Weighted '1 Minimization for Event Detection in Sensor Networks
msra(2009)
摘要
Event detection is an important application of wire- less sensor networks. When the event signature is sparse in a known domain, mechanisms from the emerging area of Com- pressed Sensing (CS) can be applied for estimation with average measurement rates far lower than the Nyquist requirement. A re- cently proposed algorithm called IDEA uses knowledge of where the signal is sparse combined with a greedy search procedure called Orthogonal Matching Pursuit (OMP) to demonstrate that detection can be performed in the sparse domain with even fewer measurements. A different approach called Basis Pursuit (BP), which uses '1 norm minimization, provides better performance in reconstruction but suffers from a larger sampling cost since it tries to estimate the signal completely. In this paper, we introduce a mechanism that uses a modified BP approach for detection of sparse signals with known signature. The modification is inspired from a novel development that uses an adaptively weighted version of BP. We show, through simulation and experiments on MicaZ motes, that by appropri- ately weighting the coefficients during '1 norm minimization, detection performance exceeds that of an unweighted approach at comparable sampling rates.
更多查看译文
AI 理解论文
溯源树
样例
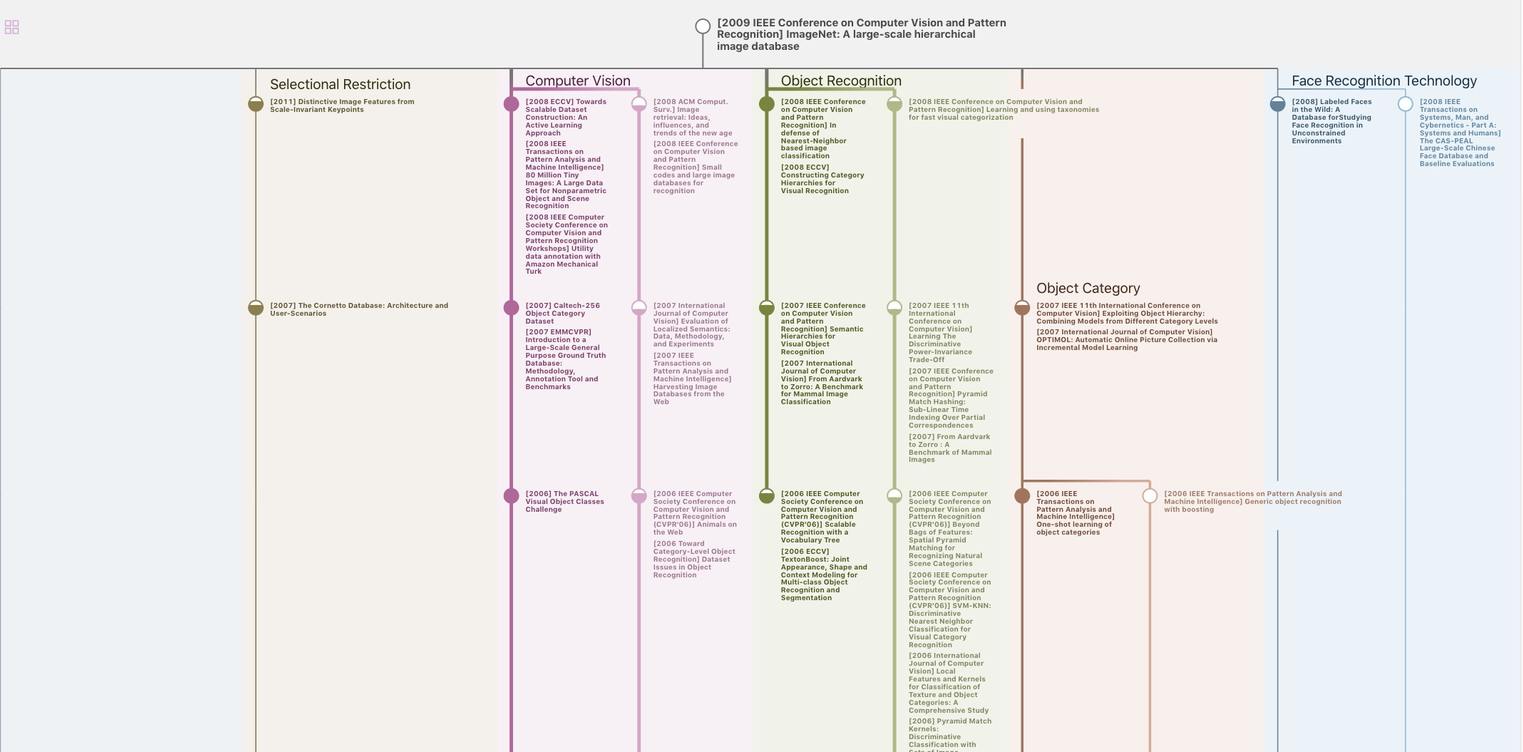
生成溯源树,研究论文发展脉络
Chat Paper
正在生成论文摘要