Fault diagnosis based on signed directed graph and support vector machine
Proceedings of SPIE(2012)
摘要
Support Vector Machine (SVM) based on Structural Risk Minimization (SRM) of Statistical Learning Theory has excellent performance in fault diagnosis. However, its training speed and diagnosis speed are relatively slow. Signed Directed Graph (SDG) based on deep knowledge model has better completeness that is knowledge representation ability. However, much quantitative information is not utilized in qualitative SDG model which often produces a false solution. In order to speed up the training and diagnosis of SVM and improve the diagnostic resolution of SDG, SDG and SVM are combined in this paper. Training samples' dimension of SVM is reduced to improve training speed and diagnosis speed by the consistent path of SDG; the resolution of SDG is improved by good classification performance of SVM. The Matlab simulation by Tennessee-Eastman Process (TEP) simulation system demonstrates the feasibility of the fault diagnosis algorithm proposed in this paper.
更多查看译文
关键词
Support Vector Machine,Signed Directed Graph,Fault Diagnosis,Tennessee-Eastman Process
AI 理解论文
溯源树
样例
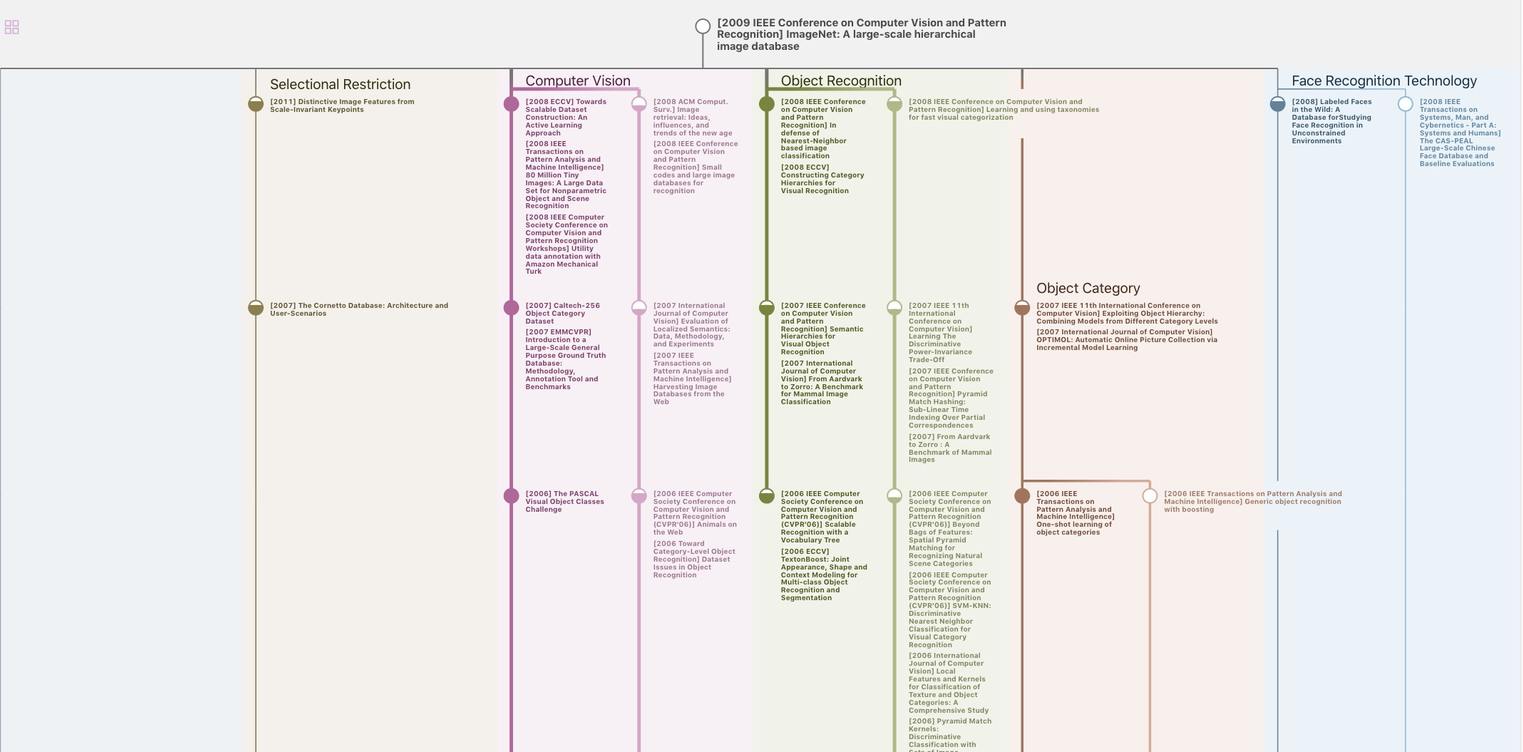
生成溯源树,研究论文发展脉络
Chat Paper
正在生成论文摘要