A New Graph Based Active Learning Method
2012 INTERNATIONAL WORKSHOP ON INFORMATION AND ELECTRONICS ENGINEERING(2012)
摘要
Both semi-supervised learning (SSL) and active learning try to use unlabeled data to train high precision classifier with limited number of labeled data. It's a natural way to think about combine them together to get a good classifier. In this paper, we give a new selection criterion for a graph based semi-supervised learner. Contribution of our work is that based on local & global consistency (LGC) SSL method we create a new framework of active learning. Firstly, we do active learning by selecting representative data and these data uniformly distribute over the manifold cloud with the largest local density and have the least chance to be error labeled. Secondly, we do greed confidence label propagation inference under LGC-F function criterion in the second active learning process. Thirdly, our work can be easily extended to multi-class problem using LGC-F function. Compared with Zhu's work, our algorithm starts from representative data in local area and make no assumption about the distribution of original dataset. The proposed active learning method only starts from one basic assumption that data close to each other should have the same label. Our method is more robust and more general compared with Zhu's work. Experiment results on two moon toy data, digit recognition, and face detection dataset prove that the efficiency of our new active leaning method. (C) 2011 Published by Elsevier Ltd. Selection and/or peer-review under responsibility of Harbin University of Science and Technology
更多查看译文
关键词
Semi-supervised Learning,Active Learning,K-Means Clustering
AI 理解论文
溯源树
样例
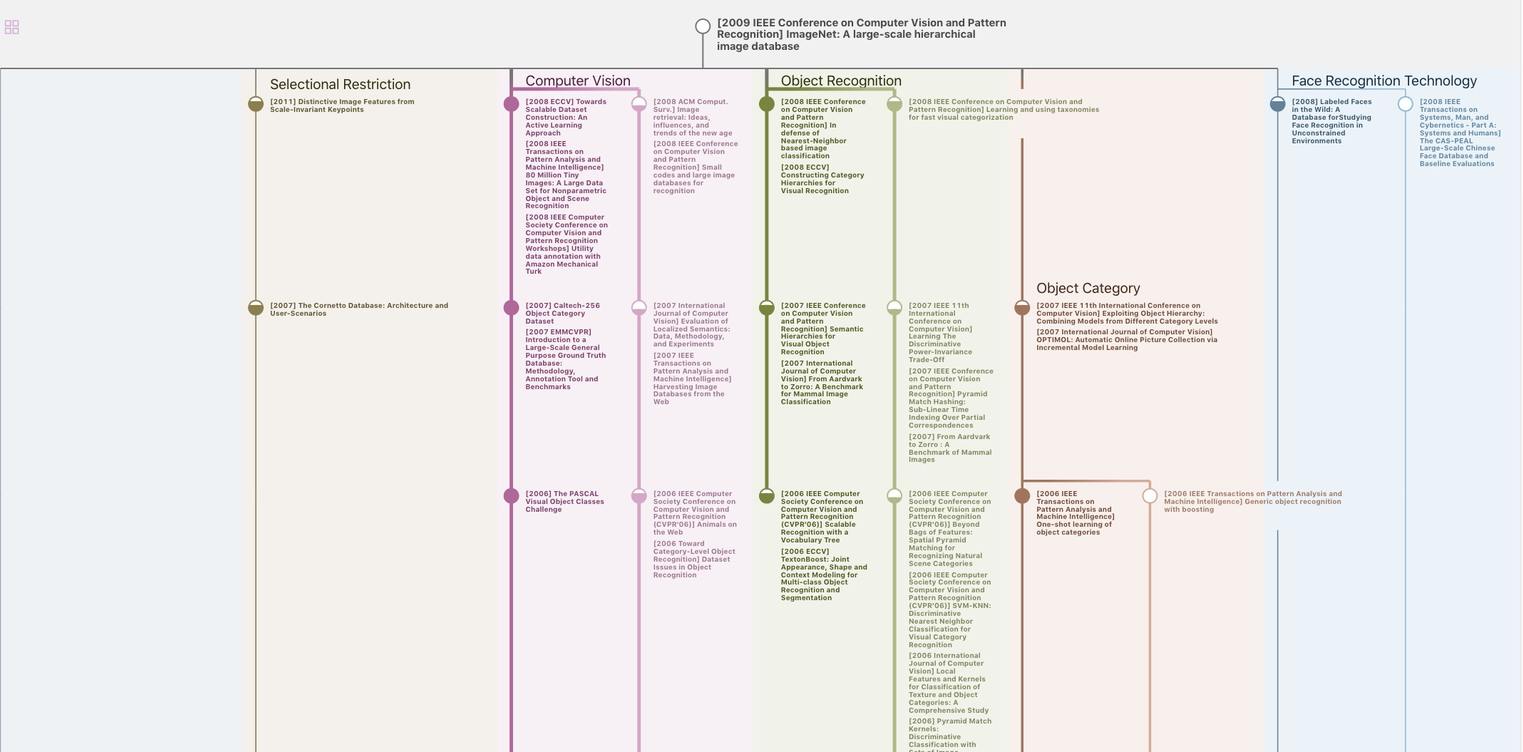
生成溯源树,研究论文发展脉络
Chat Paper
正在生成论文摘要