LD-BSCA: A local-density based spatial clustering algorithm
CIDM(2009)
摘要
Density-based clustering algorithms are very powerful to discover arbitrary-shaped clusters in large spatial databases. However, in many cases, varied local-density clusters exist in different regions of data space. In this paper, a new algorithm LD-BSCA is proposed with introducing the concept of local MinPts (a minimum number of points) and the new cluster expanding condition: ExpandConClId (Expanding Condition of ClId-th Cluster). We minimize the algorithm input down to only one parameter and let the local MinPts diversified as clusters change from one to another simultaneously. Experiments show LD-BSCA algorithm is powerful to discover all clusters in gradient distributing databases. In addition, we introduce an efficient searching method to reduce the runtime of our algorithm. Using several databases, we demonstrate the high quality of the proposed algorithm in clustering the implicit knowledge in asymmetric distribution databases.
更多查看译文
关键词
pattern clustering,visual databases,local minpts,search problems,expandconclid,local-density-based spatial clustering algorithm,large spatial database,ld-bsca,very large databases,search method,distributed database,algorithm design and analysis,clustering algorithms,databases,noise,data mining,spatial database,optics
AI 理解论文
溯源树
样例
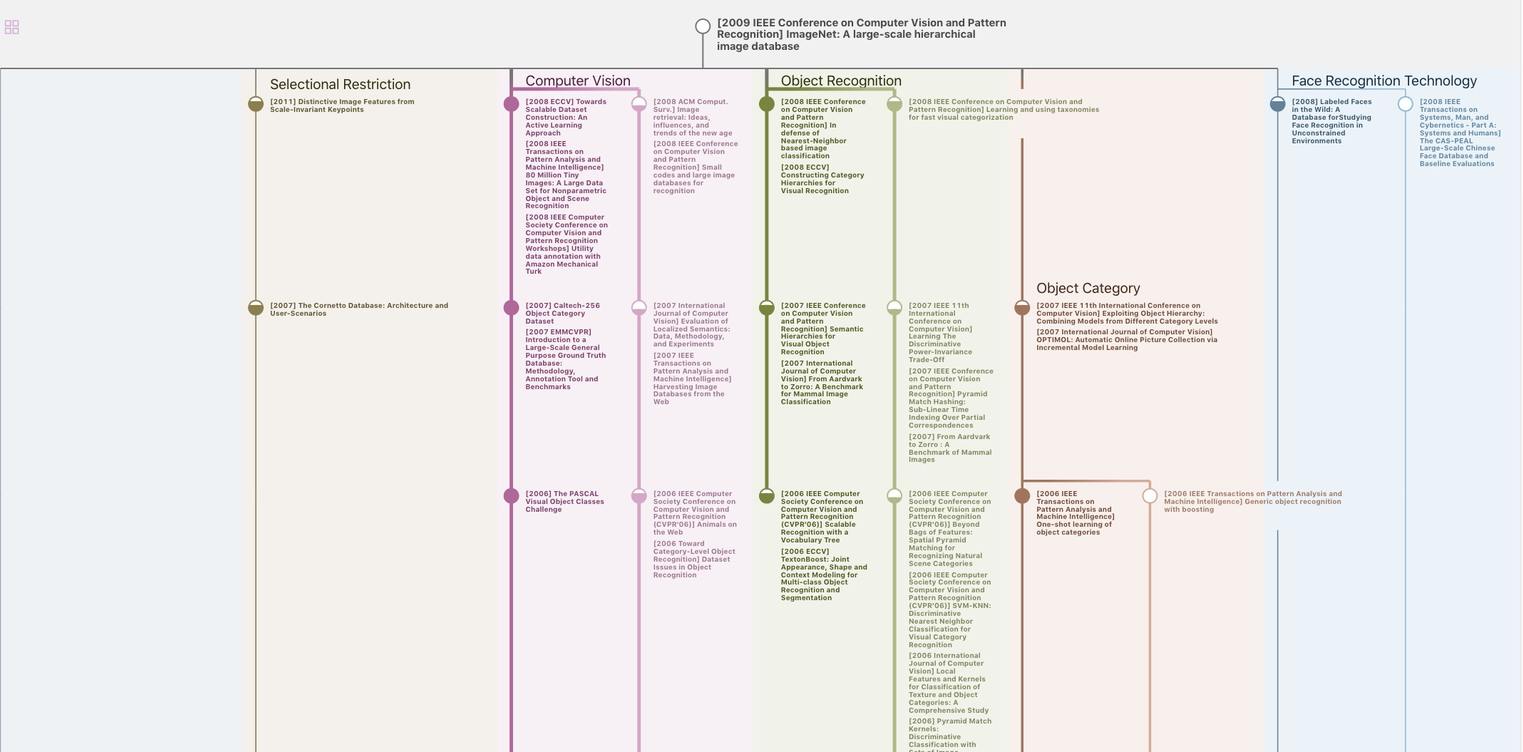
生成溯源树,研究论文发展脉络
Chat Paper
正在生成论文摘要