Q-Learning in Continuous State and Action Spaces
Australian Joint Conference on Artificial Intelligence(1999)
摘要
Q-learning can be used to learn a control policy that maximises a scalar reward through interaction with the environment. Q- learning is commonly applied to problems with discrete states and actions. We describe a method suitable for control tasks which require continuous actions, in response to continuous states. The system consists of a neureil network coupled with a novel interpolator. Simulation results are presented for a non-holonomic control task. Advantage Learning, a variation of Q-learning, is shown enhance learning speed and reliability for this task.
更多查看译文
关键词
novel interpolator,scalar reward,action spaces,continuous state,continuous action,control task,non-holonomic control task,control policy,neureil network,advantage learning,discrete state,neural network
AI 理解论文
溯源树
样例
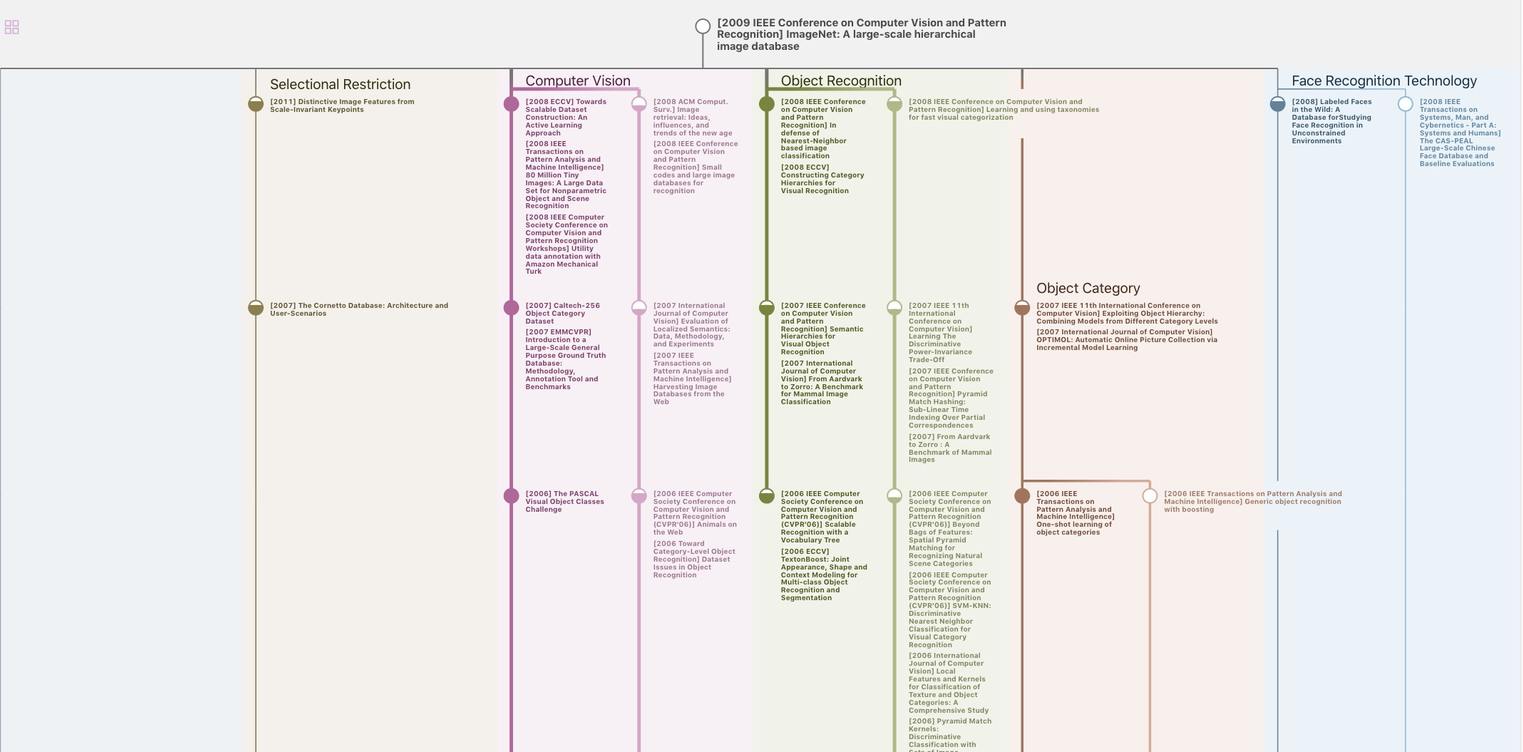
生成溯源树,研究论文发展脉络
Chat Paper
正在生成论文摘要