Data Visualisation And Identification Of Anomalies In Power System State Estimation Using Artificial Neural Networks
IEE PROCEEDINGS-GENERATION TRANSMISSION AND DISTRIBUTION(1997)
摘要
Bad data identification is one of the most important and complex problems to be addressed during power system state estimation, particularly when both analogical and topological errors (branch or bus misconfigurations) are to be considered. The paper proposes a new method that is capable of distinguishing between analogical and topological errors, and also of identifying which are the bad measurements or the misconfigurated elements due to unreported or incorrectly reported line outages, bus splits etc. The method explores the discrimination capability of the normalised innovations (the differences between the latest acquired measurements and their corresponding predicted quantities), which are used as input variables to an artificial neural network that provides, in the output, the anomaly identification. Data projection techniques are also used to visualise and confirm the discrimination capability of the normalised innovations. The method is tested using the IEEE 24-bus test system, where several types of errors have been simulated, including single and multiple bad measurements, topology errors involving branches or buses etc.
更多查看译文
关键词
power system state estimation, power system monitoring, forecasting-aided state estimation, bad data, feature selection
AI 理解论文
溯源树
样例
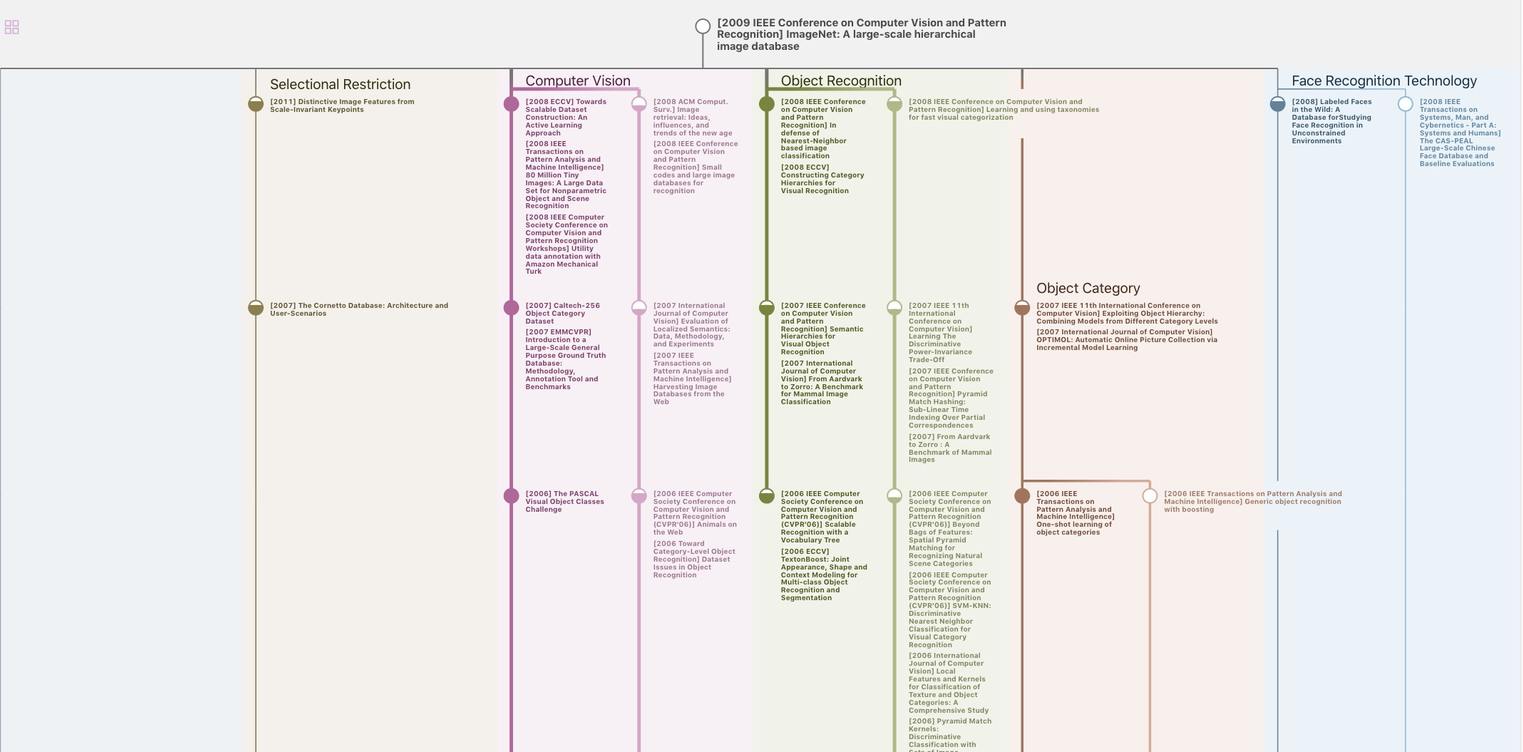
生成溯源树,研究论文发展脉络
Chat Paper
正在生成论文摘要