Unsupervised Text Segmentation using LDA and MCMC.
AusDM '12: Proceedings of the Tenth Australasian Data Mining Conference - Volume 134(2012)
摘要
In this paper, we propose a data driven approach to text segmentation, while most of the existing unsupervised methods determine segmentation boundaries by empirically exploring similarity measurement between adjacent units (e.g. sentences). Firstly, we train a latent Dirichlet allocation (LDA) model with the large scale Wikipedia Corpus to avoid the problem of vocabulary mismatch, which makes our approach domain-independent. Secondly, each segment unit is represented with a distribution of the topics, instead of a set of word tokens. Finally, a text input is modeled as a sequence of segment units and Markov Chain Monte Carlo technique is employed to decide the appropriate boundaries. The major advantage of using MCMC is its ability to detect both strong and weak boundaries. Experimental results demonstrate that our proposed approach achieve promising results on a widely used benchmark dataset when compared with the state-of-the-art methods.
更多查看译文
关键词
segment unit,approach domain-independent,proposed approach,segmentation boundary,text input,text segmentation,Markov Chain Monte Carlo,adjacent unit,appropriate boundary,benchmark dataset,Unsupervised text segmentation
AI 理解论文
溯源树
样例
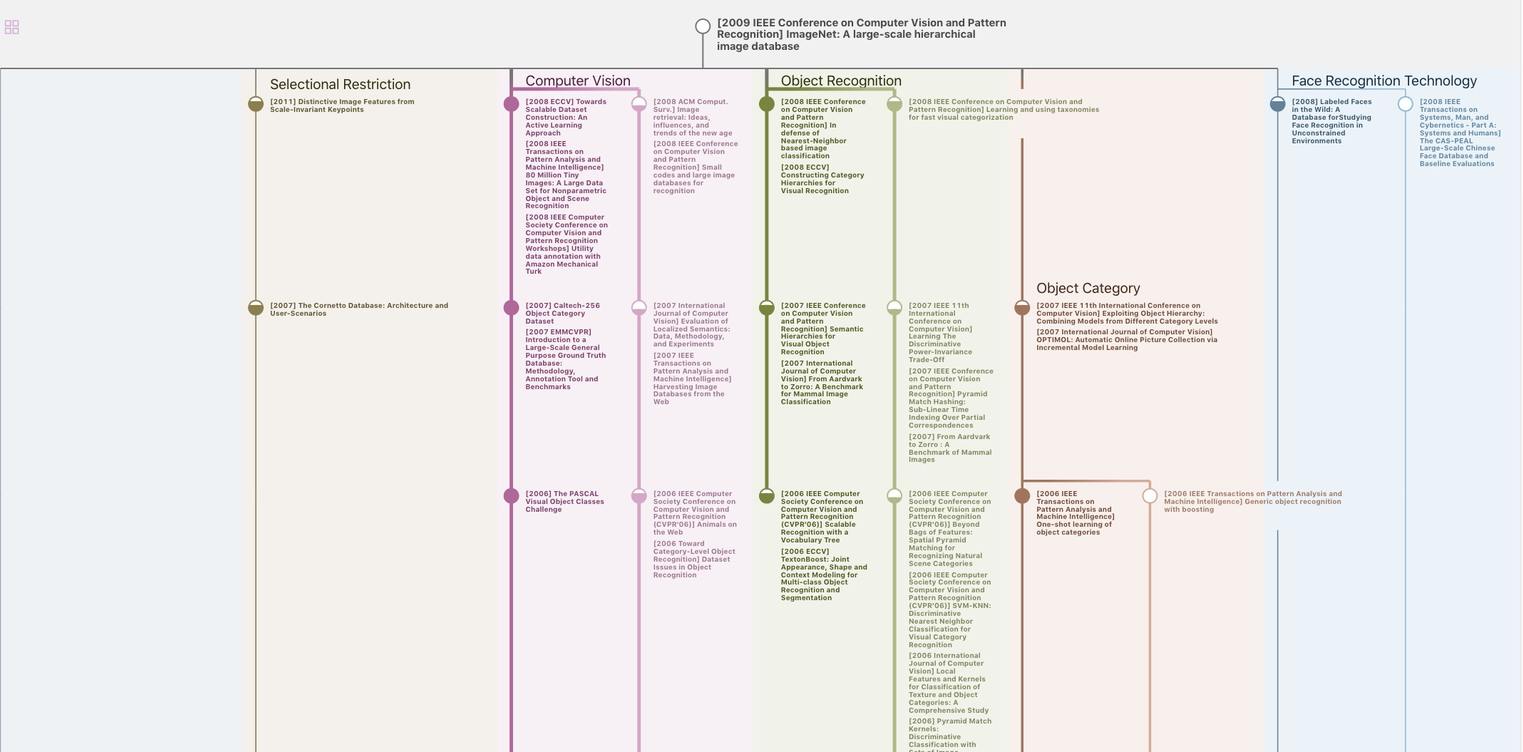
生成溯源树,研究论文发展脉络
Chat Paper
正在生成论文摘要