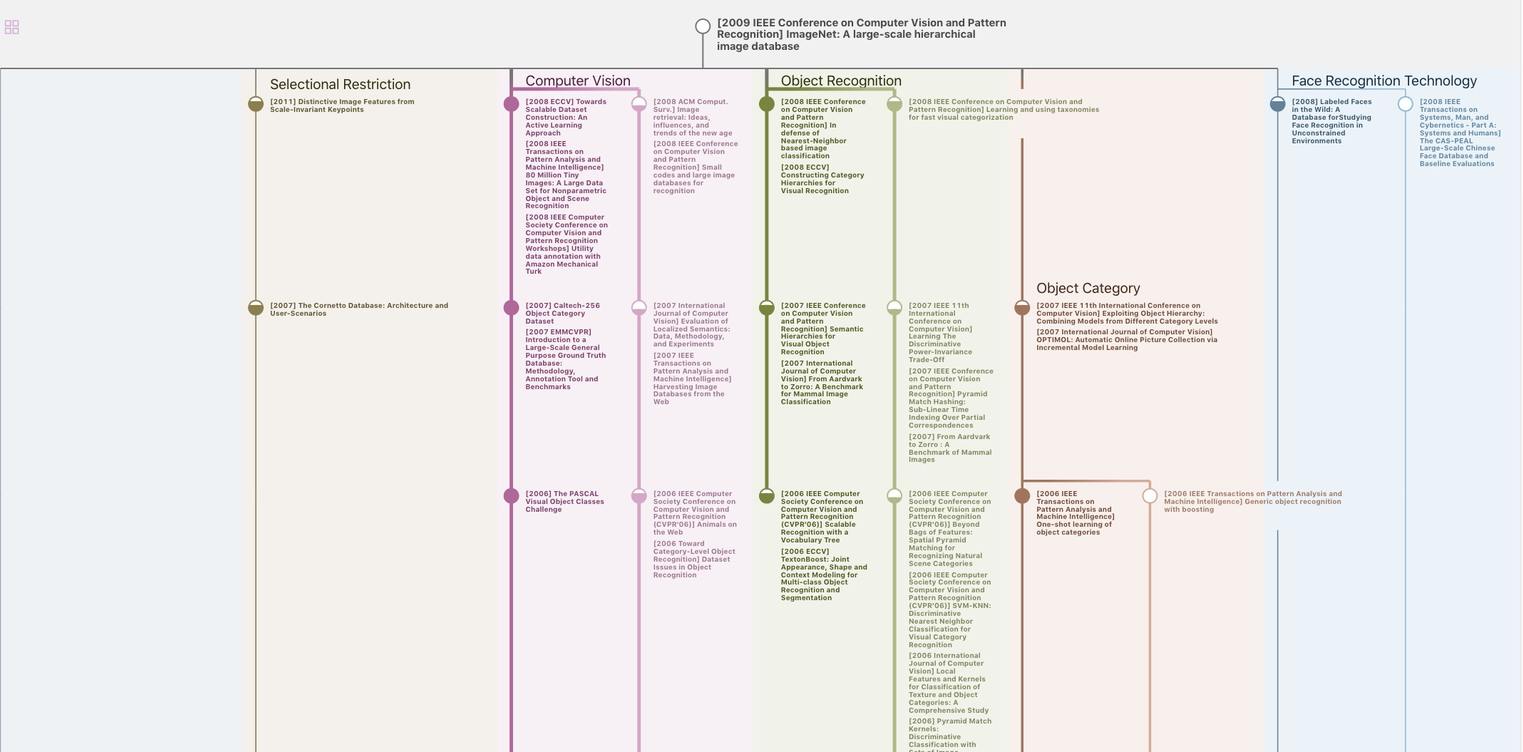
生成溯源树,研究论文发展脉络
Chat Paper
正在生成论文摘要