An improvement of symbolic aggregate approximation distance measure for time series.
Neurocomputing(2014)
摘要
Symbolic Aggregate approXimation (SAX) as a major symbolic representation has been widely used in many time series data mining applications. However, because a symbol is mapped from the average value of a segment, the SAX ignores important information in a segment, namely the trend of the value change in the segment. Such a miss may cause a wrong classification in some cases, since the SAX representation cannot distinguish different time series with similar average values but different trends. In this paper, we firstly design a measure to compute the distance of trends using the starting and the ending points of segments. Then we propose a modified distance measure by integrating the SAX distance with a weighted trend distance. We show that our distance measure has a tighter lower bound to the Euclidean distance than that of the original SAX. The experimental results on diverse time series data sets demonstrate that our proposed representation significantly outperforms the original SAX representation and an improved SAX representation for classification.
更多查看译文
关键词
Time series,Trend distance,Symbolic Aggregate approXimation,Lower bound,Classification
AI 理解论文
溯源树
样例
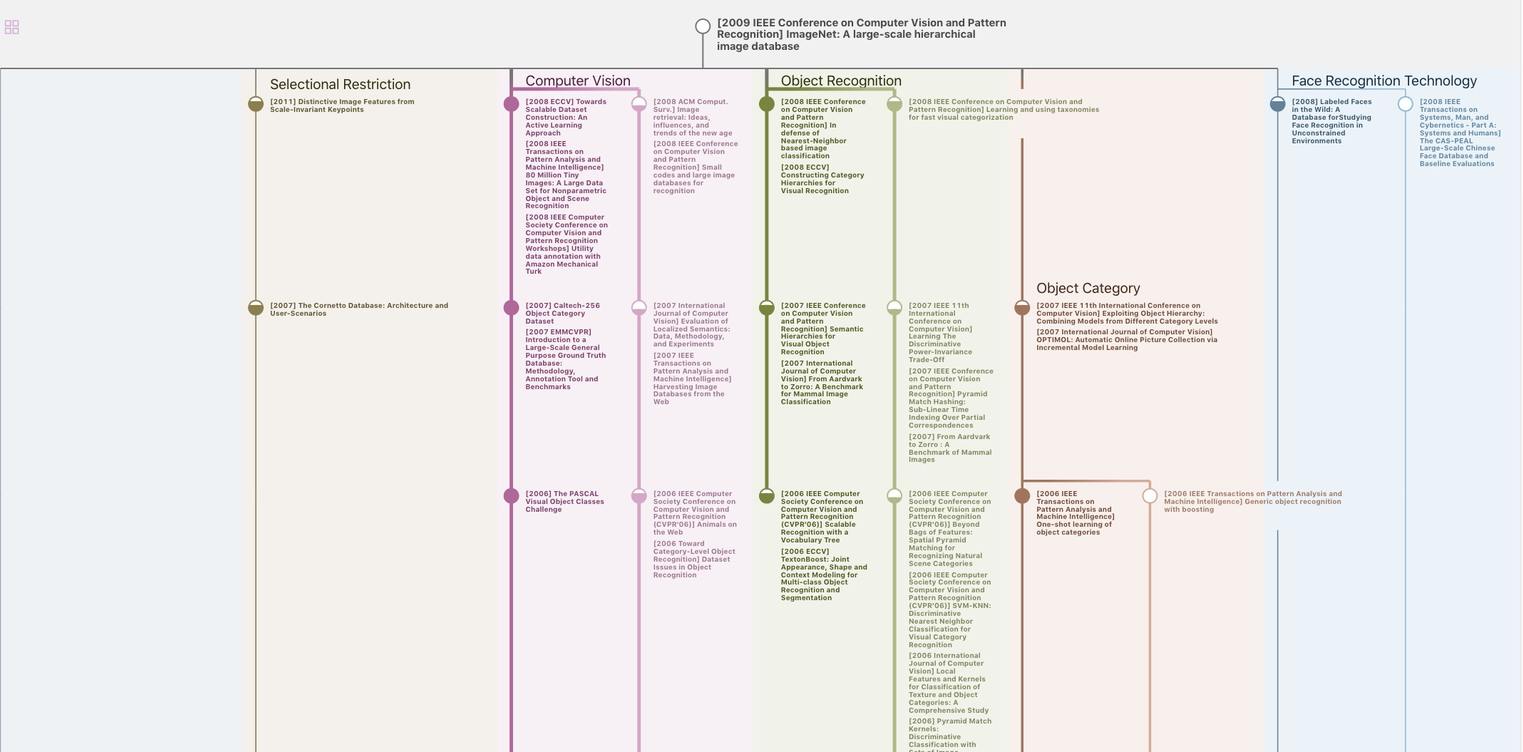
生成溯源树,研究论文发展脉络
Chat Paper
正在生成论文摘要