Domain-Constrained Semi-Supervised Mining Of Tracking Models In Sensor Networks
Proceedings of the 13th ACM SIGKDD international conference on Knowledge discovery and data mining(2007)
摘要
Accurate localization of mobile objects is a major research problem in sensor networks and an important data mining application. Specifically, the localization problem is to determine the location of a client device accurately given the radio signal strength values received at the client device from multiple beacon sensors or access points. Conventional data mining and machine learning methods can be applied to solve this problem. However, all of them require large amounts of labeled training data, which can be quite expensive. In this paper. we propose a probabilistic semi-supervised learning approach to reduce the calibration effort and increase the tracking accuracy. Our method is based on semi-supervised conditional random fields which can enhance the learned model from a small set of training data with abundant unlabeled data effectively. To make our method more efficient, we exploit a Generalized EM algorithm coupled with domain constraints. We validate our method through extensive experiments in a real sensor network using Crossbow MICA2 sensors. The results demonstrate the advantages of methods compared to other state-of-the-art object-tracking algorithms.
更多查看译文
关键词
Localization,Calibration,Tracking,Sensor Networks,EM,CRF
AI 理解论文
溯源树
样例
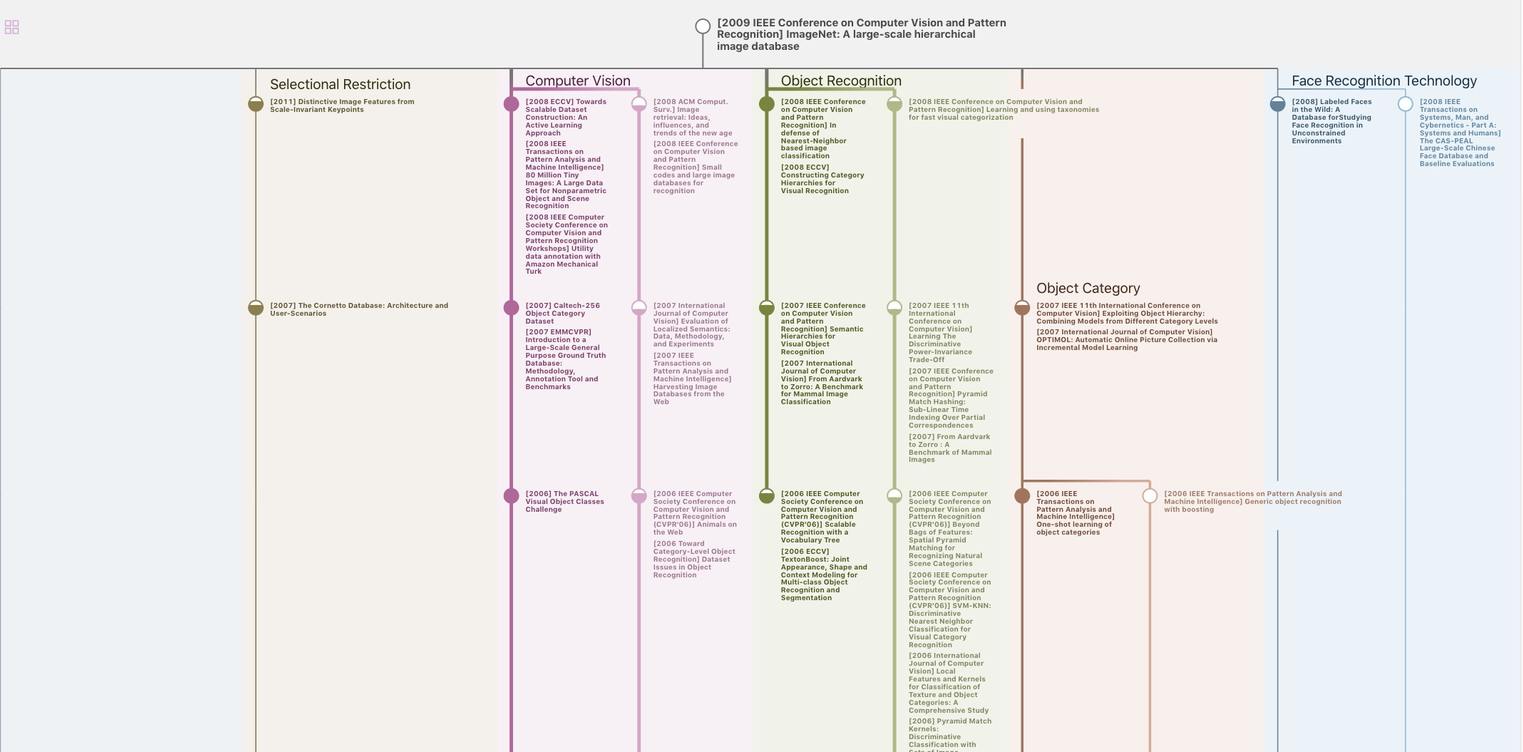
生成溯源树,研究论文发展脉络
Chat Paper
正在生成论文摘要