Vector Diffusion Maps and the Connection Laplacian.
COMMUNICATIONS ON PURE AND APPLIED MATHEMATICS(2012)
摘要
We introduce vector diffusion maps (VDM), a new mathematical framework for organizing and analyzing massive high-dimensional data sets, images, and shapes. VDMis a mathematical and algorithmic generalization of diffusion maps and other nonlinear dimensionality reduction methods, such as LLE, ISOMAP, and Laplacian eigenmaps. While existing methods are either directly or indirectly related to the heat kernel for functions over the data, VDM is based on the heat kernel for vector fields. VDM provides tools for organizing complex data sets, embedding them in a low-dimensional space, and interpolating and regressing vector fields over the data. In particular, it equips the data with a metric, which we refer to as the vector diffusion distance. In the manifold learning setup, where the data set is distributed on a low-dimensional manifold M-d embedded in R-p, we prove the relation between VDM and the connection Laplacian operator for vector fields over the manifold. (c) 2012 Wiley Periodicals, Inc.
更多查看译文
关键词
bioinformatics,biomedical research,high dimensional data,complex data,heat kernel,manifold learning,vector field
AI 理解论文
溯源树
样例
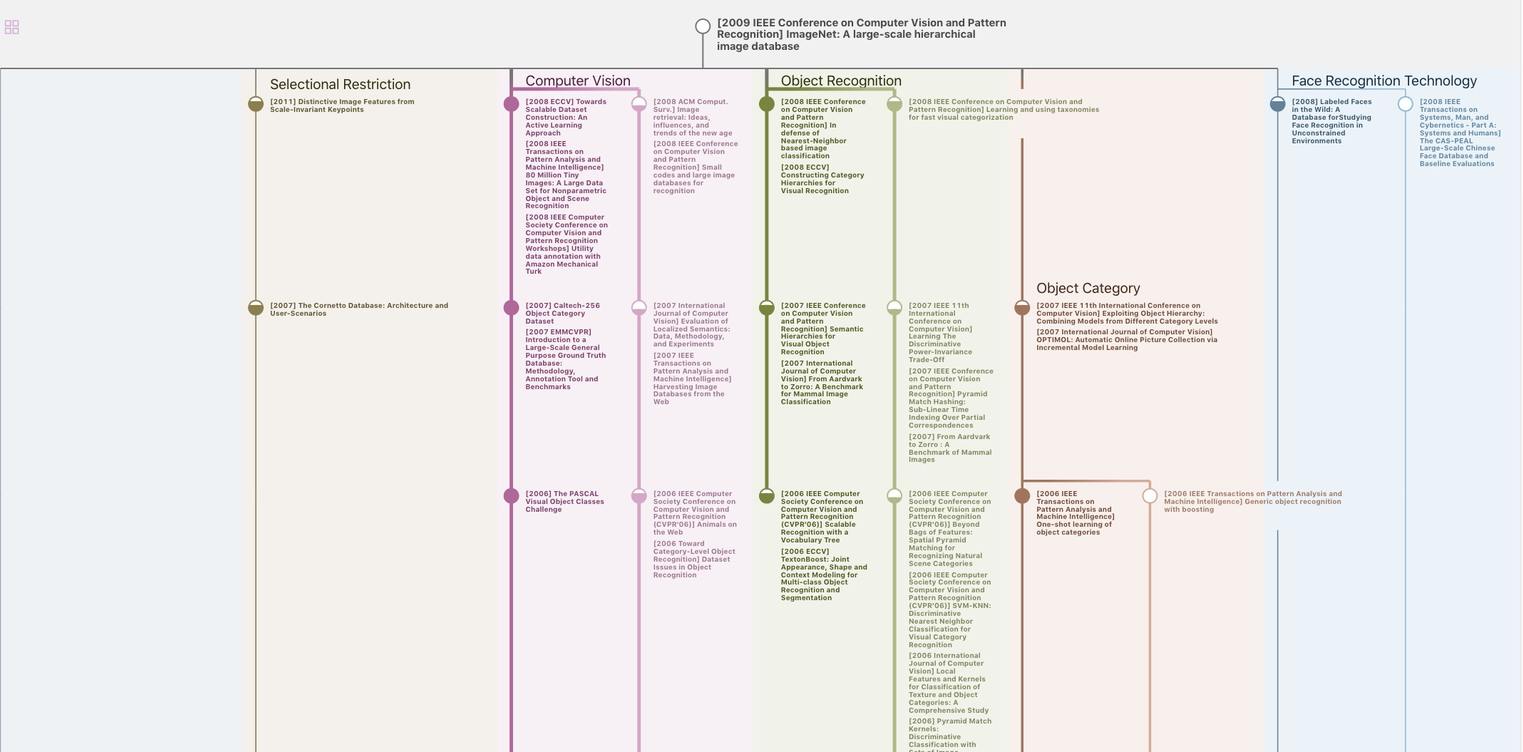
生成溯源树,研究论文发展脉络
Chat Paper
正在生成论文摘要