Classification of Sparse Time Series via Supervised Matrix Factorization.
AAAI(2012)
摘要
Data sparsity is an emerging real-world problem observed in a various domains ranging from sensor networks to medical diagnosis. Consecutively, numerous machine learning methods were modeled to treat missing values. Nevertheless, sparsity, defined as missing segments, has not been thoroughly investigated in the context of time series classification. We propose a novel principle for classifying time series, which in contrast to existing approaches, avoids reconstructing the missing segments in time series and operates solely on the observed ones. Based on the proposed principle, we develop a method that prevents adding noise that incurs during the reconstruction of the original time series. Ourmethod adapts supervised matrix factorization by projecting time series in a latent space through stochasticlearning. Furthermore the projected data is built in a supervised fashion via a logistic regression. Abundant experiments on a large collection of 37 data sets demonstrate the superiority of our method, which in the majority of cases outperforms a set of baselines that do not follow our proposed principle.
更多查看译文
关键词
time series,matrix factorization,sparsity,logistic regression
AI 理解论文
溯源树
样例
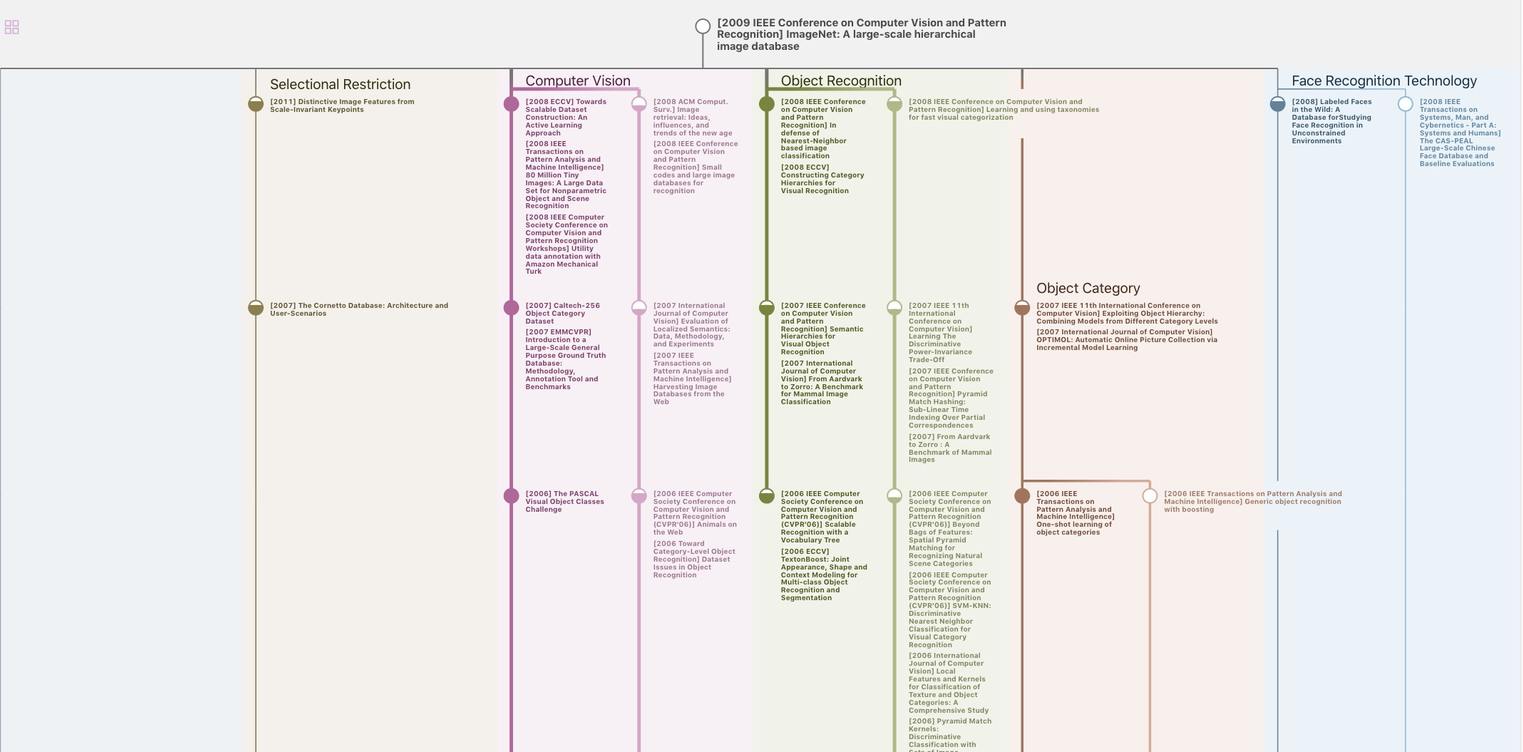
生成溯源树,研究论文发展脉络
Chat Paper
正在生成论文摘要