On The Equivalent Of Low-Rank Regressions And Linear Discriminant Analysis Based Regressions
KDD(2013)
摘要
The low-rank regression model has been studied and applied to capture the underlying classes/tasks correlation patterns, such that the regression/classification results can be enhanced. In this paper, we will prove that the low-rank regression model is equivalent to doing linear regression in the linear discriminant analysis (LDA) subspace. Our new theory reveals the learning mechanism of low-rank regression, and shows that the low-rank structures exacted from classes/tasks are connected to the LDA projection results. Thus, the low-rank regression efficiently works for the high-dimensional data.Moreover, we will propose new discriminant low-rank ridge regression and sparse low-rank regression methods. Both of them are equivalent to doing regularized regression in the regularized LDA subspace. These new regularized objectives provide better data mining results than existing low-rank regression in both theoretical and empirical validations. We evaluate our discriminant low-rank regression methods by six benchmark datasets. In all empirical results, our discriminant low-rank models consistently show better results than the corresponding full-rank methods.
更多查看译文
关键词
Low-Rank Regression, Low-Rank Ridge Regression, Sparse Low-Rank Regression, Linear Discriminant Analysis
AI 理解论文
溯源树
样例
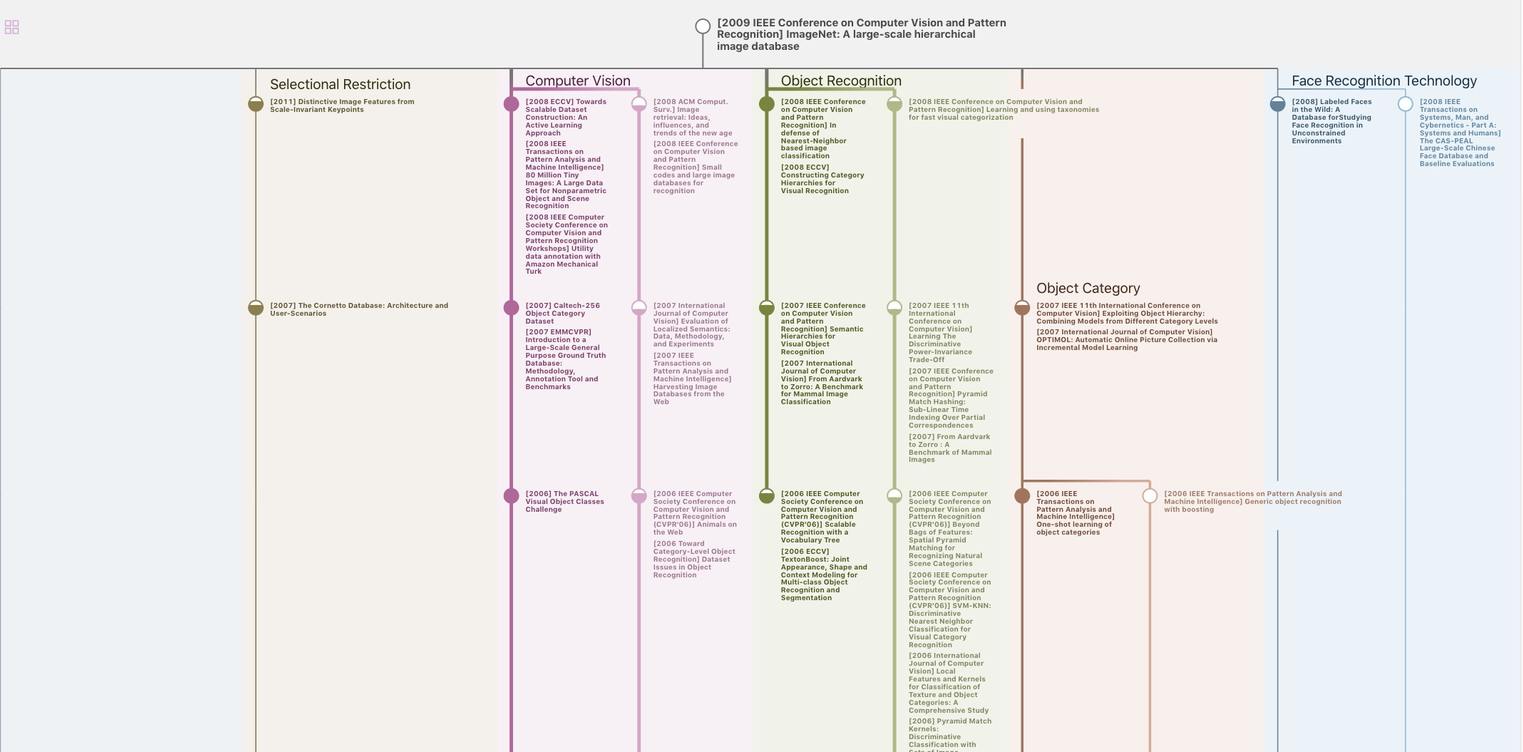
生成溯源树,研究论文发展脉络
Chat Paper
正在生成论文摘要