Generating gaits for physical quadruped robots: evolved neural networks vs. local parameterized search.
GECCO '11: Genetic and Evolutionary Computation Conference Dublin Ireland July, 2011(2011)
摘要
Creating gaits for legged robots is an important task to enable robots to access rugged terrain, yet designing such gaits by hand is a challenging and time-consuming process. In this paper we investigate various algorithms for automating the creation of quadruped gaits. Because many robots do not have accurate simulators, we test gait learning algorithms entirely on a physical robot. We compare the performance of two classes of learning gaits: locally searching parameterized motion models and evolving artificial neural networks with the HyperNEAT generative encoding. All parameter search methods outperform a manually-designed reference gait, but HyperNEAT performs better still, producing gaits nearly 9 times faster than the reference gait.
更多查看译文
AI 理解论文
溯源树
样例
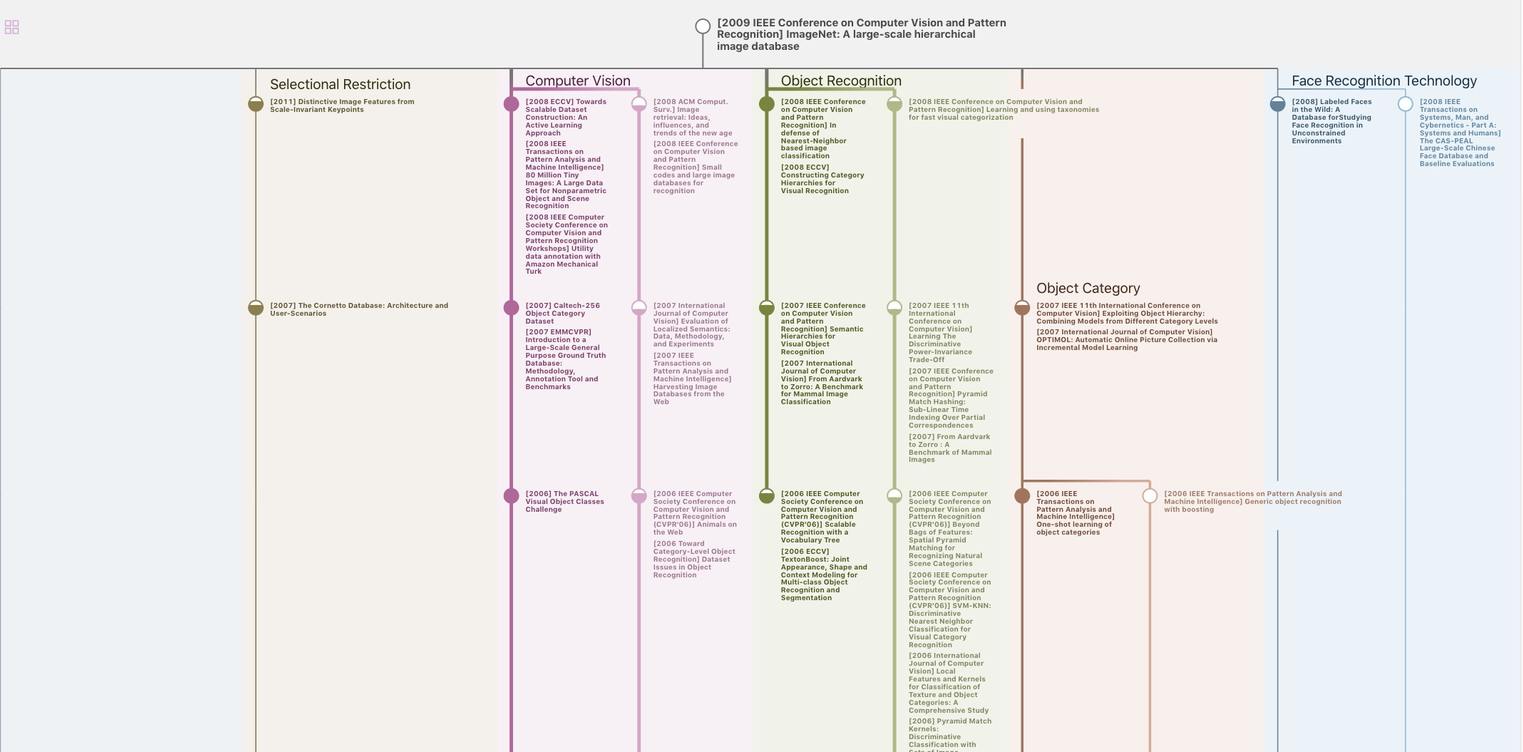
生成溯源树,研究论文发展脉络
Chat Paper
正在生成论文摘要