Using stochastic control with data compression perspectives to enhance P300 Neural Communication Prostheses
Porto(2008)
摘要
We design a study assess to improve the speed in a P300 Brain-Computer Interface, originally described by Farewell and Donchin in 1988. A grid of characters is displayed on a screen randomly, and when the character of interest is illuminated, 300 ms afterwards a deflection in the EEG signal is generated. In such scenarios, the time to specify a menu option is proportional to its size. Most researchers in the past have considered fixed menu size and focused on more advanced statistical detection/classification techniques. We propose to use statistical language structure to introduce a new degree of freedom in this paradigm. Finding the optimal policy to display menus, given previously conveyed characters, can be cast in terms of ldquodynamic programmingrdquo or ldquostochastic controlrdquo. We illustrate how the optimal policy of this dynamic programming problem satisfies a menu depth to character probability relation analogous to that of the Huffman code in data compression and information theory. By exploiting this optimality property, we illustrate how the state space of the stochastic control problem can be greatly diminished (from exponential growth to linear growth), and we exhibit optimal policies for a probabilistic model pertaining to the statistical structure of the English language.
更多查看译文
关键词
eeg signal,advanced statistical detection,electrocardiography,p300 neural communication prostheses,english language,stochastic systems,statistical analysis,pattern classification,data compression,prosthetics,i. introduction,information theory,optimal policy,advanced statistical classification,probability relation,stochastic control,optimal policies,dynamic programming,statistical language structure,p300 brain-computer interface,huffman code,probabilistic model,state space,satisfiability,degree of freedom,huffman codes,brain computer interface,exponential growth
AI 理解论文
溯源树
样例
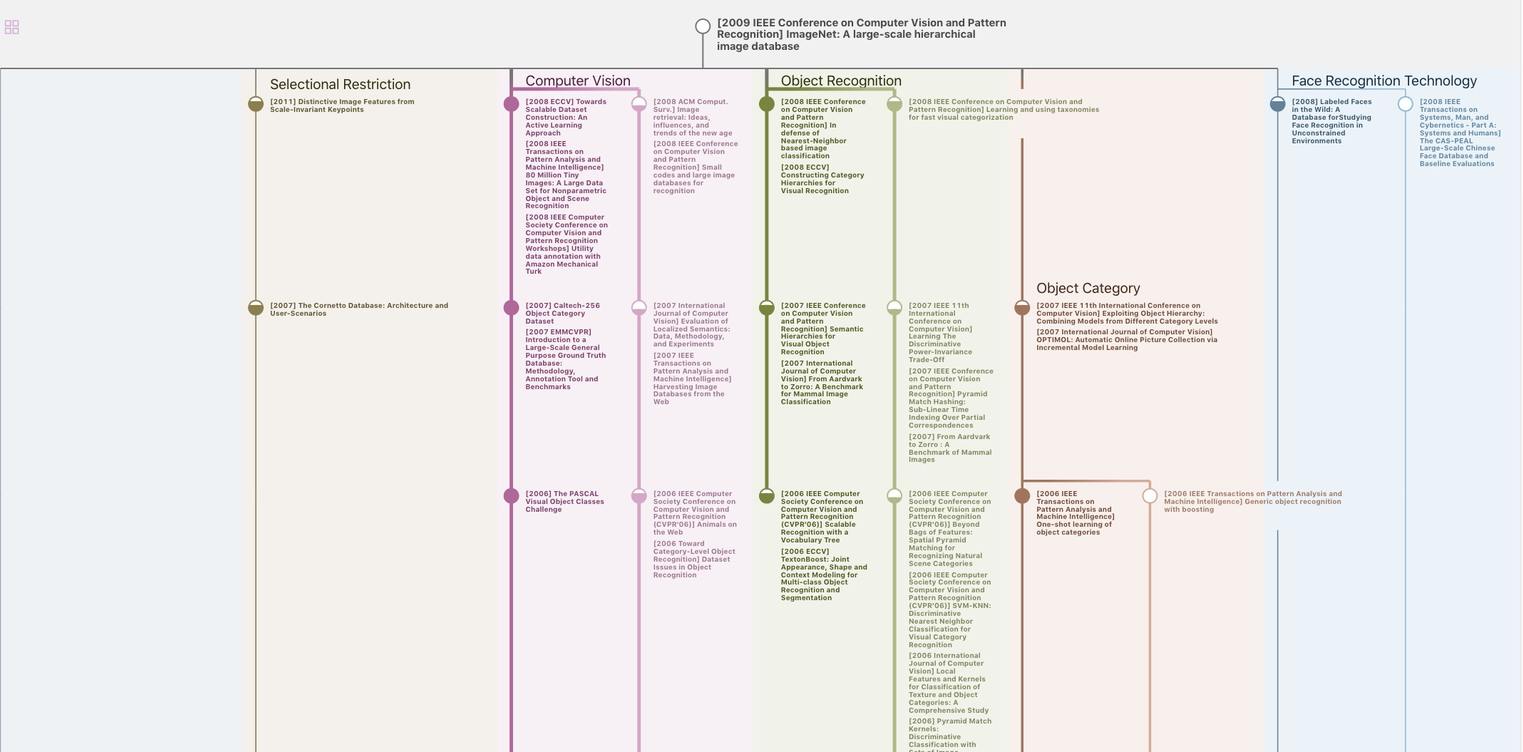
生成溯源树,研究论文发展脉络
Chat Paper
正在生成论文摘要