Sparse signal recovery under Poisson statistics.
2013 51ST ANNUAL ALLERTON CONFERENCE ON COMMUNICATION, CONTROL, AND COMPUTING (ALLERTON)(2013)
摘要
We are motivated by problems that arise in a number of applications such as explosives detection and online Marketing, where the observations are governed by Poisson statistics. Here each observation is a Poisson random variable whose mean is a sparse linear superposition of known patterns. Unlike many conventional problems observations here are not identically distributed since they are associated with different sensing modalities. We analyse the performance of a Maximum Likelihood (ML) decoder, which for our Poisson setting is computationally tractable. We derive fundamental sample complexity bounds for sparse recovery in the high-dimensional setting. We show that when the sensing matrix satisfies the socalled Restricted Eigenvalue (RE) condition the l(1) regularized ML decoder is consistent. Moreover, it converges exponentially fast in terms of number of observations. Our results apply to both deterministic and random sensing matrices and we present several results for both cases.
更多查看译文
关键词
Poisson Model Selection,Sparse Recovery,Regularized Maximum Likelihood
AI 理解论文
溯源树
样例
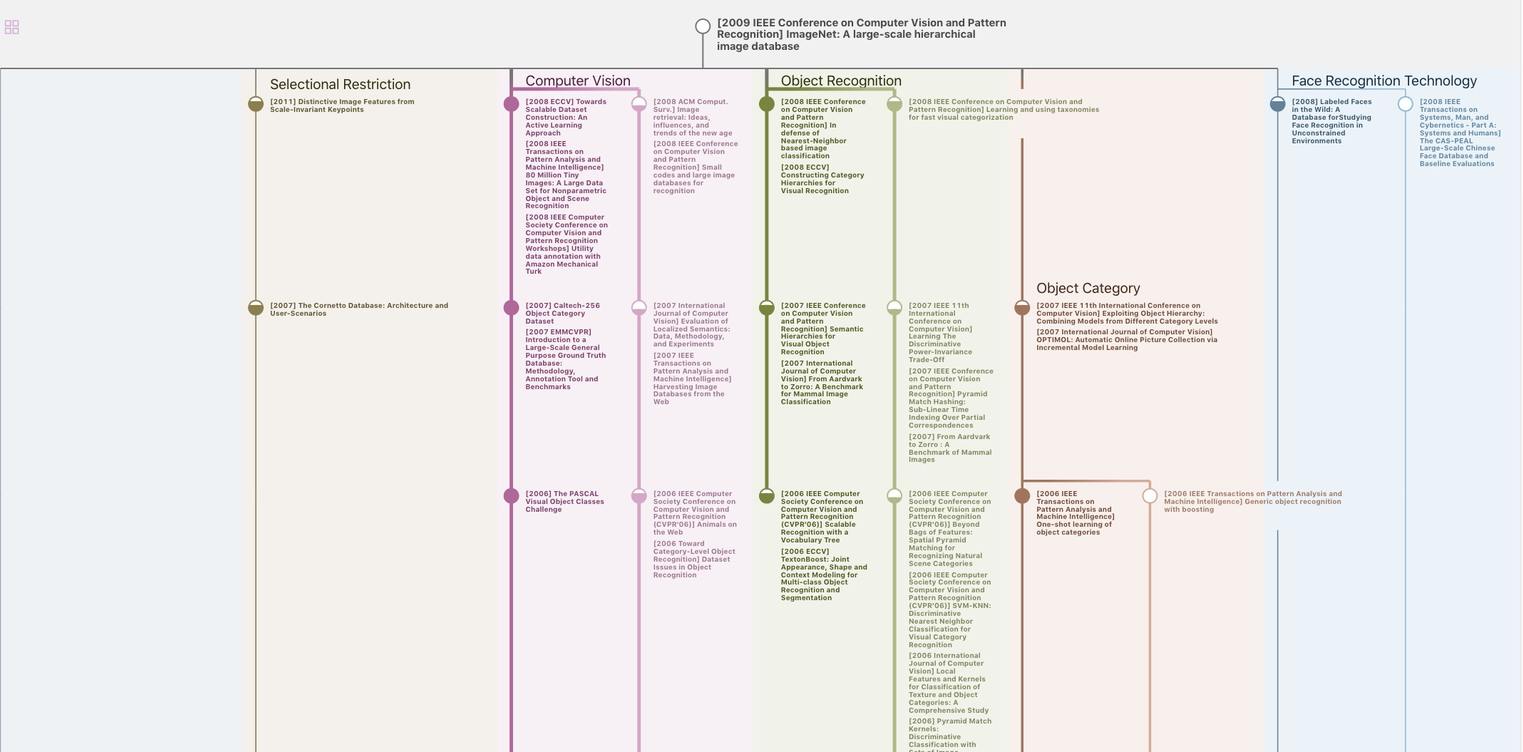
生成溯源树,研究论文发展脉络
Chat Paper
正在生成论文摘要