Seasonal adjustment with 'bad' neural networks: a case of benign underfitting
International Journal of Applied Management Science(2010)
摘要
This paper is a response to reports by a number of authors of difficulties in using feedforward neural networks (NNs) in the presence of seasonality. Such difficulties appear to contradict the well-known property of 'universal approximation', but arise in fact because the property only applies to suitably smooth functions. We report on what is an unexpected benefit of this limitation of NNs: although the network is unable to follow seasonal movements it can identify an underlying trend, through being forced to steer a middle course. We achieve such deseasonalisation through network pruning, which restricts the approximation capacity of the network by depriving it of connections. Although pruning is in general motivated by the search for the most parsimonious network, in our particular context it forces the network to ignore both seasonal movements and noise.
更多查看译文
关键词
neural network,seasonality,differential evolution,seasonal adjustment,feedforward neural network,penalty function,neural networks
AI 理解论文
溯源树
样例
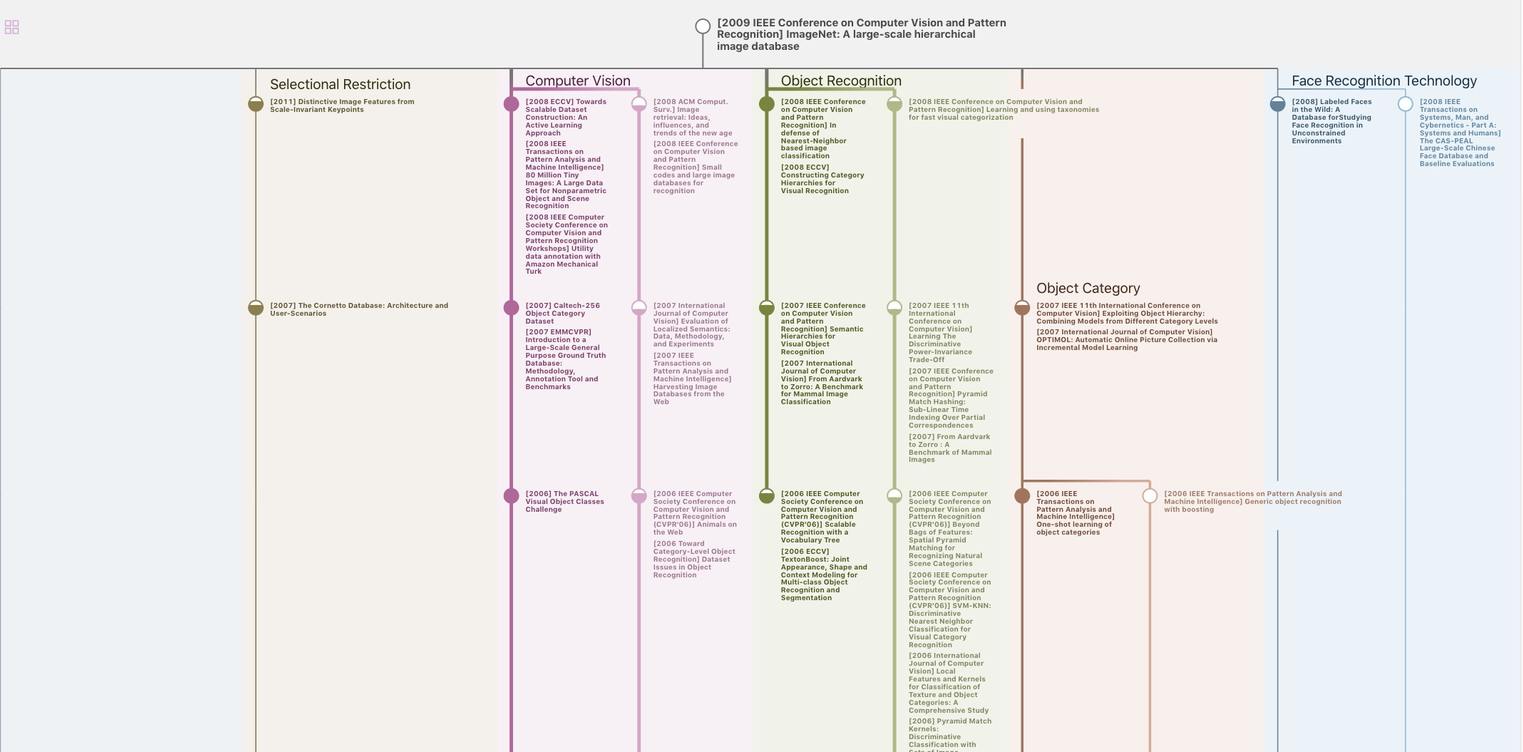
生成溯源树,研究论文发展脉络
Chat Paper
正在生成论文摘要