Virtual Sample Generation For Template-Based Shape Matching
2001 IEEE COMPUTER SOCIETY CONFERENCE ON COMPUTER VISION AND PATTERN RECOGNITION, VOL 1, PROCEEDINGS(2001)
摘要
This paper presents a method for improving the performance of matching systems that correlate using shape templates. The basic idea involves extending an existing set of training shapes with generated "virtual" shapes, in order to improve representational capability. Yet no a-priori feature correspondence is necessary among the original shapes in the training set. Instead, an integrated clustering and registration approach partitions the original shape samples into cluster's of similar and registered shapes; in each cluster a separate feature space is embedded. This allows for each cluster the derivation of standard compact parameterizations. This paper demonstrates that sampling these low-order spaces can produce an extended training set which facilitates a superior matching performance, as measured by a ROC curve. In the experiments, we consider a realistic application involving thousands of pedestrian shapes and perform correlation matching based on distance transforms.
更多查看译文
关键词
lighting,distance transform,roc curve,feature space,robustness,representation,sampling methods,gaussian distribution
AI 理解论文
溯源树
样例
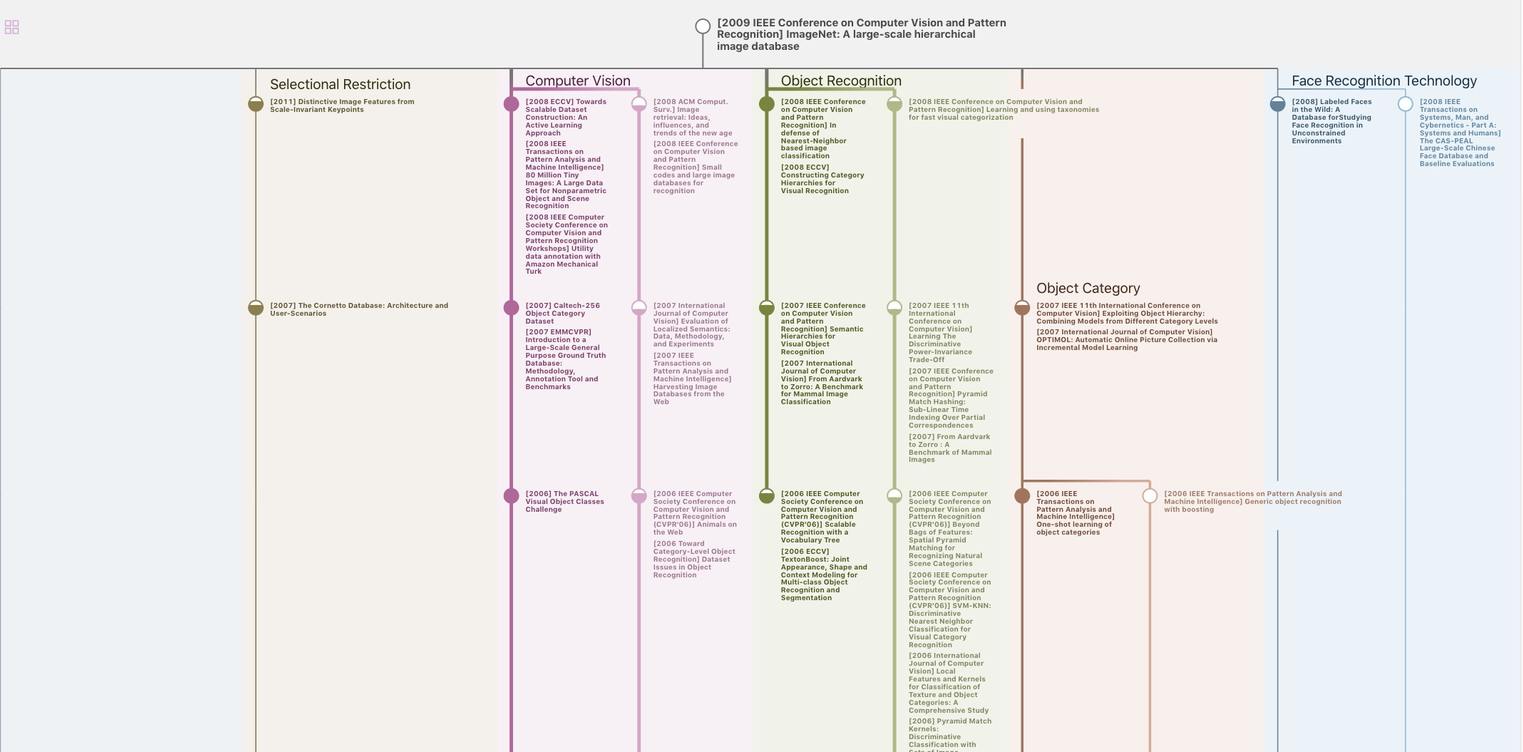
生成溯源树,研究论文发展脉络
Chat Paper
正在生成论文摘要